Object focused q-learning for autonomous agents
AAMAS(2013)
摘要
We present Object Focused Q-learning (OF-Q), a novel reinforcement learning algorithm that can offer exponential speed-ups over classic Q-learning on domains composed of independent objects. An OF-Q agent treats the state space as a collection of objects organized into different object classes. Our key contribution is a control policy that uses non-optimal Q-functions to estimate the risk of ignoring parts of the state space. We compare our algorithm to traditional Q-learning and previous arbitration algorithms in two domains, including a version of Space Invaders.
更多查看译文
关键词
different object class,traditional q-learning,autonomous agent,control policy,previous arbitration algorithm,classic q-learning,exponential speed-up,state space,space invaders,focused q-learning,of-q agent,reinforcement learning
AI 理解论文
溯源树
样例
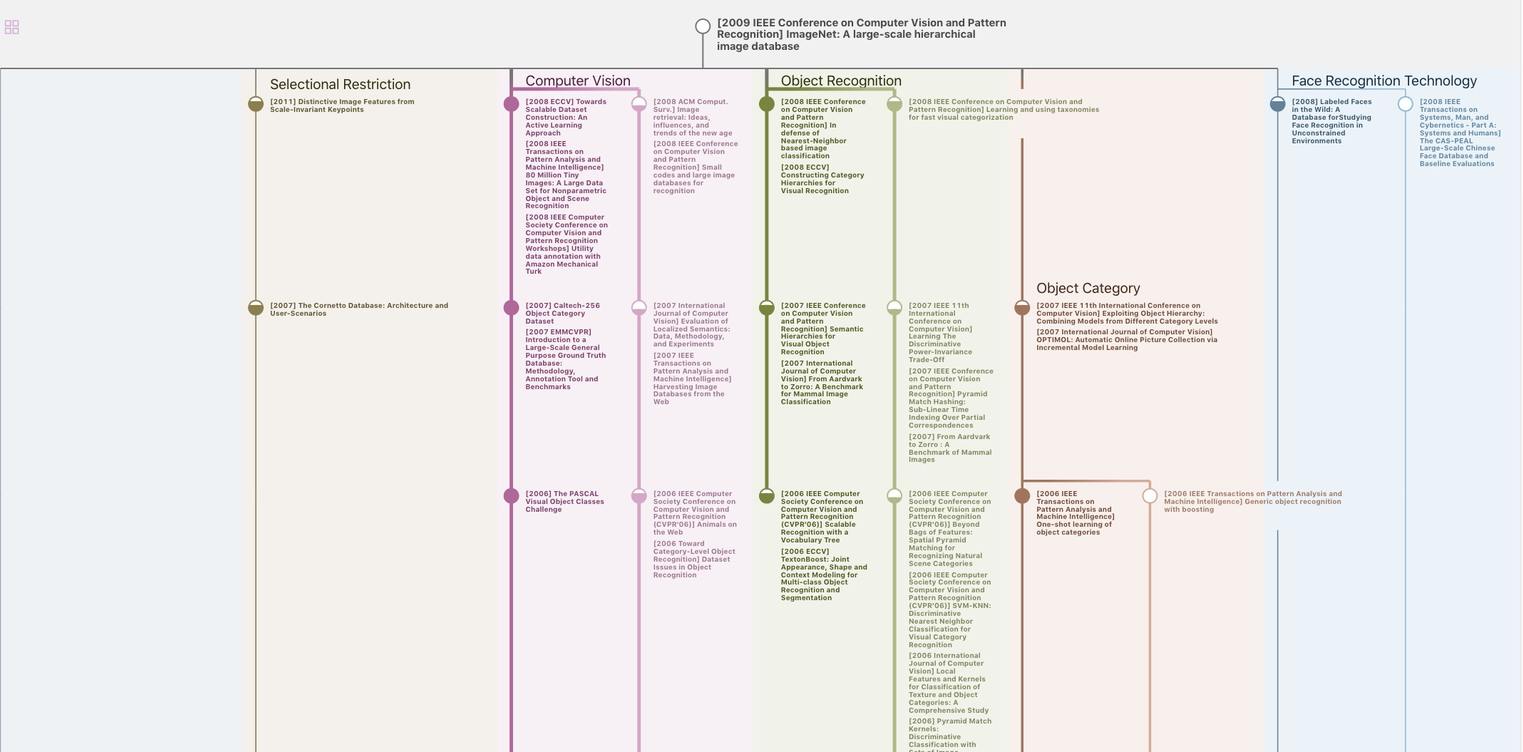
生成溯源树,研究论文发展脉络
Chat Paper
正在生成论文摘要