Sparse Bayesian kernel logistic regression
ESANN(2004)
摘要
In this paper we present a simple hierarchical Bayesian treatment of the sparse kernel logistic regression (KLR) model based MacKay's evidence approximation. The model is re-parameterised such that an isotropic Gaussian prior over parameters in the kernel induced feature space is replaced by an isotropic Gaussian prior over the trans- formed parameters, facilitating a Bayesian analysis using standard meth- ods. The Bayesian approach allows the selection of "good" values for the usual regularisation and kernel parameters through maximisation of the marginal likelihood. Results obtained on a variety of benchmark datasets are provided indicating that the Bayesian kernel logistic regres- sion model is competitive, whilst having one less parameter to determine during model selection.
更多查看译文
关键词
bayesian analysis,bayesian approach,feature space,marginal likelihood,model selection
AI 理解论文
溯源树
样例
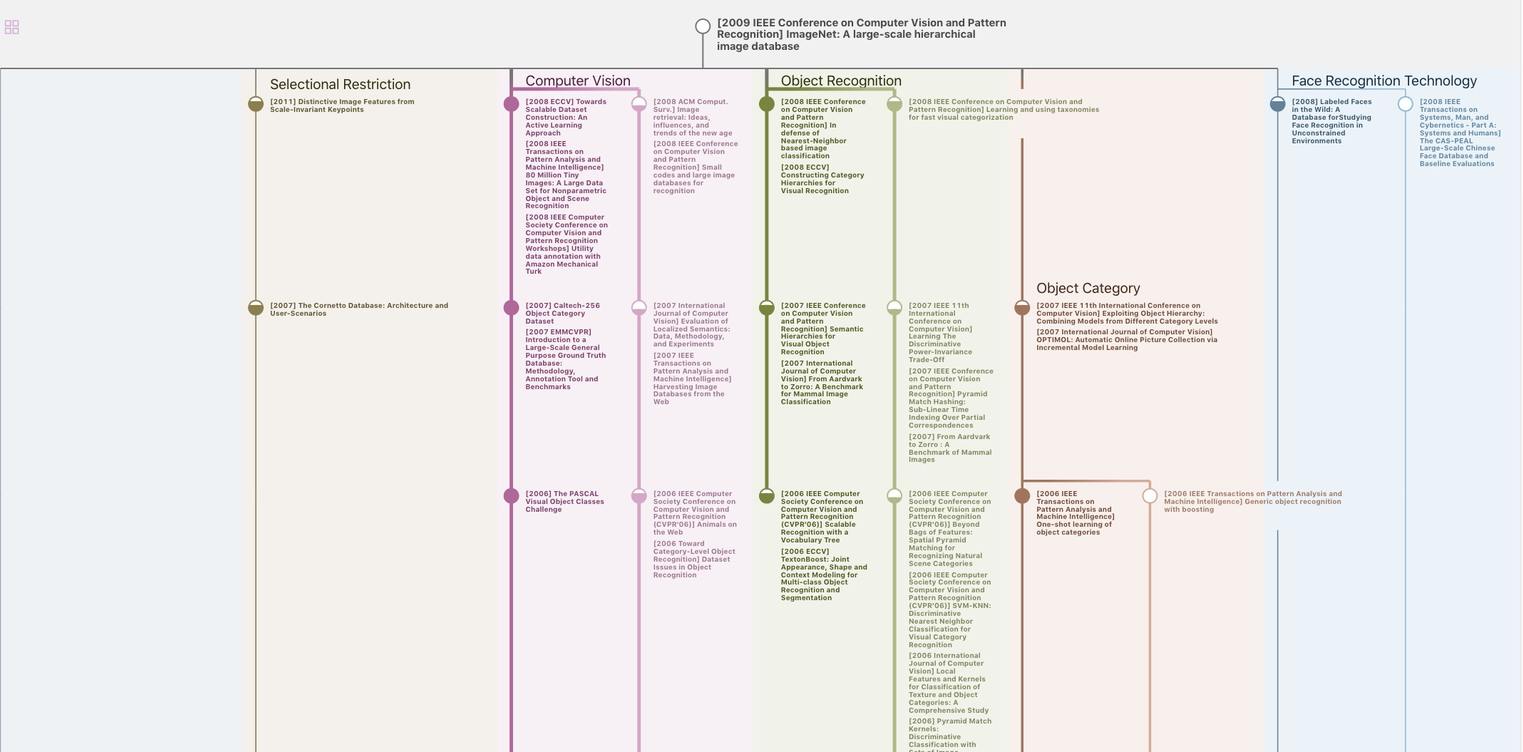
生成溯源树,研究论文发展脉络
Chat Paper
正在生成论文摘要