A general metric and parallel framework for adaptive image fusion in clusters (vol 26, pg 1375, 2014)
Concurrency and Computation: Practice & Experience(2015)
摘要
This article is dedicated to techniques and theories of image fusion in automatic ways and addresses two issues-the parameter setting and quality assessment. Optimal parameters are in demand for specific applications or comparison between fusion methods because, as basic evidence, different parameters bring different fusion effects varying over a large range. In this paper, we propose a general framework of online parameter training to search optimal values that best suit input images. Furthermore, we optimized the compute-intensive training process using parallelization and genetic algorithm, as well as patches extraction. We also propose a metric-spatial and spectral distortion-as the learning target. The spatial and spectral distortion is a fuzzy combination of mean potential energy measuring spatial distortion and Q4 measuring spectral distortion. Optimization validation on weighted Gram-Schmidt fusion indicated linear or superlinear acceleration ability, which proved that the proposed learning framework can speed up the learning process of image fusion to an acceptable time, and can thus be applied to high-performance platforms to process large volumes of data. Copyright © 2013 John Wiley & Sons, Ltd.
更多查看译文
关键词
parallel processing,genetic algorithms,Markov random fields,remote sensing image processing
AI 理解论文
溯源树
样例
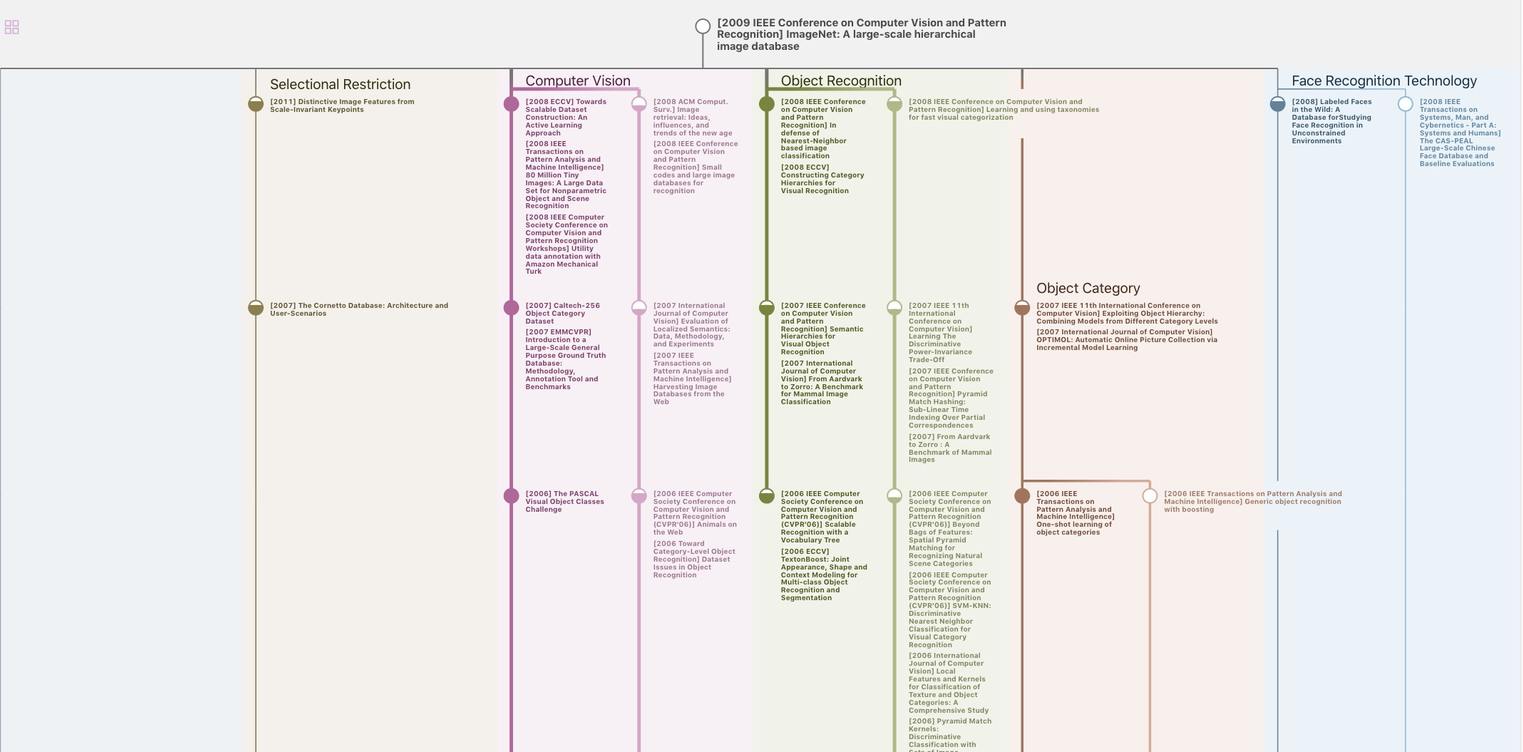
生成溯源树,研究论文发展脉络
Chat Paper
正在生成论文摘要