Space-time dynamics of topics in streaming text.
GIS(2011)
摘要
ABSTRACTHuman-generated textual data streams from services such as Twitter increasingly become geo-referenced. The spatial resolution of their coverage improves quickly, making them a promising instrument for sensing various aspects of evolution and dynamics of social systems. This work explores spacetime structures of the topical content of short textual messages in a stream available from Twitter in Ireland. It uses a streaming Latent Dirichlet Allocation topic model trained with an incremental variational Bayes method. The posterior probabilities of the discovered topics are post-processed with a spatial kernel density and subjected to comparative analysis. The identified prevailing topics are often found to be spatially contiguous. We apply Markov-modulated non-homogeneous Poisson processes to quantify a proportion of novelty in the observed abnormal patterns. A combined use of these techniques allows for real-time analysis of the temporal evolution and spatial variability of population's response to various stimuli such as large scale sportive, political or cultural events.
更多查看译文
AI 理解论文
溯源树
样例
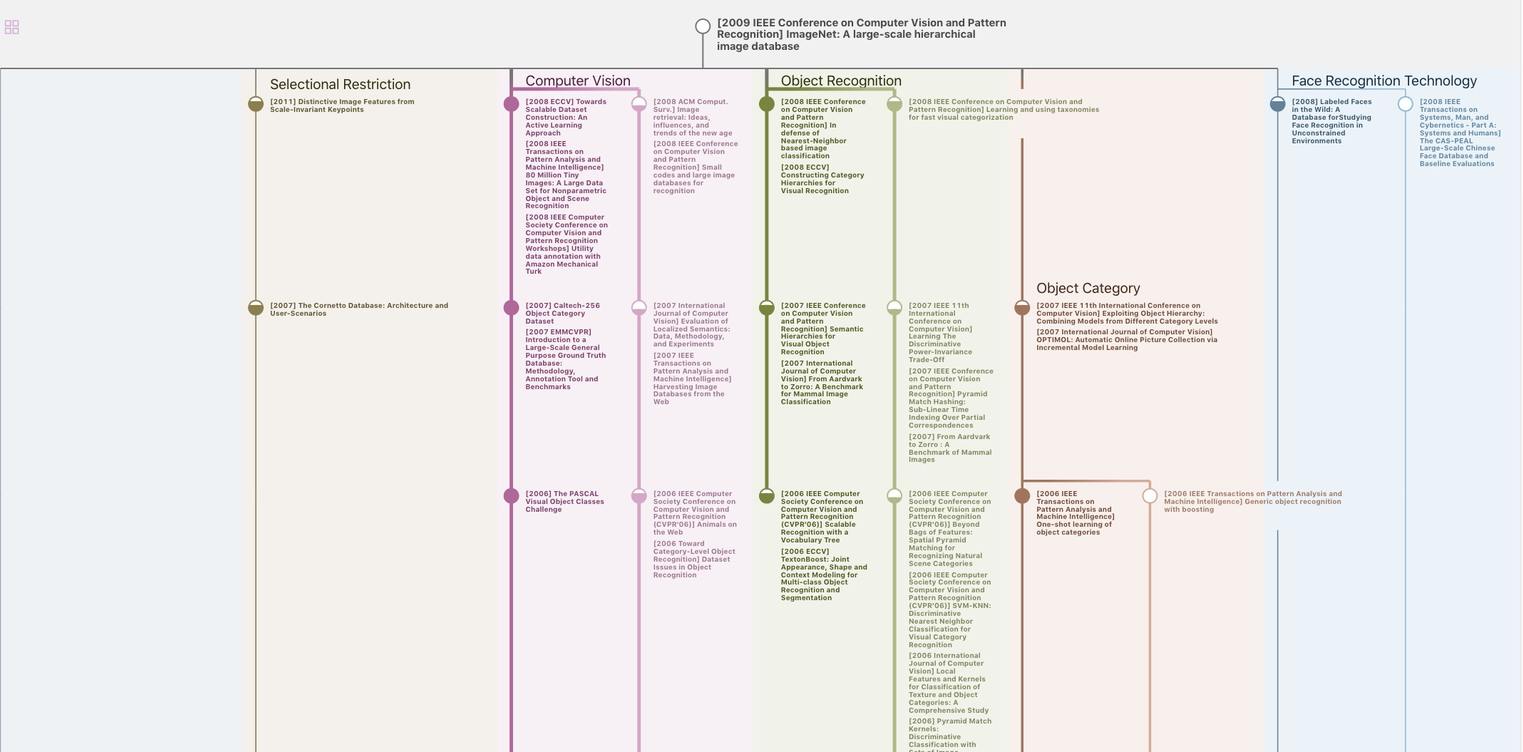
生成溯源树,研究论文发展脉络
Chat Paper
正在生成论文摘要