Understanding how people interact with web search results that change in real-time using implicit feedback.
CIKM'13: 22nd ACM International Conference on Information and Knowledge Management San Francisco California USA October, 2013(2013)
摘要
The way a searcher interacts with query results can reveal a lot about what is being sought. Considerable research has gone into using implicit relevance feedback to identify relevant con-tent in real-time, but little is known about how to best present this newly identified relevant content to users. In this paper we compare a traditional search interface with one that dynamical-ly re-ranks and recommends search results as the user interacts with it in order to build a picture of how and when users should be offered dynamically identified relevant content. We present several studies that compare logged behavior for hun-dreds of thousands of users and millions of queries as well as self-reported measures of success across the two interaction models. Compared to traditional web search, users presented with dynamically ranked results exhibit higher engagement and find information faster, particularly during exploratory tasks. These findings have implications for how search engines might best exploit implicit feedback in real-time in order to help users identify the most relevant results as quickly as possible.
更多查看译文
AI 理解论文
溯源树
样例
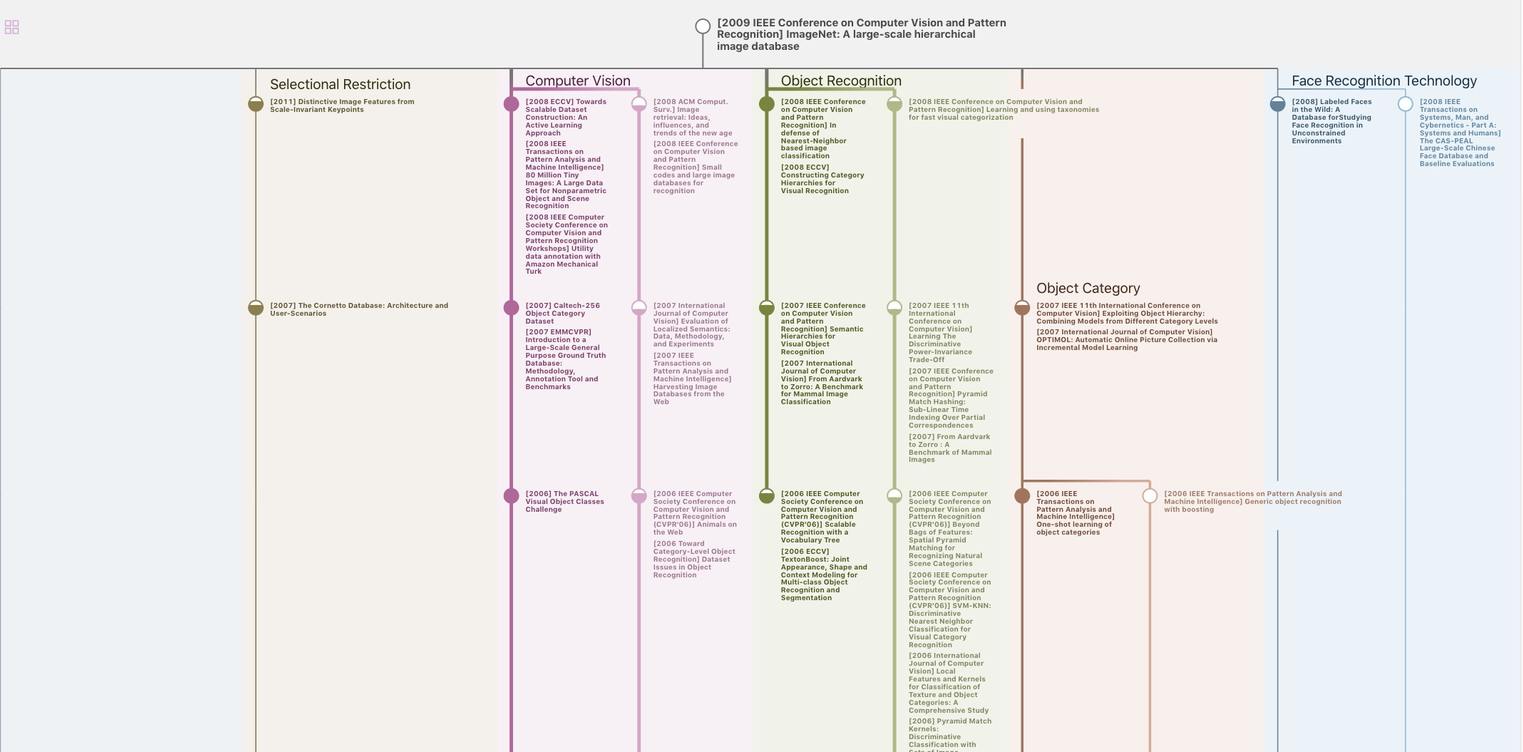
生成溯源树,研究论文发展脉络
Chat Paper
正在生成论文摘要