Adaptor Grammars: A Framework for Specifying Compositional Nonparametric Bayesian Models
NIPS(2006)
摘要
This paper introduces adaptor grammars, a class of probabil istic models of lan- guage that generalize probabilistic context-free grammar s (PCFGs). Adaptor grammars augment the probabilistic rules of PCFGs with "adaptors" that can in- duce dependencies among successive uses. With a particular choice of adaptor, based on the Pitman-Yor process, nonparametric Bayesian models of language using Dirichlet processes and hierarchical Dirichlet proc esses can be written as simple grammars. We present a general-purpose inference algorithm for adaptor grammars, making it easy to define and use such models, and ill ustrate how several existing nonparametric Bayesian models can be expressed within this framework.
更多查看译文
关键词
bayesian model
AI 理解论文
溯源树
样例
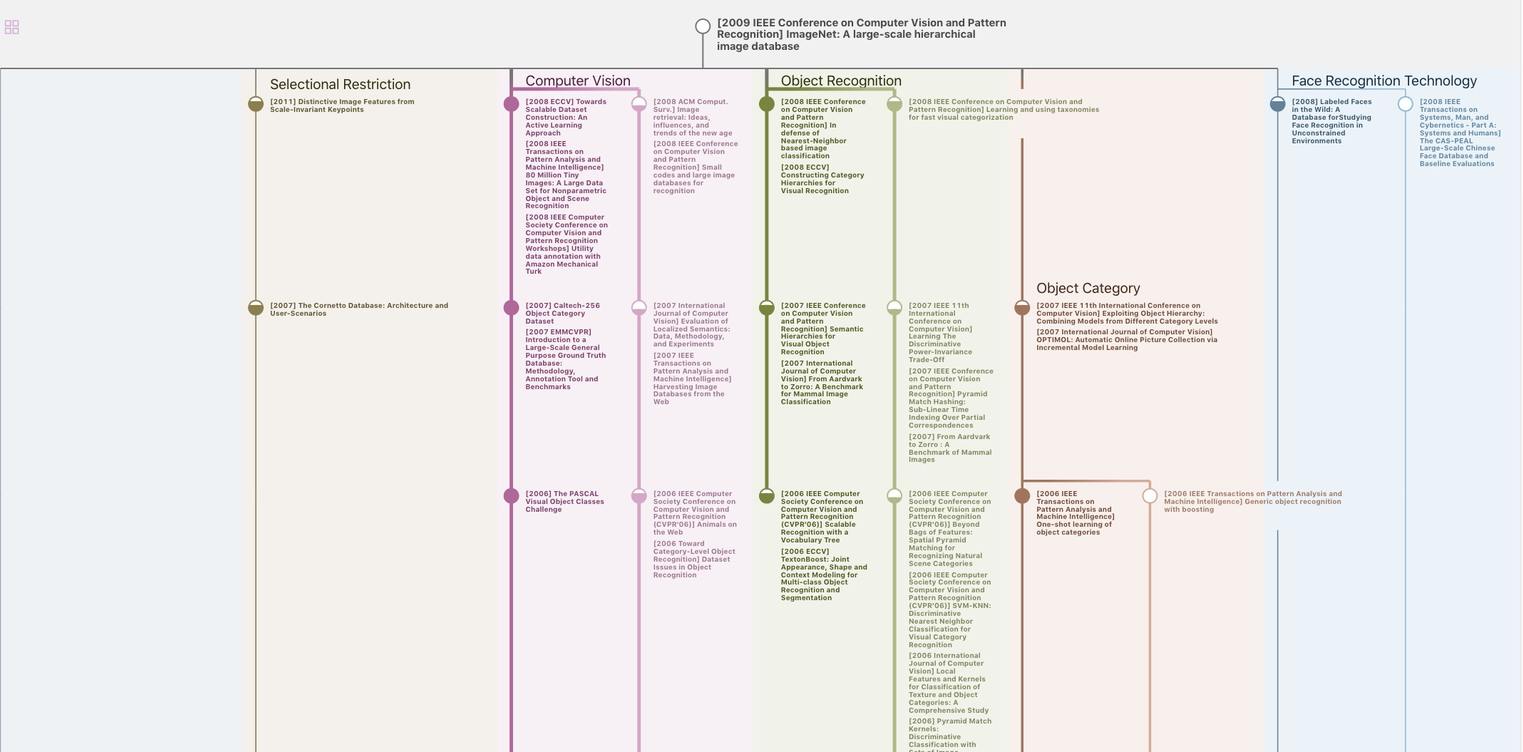
生成溯源树,研究论文发展脉络
Chat Paper
正在生成论文摘要