The Value Of User Feedback
ECIR 2011: Proceedings of the 33rd European Conference on Advances in Information Retrieval - Volume 6611(2011)
摘要
Information retrieval systems and their users engage in a dialogue. While the main flow of information is from the system to the user, feedback from the user to the system provides many opportunities for short-term and long-term learning. In this talk, I will explore two interrelated questions that are central to the effective use of feedback. First, how can user feedback be collected so that it does not lay a burden on the user? I will argue that the mechanisms for collecting feedback have to be integrated into the design of the retrieval process, so that the user's short-term goals are well-aligned with the system's goal of collecting feedback. Second, if this integration succeeds, how valuable is the information that user feedback provides? For the tasks of retrieval evaluation and query disambiguation, the talk will quantify by how much user feedback can save human annotator effort and improve retrieval quality respectively. Bio. Thorsten Joachims is an Associate Professor in the Department of Computer Science at Cornell University. In 2001, he finished his dissertation with the title "The Maximum-Margin Approach to Learning Text Classifiers: Methods, Theory, and Algorithms", advised by Prof. Katharina Morik at the University of Dortmund. From there he also received his Diplom in Computer Science in 1997 with a thesis on WebWatcher, a browsing assistant for the Web. From 1994 to 1996 he was a visiting scientist at Carnegie Mellon University with Prof. Tom Mitchell. His research interests center on a synthesis of theory and system building in the field of machine learning, with a focus on support vector machines, structured output prediction, and learning algorithms for information retrieval.
更多查看译文
关键词
user feedback,information retrieval system,retrieval evaluation,retrieval process,retrieval quality,short-term goal,effective use,human annotator effort,interrelated question,long-term learning
AI 理解论文
溯源树
样例
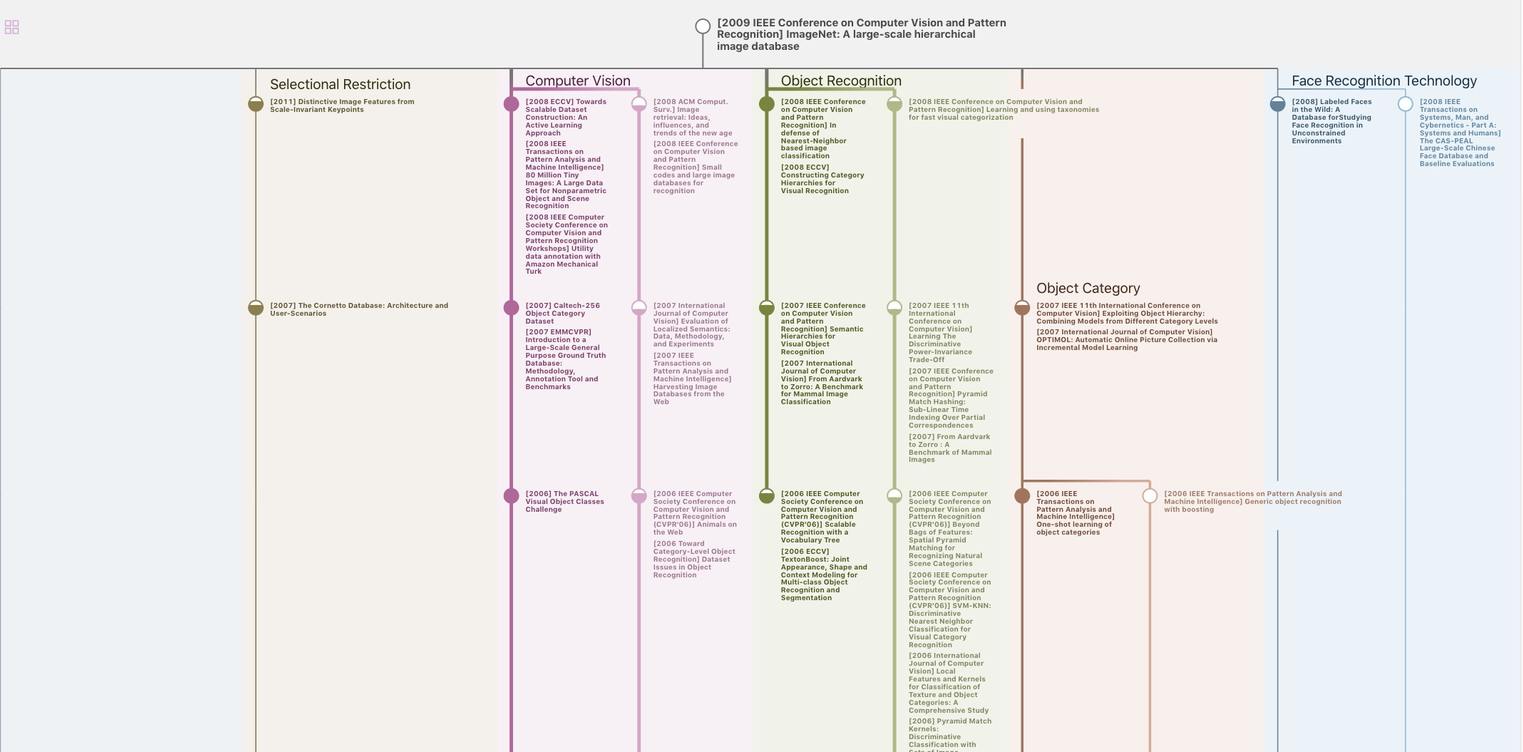
生成溯源树,研究论文发展脉络
Chat Paper
正在生成论文摘要