MULTIPLE-EVENT LOCATION USING THE MARKOV-CHAIN MONTE CARLO TECHNIQUE
msra(2005)
摘要
The goal of next-generation seismic location is to ascertain a consistent set of event locations and travel-time corrections through simultaneous analysis of all relevant data. Towards that end, we are developing a new multiple-event location algorithm that utilizes the Markov-Chain Monte Carlo (MCMC) method for solving large, non-linear inverse problems. Unlike most inverse methods, the MCMC approach produces a suite of solutions, each of which is consistent with seismic and other observations, as well as prior estimates of data and model uncertainties. In the MCMC multiple-event locator (MCMCloc), the model uncertainties consist of prior estimates on the accuracy of each input event location, travel-time prediction uncertainties, phase measurement uncertainties, and assessments of phase identification. The prior uncertainty estimates include correlations between travel-time predictions, correlations between measurement errors, and the probability of misidentifying one phase for another (or bogus picks). The implementation of prior constraints on location accuracy allows the direct utilization of ground-truth events in the location algorithm. This is a significant improvement over most other grid multiple-event locators (GMEL is an exception), for which location accuracy is achieved through post-processing comparisons with ground-truth information. Like the double-difference algorithm, the implementation of a correlation structure for travel-time predictions allows MCMCloc to operate over arbitrarily large geographic areas. MCMCloc can accommodate non-Gaussian and multi-modal pick distributions, which can enhance application to poorly recorded events. Further, MCMCloc allows for ambiguous determination of phase assignments, and the solution includes the probability that phases are properly assigned. The probabilities that phase assignments are correct are propagated to the estimates of all other model parameters. Posteriori estimates of event locations, path corrections, pick errors, and phase identifications are made through analysis of the posteriori suite of acceptable solutions. We test the MCMC locator on a regional data set of Nevada Test Site nuclear explosions. Event locations and origin times are known for these events, allowing us to tests the features of MCMCloc against a true ground truth (GT0) data set. Preliminary tests suggest that MCMCloc provides excellent relative locations (similar to other algorithms), and excellent absolute locations when constraints from one or more ground truth event are included. Tests also include realistic phase misidentification, where phase assignments are switched for phases that arrive within a few seconds of one another. We find that MCMCloc is a promising method for simultaneously locating large, geographically distributed data sets. Because we allow for input of prior knowledge on many aspects of the data set, MCMCloc is ideal for combining trusted and lesser-quality data.
更多查看译文
关键词
origin,forecasting,geosciences,probability,accuracy,algorithms,markov chain monte carlo,ground truth,measurement error,implementation,inverse problem
AI 理解论文
溯源树
样例
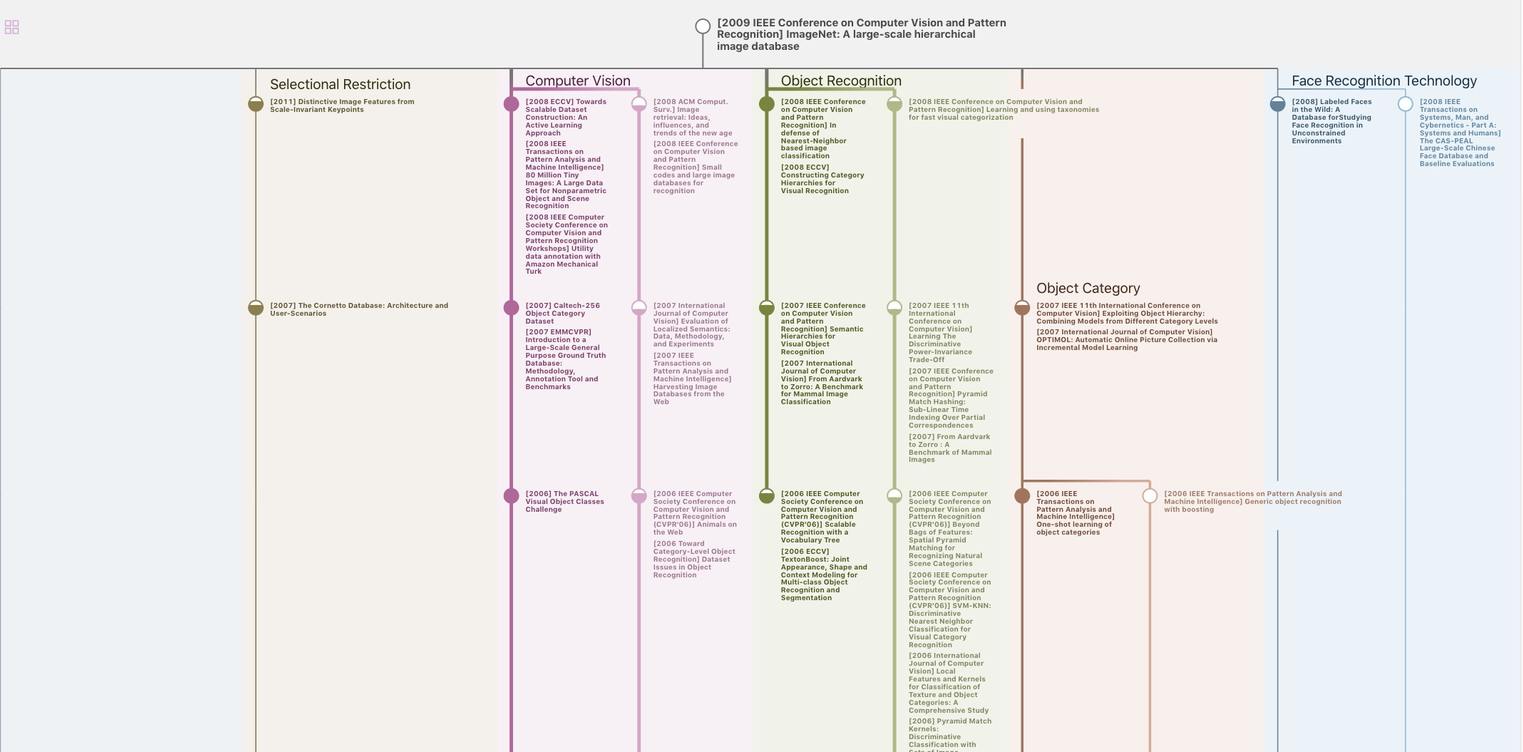
生成溯源树,研究论文发展脉络
Chat Paper
正在生成论文摘要