Robust optimization of factor graphs by using condensed measurements
IROS(2012)
摘要
Popular problems in robotics and computer vision like simultaneous localization and mapping (SLAM) or structure from motion (SfM) require to solve a least-squares problem that can be effectively represented by factor graphs. The chance to find the global minimum of such problems depends on both the initial guess and the non-linearity of the sensor models. In this paper we propose an approach to determine an approximation of the original problem that has a larger convergence basin. To this end, we employ a divide-and-conquer approach that exploits the structure of the factor graph. Our approach has been validated on real-world and simulated experiments and is able to succeed in finding the global minimum in situations where other state-of-the-art methods fail.
更多查看译文
关键词
robust optimization,optimisation,factor graphs,global minimum,simultaneous localization and mapping,sensor model nonlinearity,slam,approximation determination,robotics,divide and conquer approach,least squares approximations,image sensors,structure from motion,slam (robots),computer vision,graph theory,least squares problem,sfm,condensed measurements,divide and conquer methods,robot vision
AI 理解论文
溯源树
样例
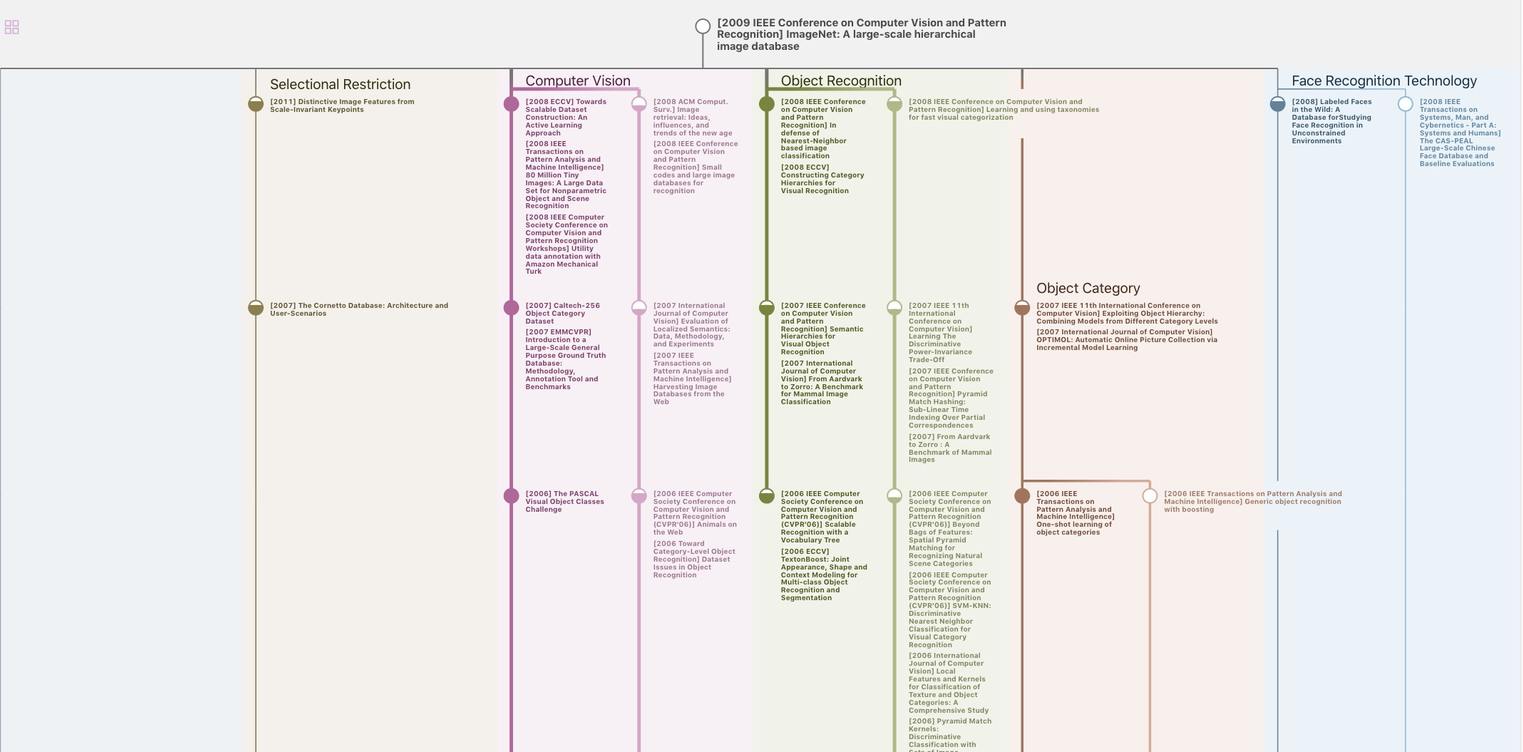
生成溯源树,研究论文发展脉络
Chat Paper
正在生成论文摘要