Efficient Sum-Based Hierarchical Smoothing Under ℓ_1-Norm
arxiv(2011)
摘要
We introduce a new regression problem which we call the Sum-Based
Hierarchical Smoothing problem. Given a directed acyclic graph and a
non-negative value, called target value, for each vertex in the graph, we wish
to find non-negative values for the vertices satisfying a certain constraint
while minimizing the distance of these assigned values and the target values in
the lp-norm. The constraint is that the value assigned to each vertex should be
no less than the sum of the values assigned to its children. We motivate this
problem with applications in information retrieval and web mining. While our
problem can be solved in polynomial time using linear programming, given the
input size in these applications such a solution might be too slow. We mainly
study the ℓ_1-norm case restricting the underlying graphs to rooted trees.
For this case we provide an efficient algorithm, running in O(n^2) time. While
the algorithm is purely combinatorial, its proof of correctness is an elegant
use of linear programming duality. We believe that our approach may be
applicable to similar problems, where comparable hierarchical constraints are
involved, e.g. considering the average of the values assigned to the children
of each vertex. While similar in flavor to other smoothing problems like
Isotonic Regression (see for example [Angelov et al. SODA'06]), our problem is
arguably richer and theoretically more challenging.
更多查看译文
AI 理解论文
溯源树
样例
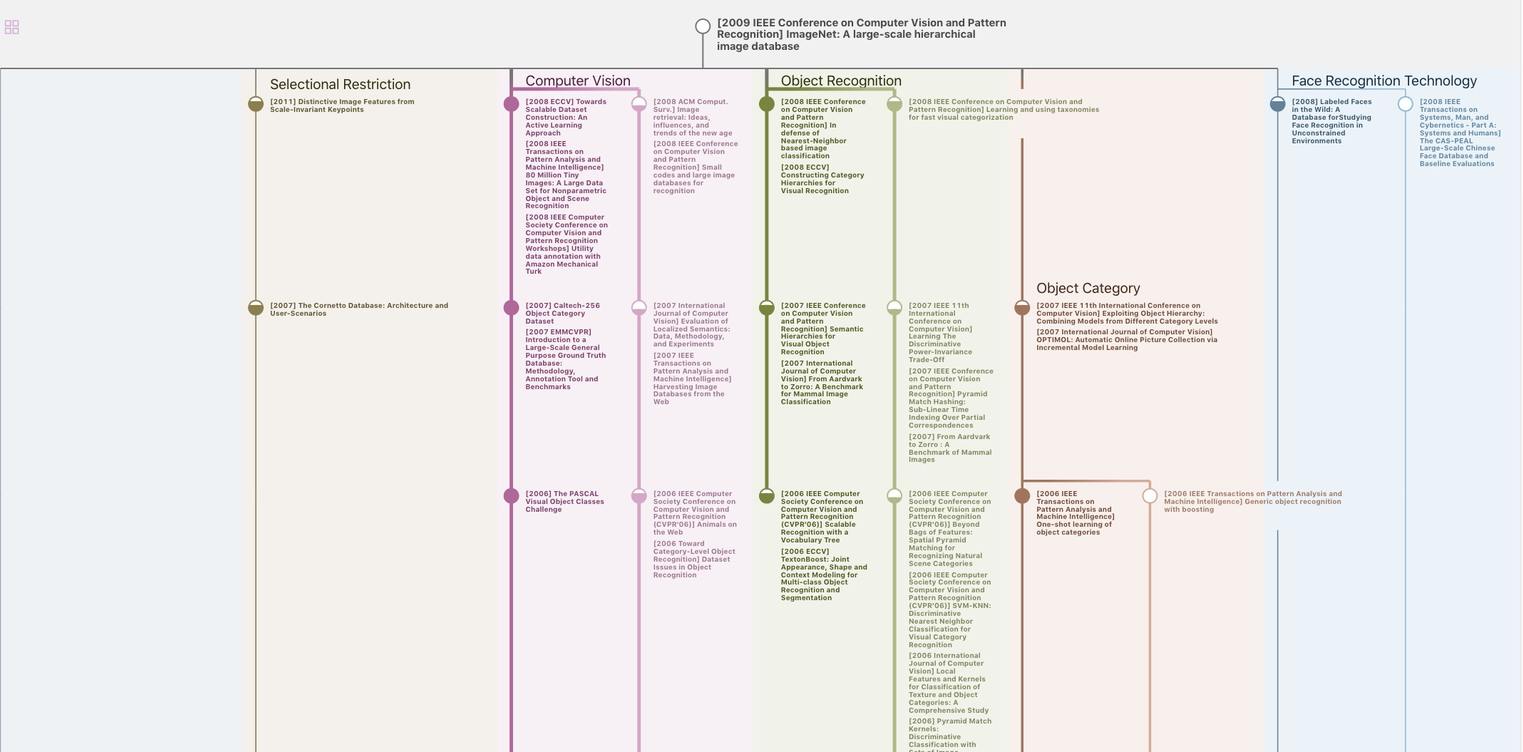
生成溯源树,研究论文发展脉络
Chat Paper
正在生成论文摘要