Autonomous Vehicle Cut-In Algorithm for Lane-Merging Scenarios via Policy-Based Reinforcement Learning Nested Within Finite-State Machine
IEEE Transactions on Intelligent Transportation Systems(2022)
摘要
Lane-merging scenarios pose highly challenging problems for autonomous vehicles due to conflicts of interest between the human-driven and cutting-in autonomous vehicles. Such conflicts become severe when traffic increases, and cut-in algorithms suffer from a steep trade-off between safety and cut-in performance. In this study, a reinforcement learning (RL)-based cut-in policy network nested within a finite state machine (FSM)—which is a high-level decision maker, is proposed to achieve high cut-in performance without sacrificing safety. This FSM-RL hybrid approach is proposed to obtain 1) a strategic and adjustable algorithm, 2) optimal safety and cut-in performance, and 3) robust and consistent performance. In the high-level decision making algorithm, the FSM provides a framework for four cut-in phases (ready for safe gap selection, gap approach, negotiation, and lane-change execution) and handles the transitions between these phases by calculating the collision risks associated with target vehicles. For the lane-change phase, a policy-based deep-RL approach with a soft actor–critic network is employed to get optimal cut-in performance. The results of simulations show that the proposed FSM-RL cut-in algorithm consistently achieves a high cut-in success rate without sacrificing safety. In particular, as the traffic increases, the cut-in success rate and safety are significantly improved over existing optimized rule-based cut-in algorithms and end-to-end RL algorithm.
更多查看译文
关键词
Autonomous vehicle,lane-merge,cut-in,deep reinforcement learning,finite-state machine
AI 理解论文
溯源树
样例
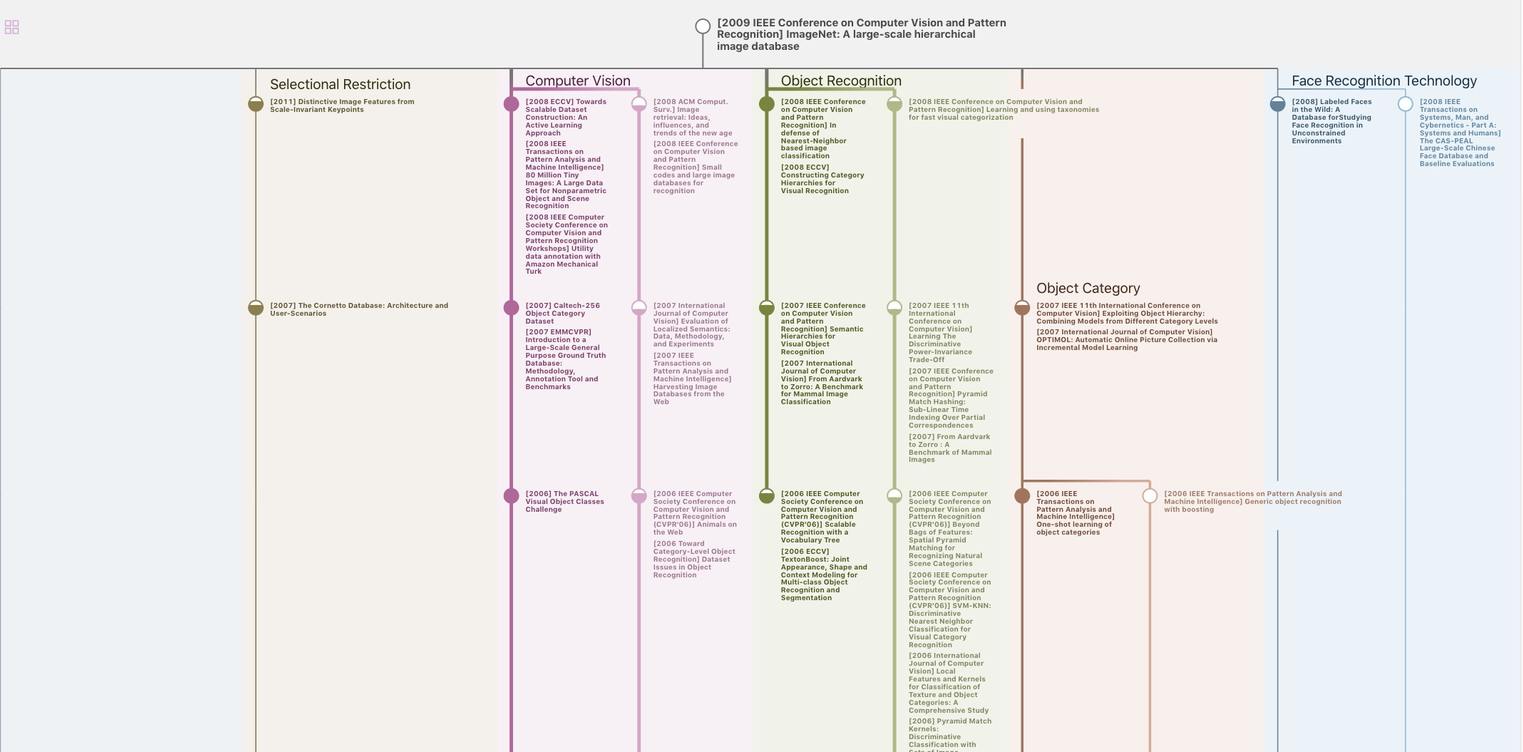
生成溯源树,研究论文发展脉络
Chat Paper
正在生成论文摘要