Quantitative Visually Lossless Compression Ratio Determination of JPEG2000 in Digitized Mammograms
Journal of Digital Imaging(2012)
摘要
The current study presents a quantitative approach towards visually lossless compression ratio (CR) threshold determination of JPEG2000 in digitized mammograms. This is achieved by identifying quantitative image quality metrics that reflect radiologists’ visual perception in distinguishing between original and wavelet-compressed mammographic regions of interest containing microcalcification clusters (MCs) and normal parenchyma, originating from 68 images from the Digital Database for Screening Mammography. Specifically, image quality of wavelet-compressed mammograms (CRs, 10:1, 25:1, 40:1, 70:1, 100:1) is evaluated quantitatively by means of eight image quality metrics of different computational principles and qualitatively by three radiologists employing a five-point rating scale. The accuracy of the objective metrics is investigated in terms of (1) their correlation ( r ) with qualitative assessment and (2) ROC analysis ( A z index), employing pooled radiologists’ rating scores as ground truth. The quantitative metrics mean square error, mean absolute error, peak signal-to-noise ratio, and structural similarity demonstrated strong correlation with pooled radiologists’ ratings ( r , 0.825, 0.823, −0.825, and −0.826, respectively) and the highest area under ROC curve ( A z , 0.922, 0.920, 0.922, and 0.922, respectively). For each quantitative metric, the highest accuracy values of corresponding ROC curves were used to define metric cut-off values. The metrics cut-off values were subsequently used to suggest a visually lossless CR threshold, estimated to be between 25:1 and 40:1 for the dataset analyzed. Results indicate the potential of the quantitative metrics approach in predicting visually lossless CRs in case of MCs in mammography.
更多查看译文
关键词
Image compression,Visually lossless,Compression ratio threshold,Image quality metrics,JPEG2000,Mammography,Microcalcification cluster
AI 理解论文
溯源树
样例
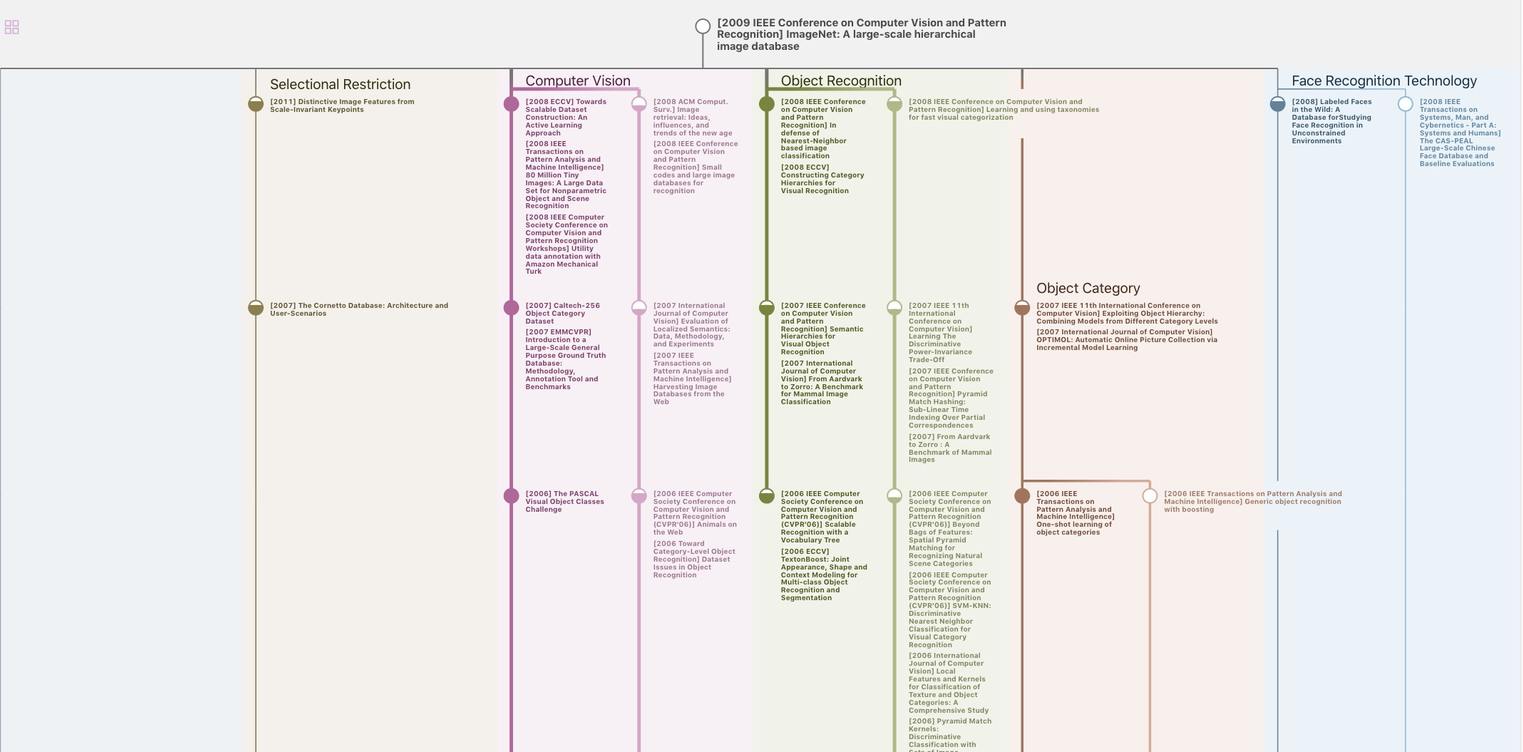
生成溯源树,研究论文发展脉络
Chat Paper
正在生成论文摘要