Bacterial Foraging Optimization Based Least Square Support Vector Machine for Short-Term Electricity Load Forecasting
2024 International Conference on Green Energy, Computing and Sustainable Technology (GECOST)(2024)
摘要
The impact of the COVID-19 pandemic has been related to energy consumption in Peninsular Malaysia due to the imposition of the Movement Control Order in March 2020. Consequently, energy consumption in residential buildings has risen while in the services and industrial sectors has reduced tremendously, causing electricity consumption demand to fall to Sunday consumption levels. The highly stochastic nature of electricity demand poses increasing challenges for power utilities in maintaining demand-supply balance, particularly in the context of high forecast errors. Thus, a thorough understanding of electricity demand patterns and their influence on load forecasting is essential for ensuring the reliable operation of the electrical grid. Several studies published have shown that the single load forecasting model such as neural network and support vector machine has relatively low prediction accuracy compared to the hybrid method. Thus, this study aims to examine the suitability and performance of the Bacterial Foraging Optimization Algorithm integration with the Least Square Support Vector Machine to predict a day-ahead load in Peninsular Malaysia during the pandemic. The mean absolute percentage error as the obj ective function of the prediction model obtained ranges from 3.17% to 4.99% considering 5 day-type in a week.
更多查看译文
关键词
short-term load forecasting,least square support vector machine,bacterial foraging optimization algorithm,machine learning,power system
AI 理解论文
溯源树
样例
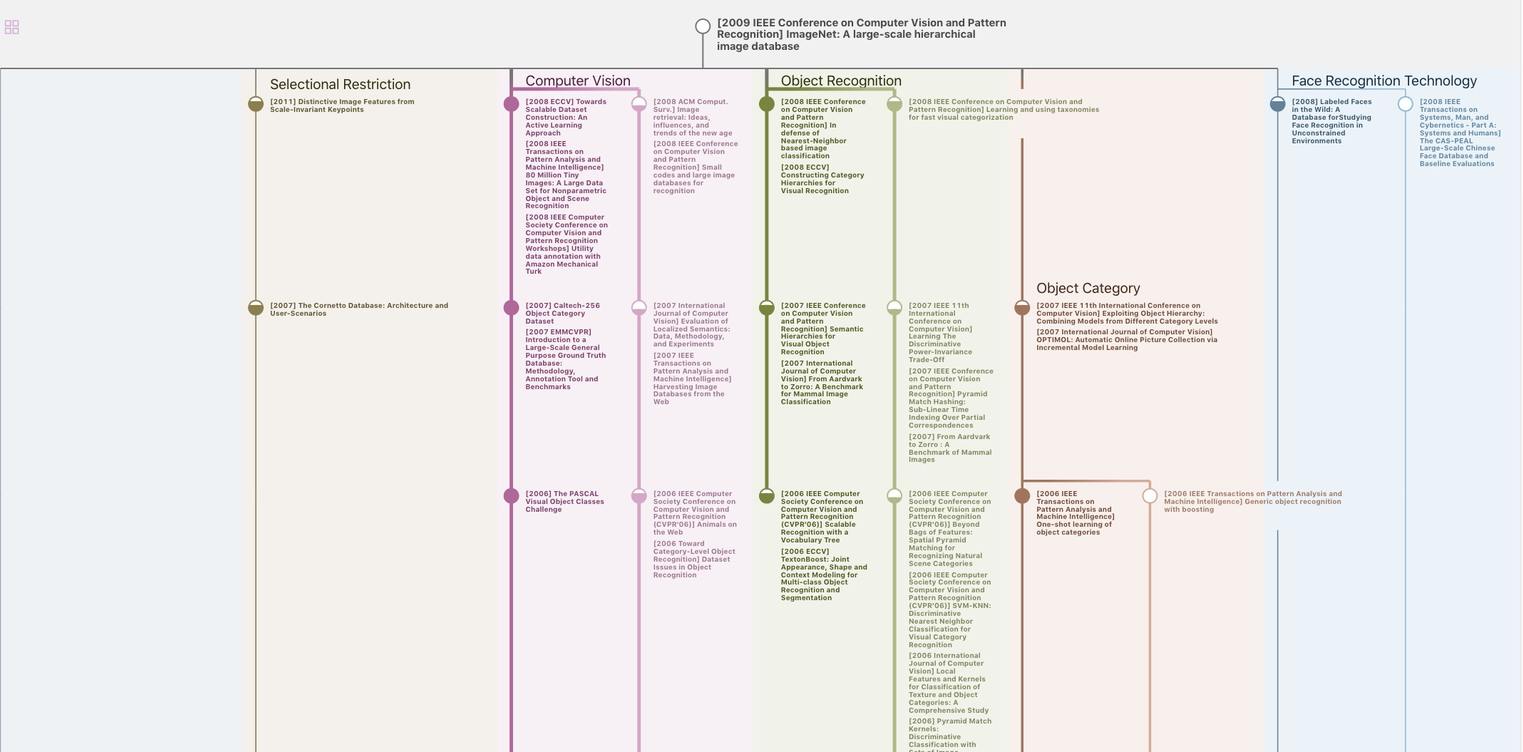
生成溯源树,研究论文发展脉络
Chat Paper
正在生成论文摘要