Sparse coding for speech recognition
Acoustics Speech and Signal Processing(2010)
摘要
This paper proposes a novel feature extraction technique for speech recognition based on the principles of sparse coding. The idea is to express a spectro-temporal pattern of speech as a linear combination of an overcomplete set of basis functions such that the weights of the linear combination are sparse. These weights (features) are subsequently used for acoustic modeling. We learn a set of overcomplete basis functions (dictionary) from the training set by adopting a previously proposed algorithm which iteratively minimizes the reconstruction error and maximizes the sparsity of weights. Furthermore, features are derived using the learned basis functions by applying the well established principles of compressive sensing. Phoneme recognition experiments show that the proposed features outperform the conventional features in both clean and noisy conditions.
更多查看译文
关键词
feature extraction,speech coding,speech recognition,acoustic modeling,compressive sensing,feature extraction technique,overcomplete basis functions,phoneme recognition,sparse coding,spectro temporal speech pattern,speech recognition,compressive sensing,feature extraction,sparse coding,speech recognition
AI 理解论文
溯源树
样例
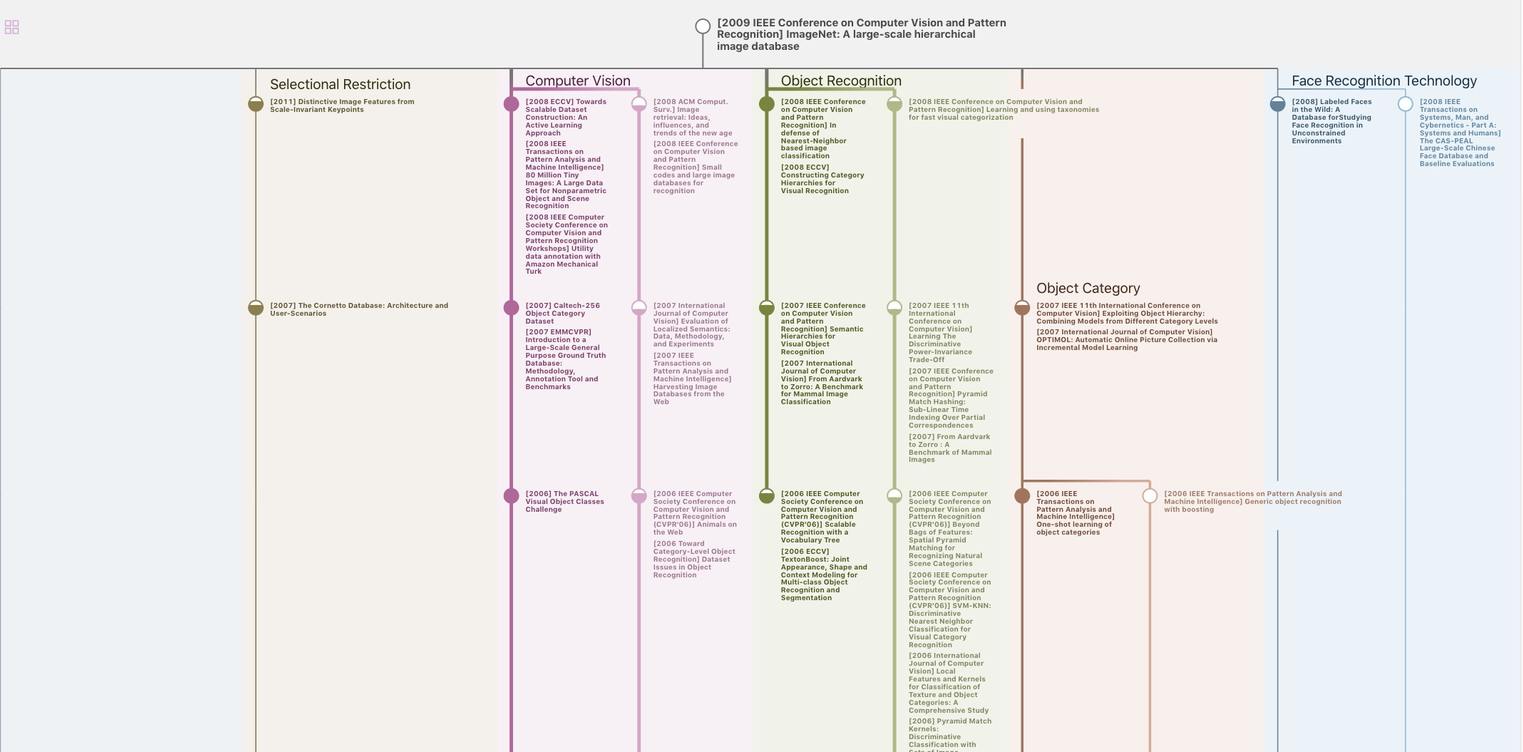
生成溯源树,研究论文发展脉络
Chat Paper
正在生成论文摘要