Effective and Efficient Clustering Methods for Correlated Probabilistic Graphs
IEEE Transactions on Knowledge and Data Engineering(2014)
摘要
Recently, probabilistic graphs have attracted significant interests of the data mining community. It is observed that correlations may exist among adjacent edges in various probabilistic graphs. As one of the basic mining techniques, graph clustering is widely used in exploratory data analysis, such as data compression, information retrieval, image segmentation, etc. Graph clustering aims to divide data into clusters according to their similarities, and a number of algorithms have been proposed for clustering graphs, such as the pKwikCluster algorithm, spectral clustering, k-path clustering, etc. However, little research has been performed to develop efficient clustering algorithms for probabilistic graphs. Particularly, it becomes more challenging to efficiently cluster probabilistic graphs when correlations are considered. In this paper, we define the problem of clustering correlated probabilistic graphs. To solve the challenging problem, we propose two algorithms, namely the \($PEED\) and the \($CPG\) clustering algorithm. For each of the proposed algorithms, we develop several pruning techniques to further improve their efficiency. We evaluate the effectiveness and efficiency of our algorithms and pruning methods through comprehensive experiments.
更多查看译文
关键词
efficient clustering method,pattern clustering,data analysis,peedr clustering algorithm,correlation theory,and association rules,pruning techniques,algorithm,probabilistic graphs,correlated,data mining,graph theory,classification,exploratory data analysis,adjacent edges,correlated probabilistic graph clustering,effective clustering method,cpgs clustering algorithm,clustering,correlation,clustering algorithms,probability,probabilistic logic,algorithm design and analysis
AI 理解论文
溯源树
样例
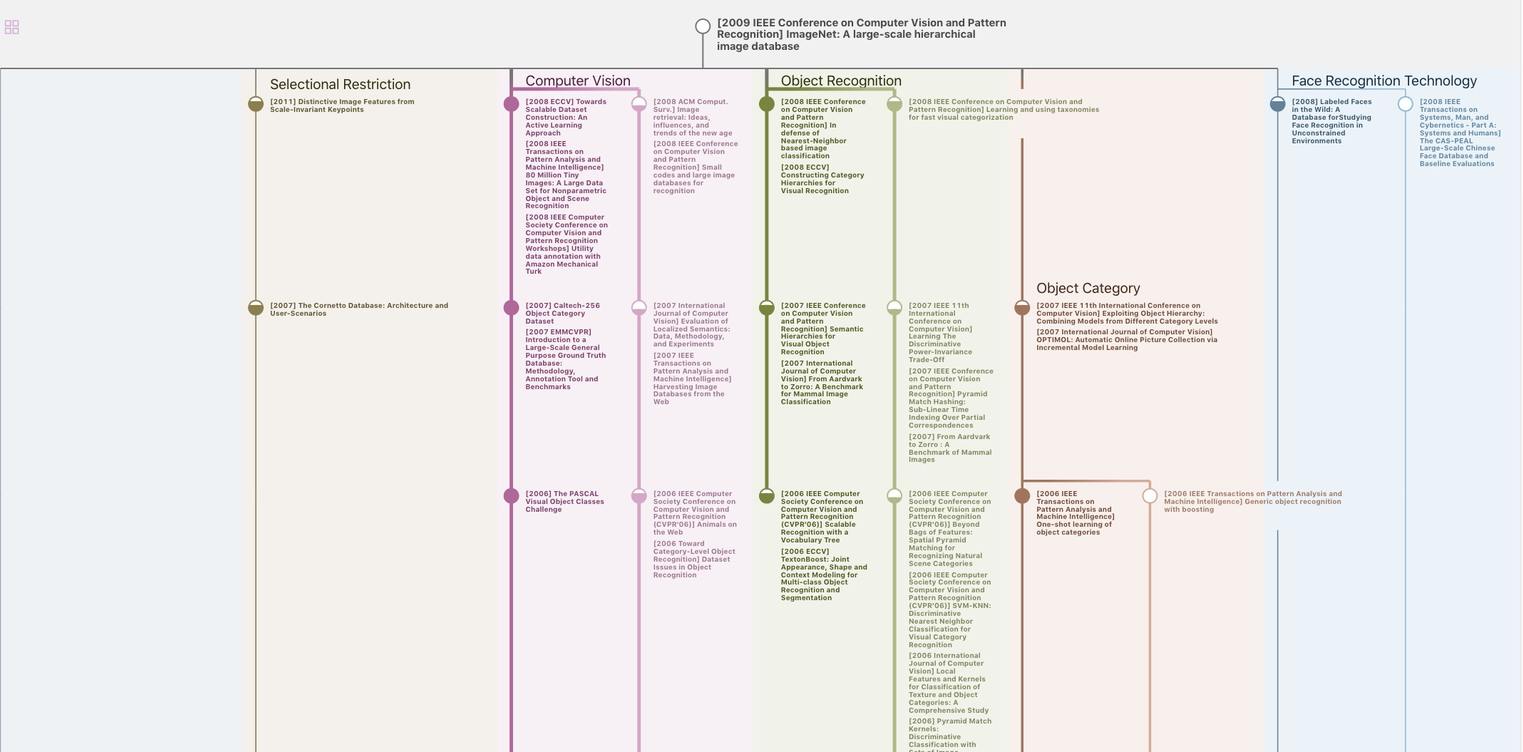
生成溯源树,研究论文发展脉络
Chat Paper
正在生成论文摘要