Stereoscopic learning for disparity estimation
ISCAS(2011)
摘要
In this paper, we propose a learning based approach to estimating pixel disparities from the motion information extracted out of input monoscopic video sequences. We represent each video frame with superpixels, and extract the motion features from the superpixels and the frame boundary. These motion features account for the motion pattern of the superpixel as well as camera motion. In the learning phase, given a pair of stereoscopic video sequences, we employ a state-of-the-art stereo matching method to compute the disparity map of each frame as ground truth. Then a multi-label SVM is trained from the estimated disparities and the corresponding motion features. In the testing phase, we use the learned SVM to predict the disparity for each superpixel in a monoscopic video sequence. Experiment results show that the proposed method achieves low error rate in disparity estimation.
更多查看译文
关键词
frame boundary,superpixel,image matching,motion information,input monoscopic video sequences,stereoscopic video sequences,testing phase,estimation theory,multilabel svm,camera motion,motion pattern,image sequences,disparity map,stereo matching method,stereo image processing,pixel disparities,stereoscopic learning,learning phase,motion features,support vector machines,video frame,disparity estimation,three dimensional,information extraction,pixel,histograms,feature extraction,ground truth,support vector machine,error rate,optical imaging
AI 理解论文
溯源树
样例
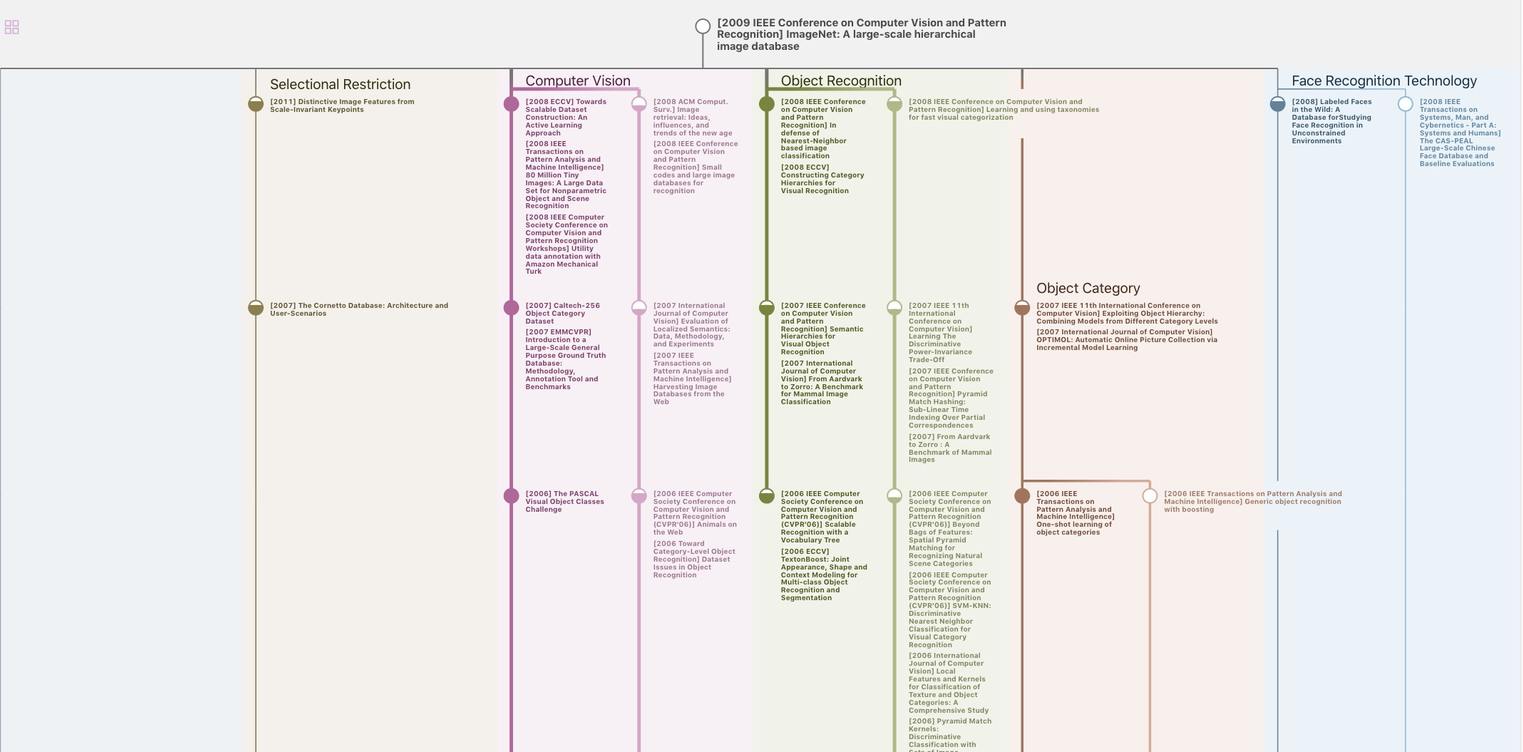
生成溯源树,研究论文发展脉络
Chat Paper
正在生成论文摘要