Automatic Annotation of Interactions in Meetings Using Semi-supervised Learning Techniques
msra
摘要
People spend many hours in meetings during their working lives. The growing need for help in keeping records in meetings and searching through them has been recognized, and several groups around the world are working on a meeting browser or a summarization tool. In this research, we propose the development of a classification system that uses machine learning techniques to segment and detect meeting acts, which are high-level interactions among meeting participants as a group (e.g. negotiation, reporting, discussion, planning). As in other data-driven tasks, this requires a large amount of data, but labeling data can be costly, time-consuming and errorprone. To address this problem, semi-supervised learning techniques are often applied, in which a small amount of data are labeled and is used to train a classifier together with a large body of unlabeled data. In this study, we propose to use and extend a novel semi-supervised learning algorithm, the contrast classifier approach, which exploits the contrast between the distributions of labeled and unlabeled data. We will also present our research plan to investigate the impact of different labeling mechanisms on the performance of existing and proposed semi-supervised learning techniques, especially in the presence of imbalanced class distribution.
更多查看译文
AI 理解论文
溯源树
样例
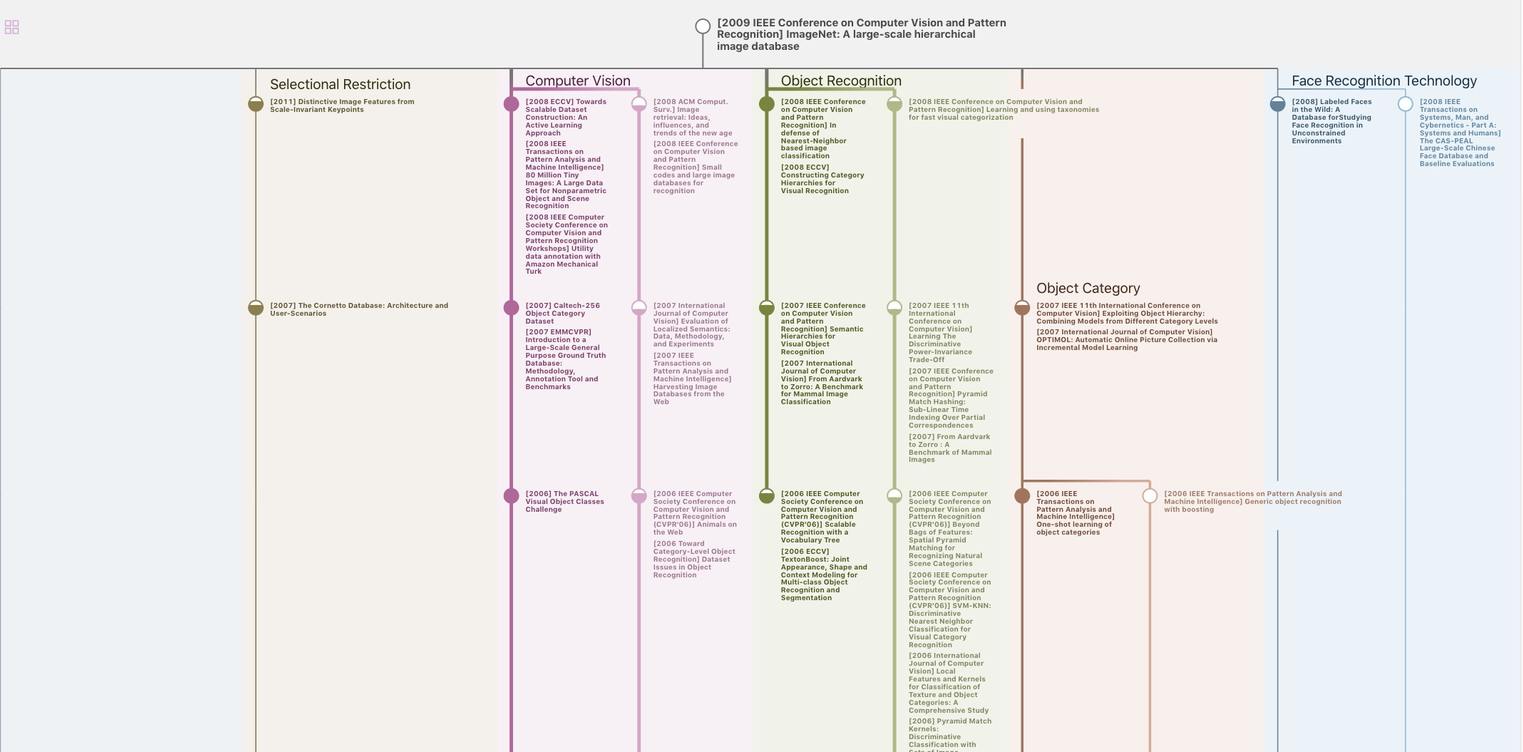
生成溯源树,研究论文发展脉络
Chat Paper
正在生成论文摘要