Learning a multiview part-based model in virtual world for pedestrian detection
Intelligent Vehicles Symposium(2013)
摘要
State-of-the-art deformable part-based models based on latent SVM have shown excellent results on human detection. In this paper, we propose to train a multiview deformable part-based model with automatically generated part examples from virtual-world data. The method is efficient as: (i) the part detectors are trained with precisely extracted virtual examples, thus no latent learning is needed, (ii) the multiview pedestrian detector enhances the performance of the pedestrian root model, (iii) a top-down approach is used for part detection which reduces the searching space. We evaluate our model on Daimler and Karlsruhe Pedestrian Benchmarks with publicly available Caltech pedestrian detection evaluation framework and the result outperforms the state-of-the-art latent SVM V4.0, on both average miss rate and speed (our detector is ten times faster).
更多查看译文
关键词
virtual world,pedestrians,virtual reality,deformable part-based models,pedestrian detection,pedestrian root model,svm,multiview pedestrian detector,object detection,driver information systems,daimler and karlsruhe pedestrian benchmarks,caltech pedestrian detection evaluation framework,human detection,part detection,multiview part-based model learning,support vector machines,detectors,testing,computational modeling
AI 理解论文
溯源树
样例
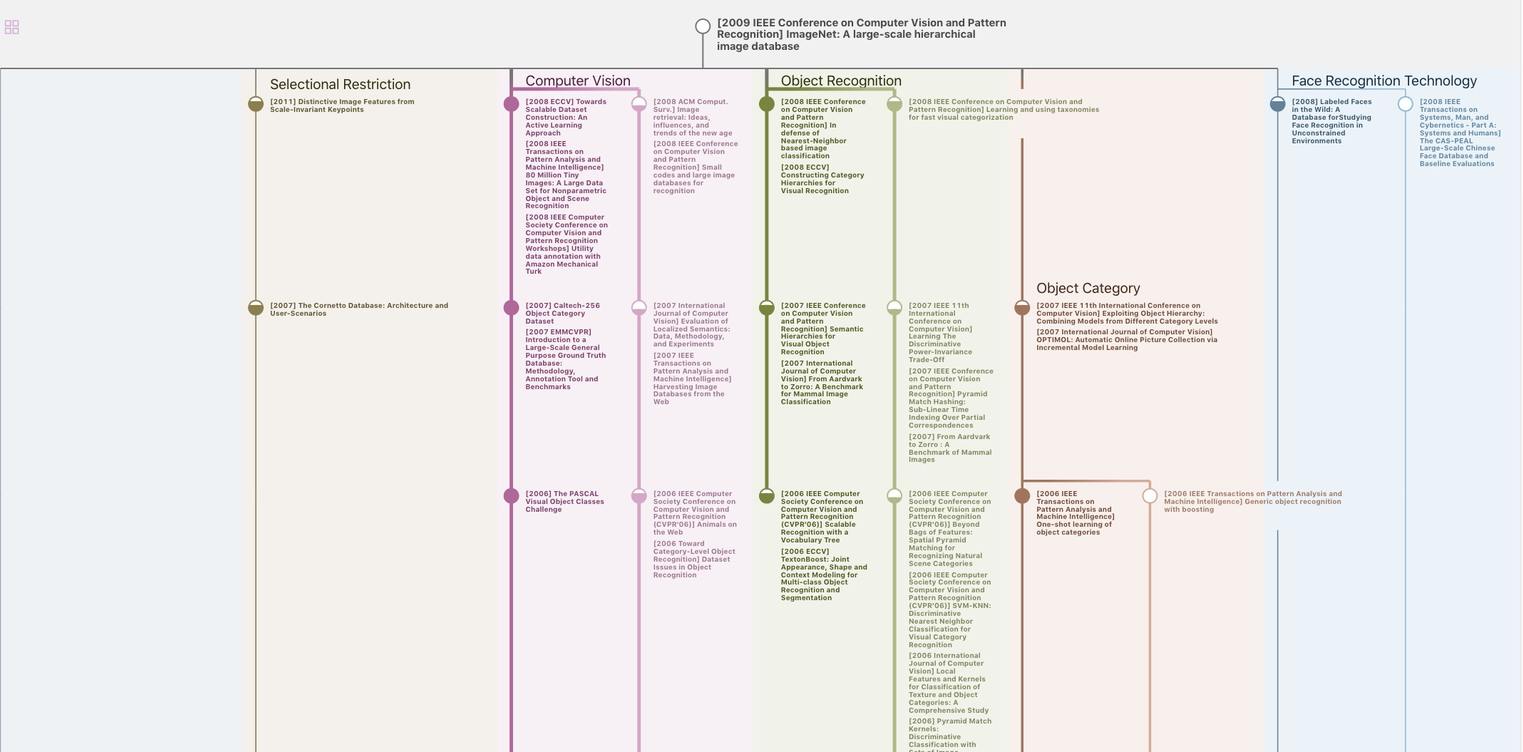
生成溯源树,研究论文发展脉络
Chat Paper
正在生成论文摘要