Detection and Length Measurement of Cracks Captured in Low Definitions Using Convolutional Neural Networks.
Sensors(2023)
摘要
Continuous efforts were made in detecting cracks in images. Varied CNN models were developed and tested for detecting or segmenting crack regions. However, most datasets used in previous works contained clearly distinctive crack images. No previous methods were validated on blurry cracks captured in low definitions. Therefore, this paper presented a framework of detecting the regions of blurred, indistinct concrete cracks. The framework divides an image into small square patches which are classified into crack or non-crack. Well-known CNN models were employed for the classification and compared with each other with experimental tests. This paper also elaborated on critical factors-the patch size and the way of labeling patches-which had considerable influences on the training performance. Furthermore, a series of post-processes for measuring crack lengths were introduced. The proposed framework was tested on the images of bridge decks containing blurred thin cracks and showed reliable performance comparable to practitioners.
更多查看译文
关键词
deep learning,concrete crack,convolutional neural network,low definition crack image,length measurement
AI 理解论文
溯源树
样例
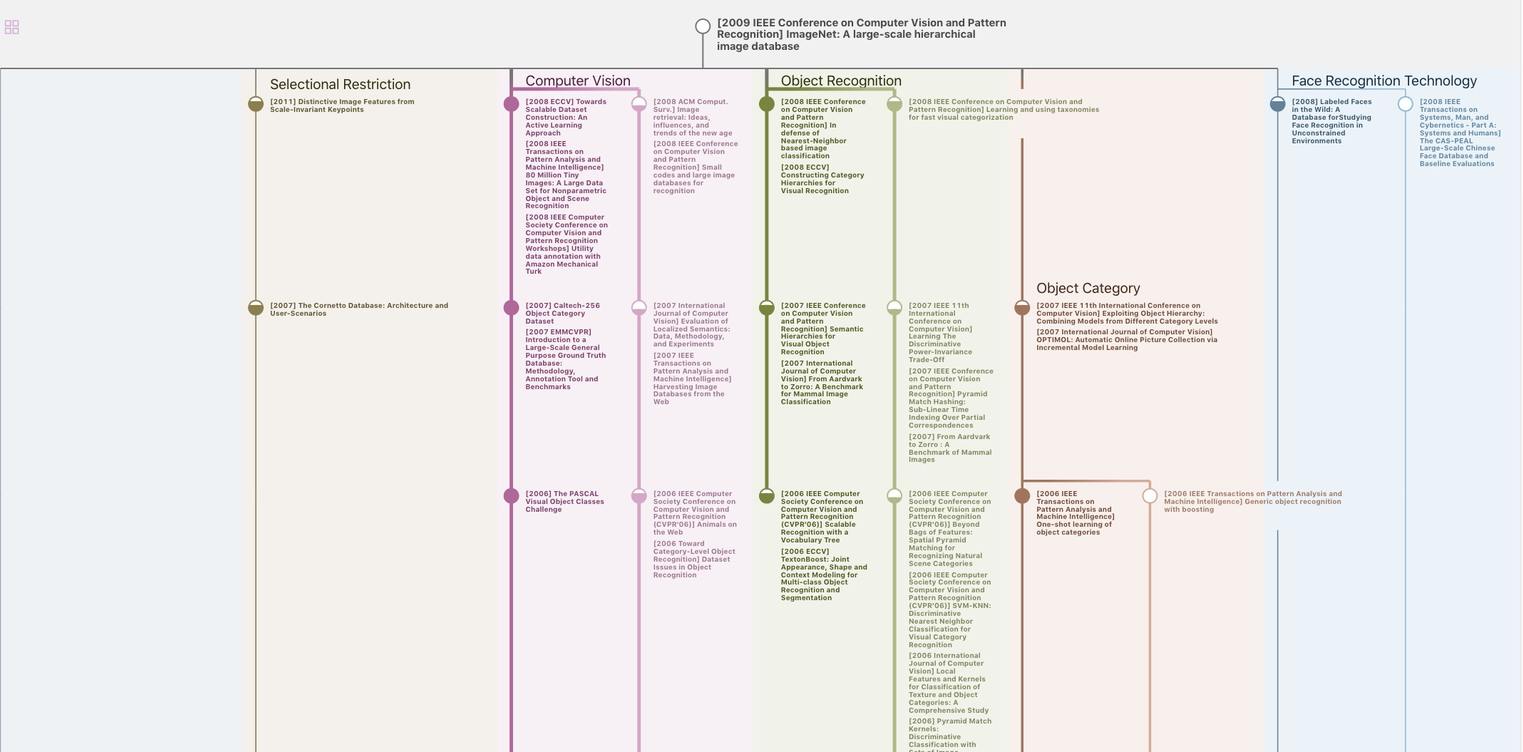
生成溯源树,研究论文发展脉络
Chat Paper
正在生成论文摘要