Integration and differentiation in dynamic recurrent neural networks
Neural Computation(1994)
摘要
Dynamic neural networks with recurrent connections were trained by backpropagation to generate the differential or the leaky integral of a nonrepeating frequency-modulated sinusoidal signal. The trained networks performed these operations on arbitrary input waveforms. Reducing the network size by deleting ineffective hidden units and combining redundant units, and then retraining the network produced a minimal network that computed the same function and revealed the underlying computational algorithm. Networks could also be trained to compute simultaneously the differential and integral of the input on two outputs; the two operations were performed in distributed overlapping fashion, and the activations of the hidden units were dominated by the integral. Incorporating units with time constants into model networks generally enhanced their performance as integrators and interfered with their ability to differentiate.
更多查看译文
关键词
dynamic recurrent neural network,network size,model network,minimal network,ineffective hidden unit,arbitrary input waveform,trained network,dynamic neural network,nonrepeating frequency-modulated sinusoidal signal,hidden unit,incorporating unit,recurrent neural network,time constant,backpropagation,network performance
AI 理解论文
溯源树
样例
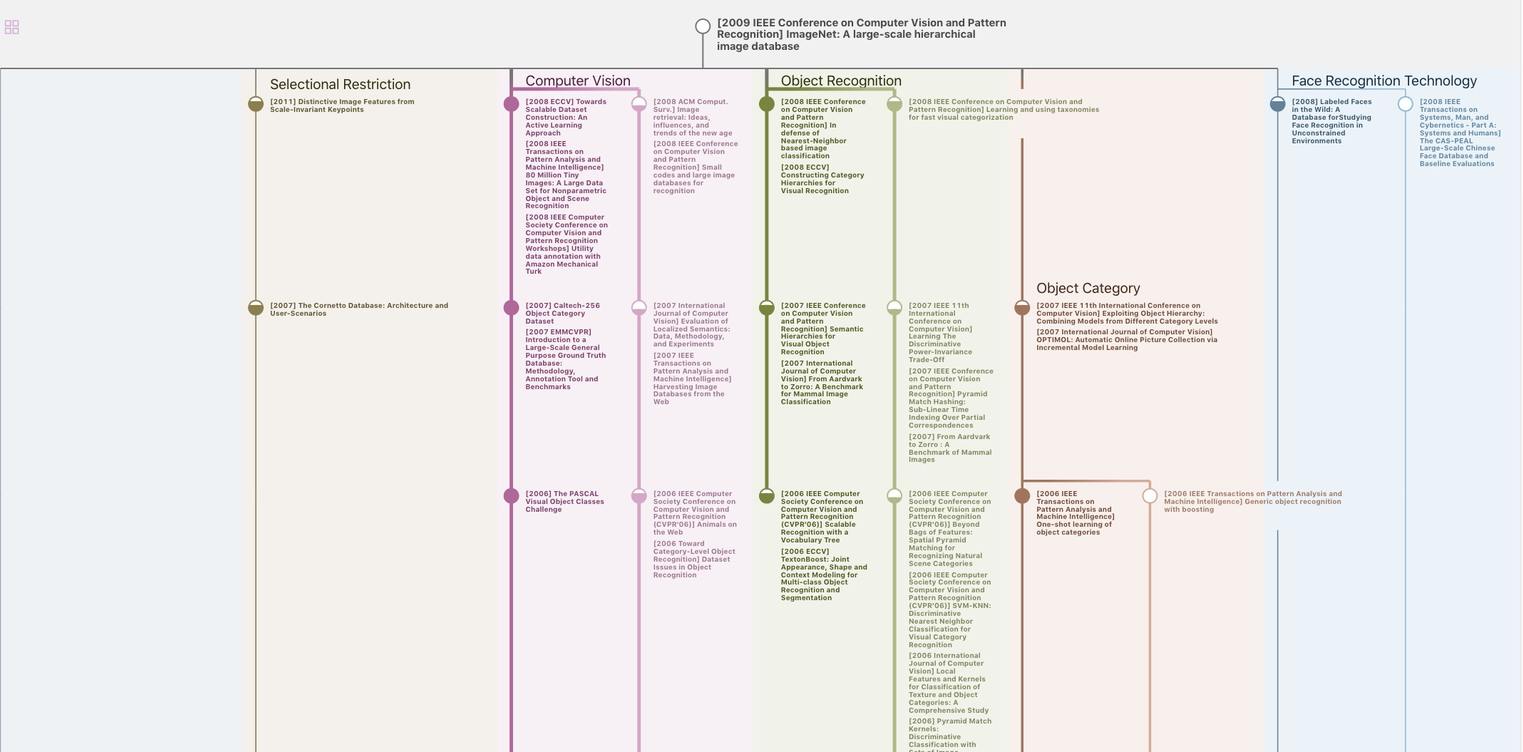
生成溯源树,研究论文发展脉络
Chat Paper
正在生成论文摘要