Recommending with an Agenda: Active Learning of Private Attributes using Matrix Factorization
RECSYS(2014)
摘要
Recommender systems leverage user demographic information, such as age, gender, etc., to personalize recommendations and better place their targeted ads. Oftentimes, users do not volunteer this information due to privacy concerns, or due to a lack of initiative in filling out their online profiles. We illustrate a new threat in which a recommender learns private attributes of users who do not voluntarily disclose them. We design both passive and active attacks that solicit ratings for strategically selected items, and could thus be used by a recommender system to pursue this hidden agenda. Our methods are based on a novel usage of Bayesian matrix factorization in an active learning setting. Evaluations on multiple datasets illustrate that such attacks are indeed feasible and use significantly fewer rated items than static inference methods. Importantly, they succeed without sacrificing the quality of recommendations to users.
更多查看译文
关键词
Recommendations,Privacy,Active Learning
AI 理解论文
溯源树
样例
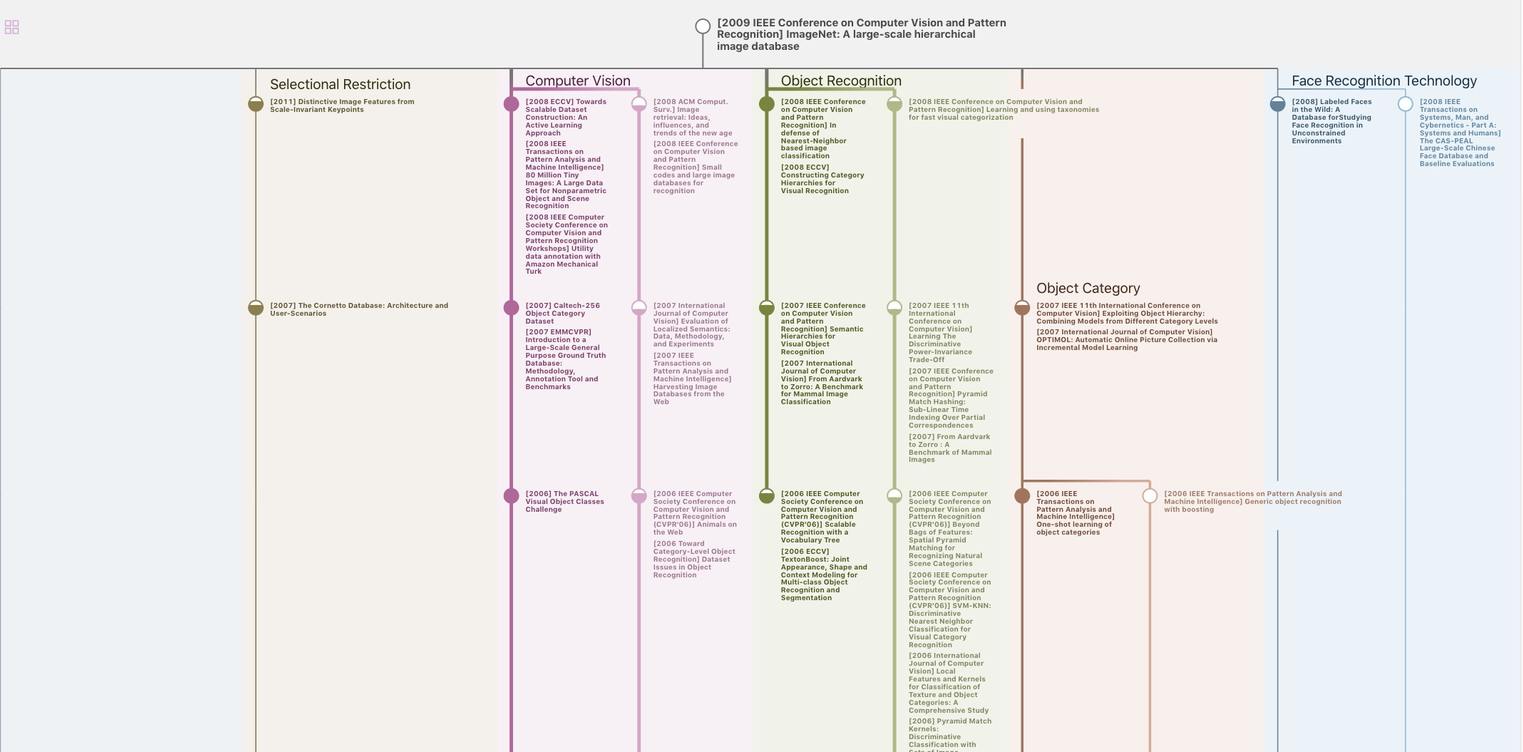
生成溯源树,研究论文发展脉络
Chat Paper
正在生成论文摘要