Variational multinomial logit gaussian process
Journal of Machine Learning Research(2012)
摘要
Gaussian process prior with an appropriate likelihood function is a flexible non-parametric model for a variety of learning tasks. One important and standard task is multi-class classification, which is the categorization of an item into one of several fixed classes. A usual likelihood function for this is the multinomial logistic likelihood function. However, exact inference with this model has proved to be difficult because high-dimensional integrations are required. In this paper, we propose a variational approximation to this model, and we describe the optimization of the variational parameters. Experiments have shown our approximation to be tight. In addition, we provide data-independent bounds on the marginal likelihood of the model, one of which is shown to be much tighter than the existing variational mean-field bound in the experiments. We also derive a proper lower bound on the predictive likelihood that involves the Kullback-Leibler divergence between the approximating and the true posterior. We combine our approach with a recently proposed sparse approximation to give a variational sparse approximation to the Gaussian process multi-class model. We also derive criteria which can be used to select the inducing set, and we show the effectiveness of these criteria over random selection in an experiment.
更多查看译文
关键词
marginal likelihood,appropriate likelihood function,predictive likelihood,existing variational mean-field,gaussian process multi-class model,sparse approximation,multinomial logistic likelihood function,usual likelihood function,variational approximation,flexible non-parametric model,probabilistic classification,gaussian process
AI 理解论文
溯源树
样例
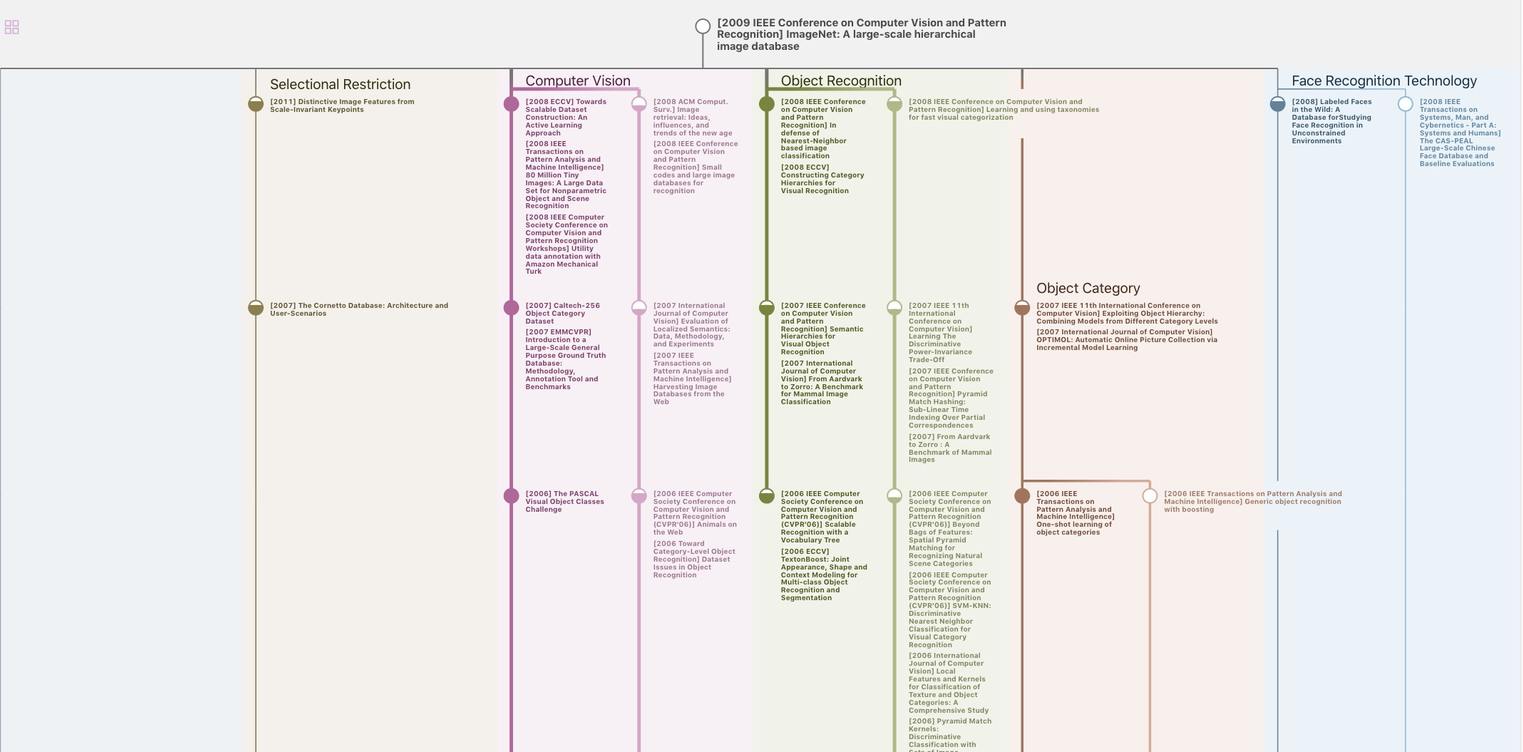
生成溯源树,研究论文发展脉络
Chat Paper
正在生成论文摘要