Mitigating Behavioral Variability for Mouse Dynamics: A Dimensionality-Reduction-Based Approach
IEEE T. Human-Machine Systems(2014)
摘要
Mouse dynamics is the process of identifying individual users on the basis of their mouse operating behaviors. Mouse dynamics analysis techniques do not provide an acceptable level of accuracy, perhaps due to behavioral variability. This study presents a dimensionality-reduction-based approach to mitigate the behavioral variability of mouse dynamics and improve the performance of mouse-dynamics-based continuous authentication. Variability was measured over the schematic features and motor-skill features extracted from each mouse behavior data session. A unified framework of employing dimensionality reduction methods (Multidimensional Scaling, Laplacian Eigenmap, Isometric Feature Mapping, and Local Linear Embedding) was developed to reduce behavioral variability by obtaining predominant characteristics from the original feature space. Classification techniques (Random Forest, Support Vector Machine, Neural Network, and Nearest Neighbor) were applied to the transformed feature space to perform the authentication task. Analyses were conducted using data from 840 half-hour sessions of 28 participants. Results indicated that for sufficiently long sequences, the transformed feature spaces had much less variability and the corresponding authentication performance was better than the original feature space with improvements of the false-acceptance rate by 89.6% and of the false-rejection rate by 77.4% in some cases. Additionally, an investigation of the relationships between variability and authentication error rates and detection time indicated that the variability and authentication error rates reduce greatly with the increase of detection time. For the data collected, the approach fared better than the state-of-the-art approaches. These findings suggest that variability reduction could improve mouse dynamics, so it may enhance current authentication mechanisms.
更多查看译文
关键词
motor-skill feature extraction,mouse controllers (computers),false-acceptance rate,neural network,behavioral variability mitigation,data reduction,random forest,classification techniques,schematic feature extraction,continuous authentication,authentication error rates,mouse dynamics,false-rejection rate,pattern classification,isometric feature mapping,dimensionality reduction,multidimensional scaling,behavioral variability,local linear embedding,variability reduction,biometrics (access control),feature extraction,mouse-dynamics-based continuous authentication,support vector machine,nearest neighbor,dimensionality-reduction-based approach,laplacian eigenmap,support vector machines,neural nets,mouse dynamics analysis techniques,data collection,authentication
AI 理解论文
溯源树
样例
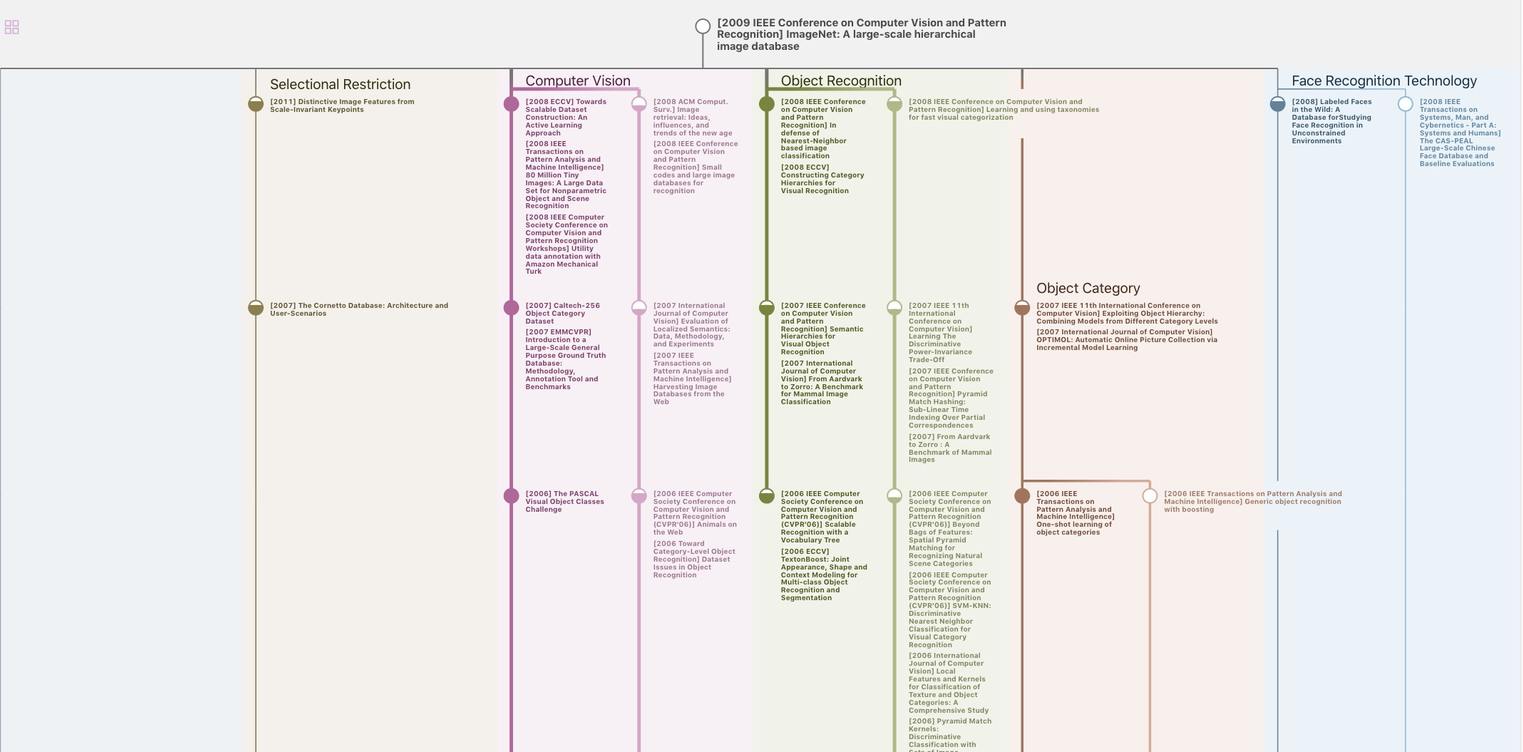
生成溯源树,研究论文发展脉络
Chat Paper
正在生成论文摘要