Monte-Carlo Tree Search in Production Management Problems
belgium-netherlands conference on artificial intelligence(2006)
摘要
Classical search algorithms rely on the existence of a suffic iently powerful evalua- tion function for non-terminal states. In many task domains, the development of such an evaluation function requires substantial effort and domain knowledge, or is not even possible. As an alternative in recent years, Monte-Carlo evaluation has been succesfully applied in such task domains. In this paper, we apply a search algorithm based on Monte-Carlo evaluation, Monte- Carlo Tree Search, in the task domain of production management problems. These can be defined as single-agent problems which consist of selecti ng a sequence of actions with side effects, leading to high quantities of one or more goal products. They are challenging and can be constructed with highly variable difficulty. Earlier research yielded an offline learning algorithm that leads to good solu tions, but requires a long time to run. We show that Monte-Carlo Tree Search leads to a solution in a shorter period of time than this algorithm, with improved solutions for large problems. Our findings can be generalized to other task domains.
更多查看译文
AI 理解论文
溯源树
样例
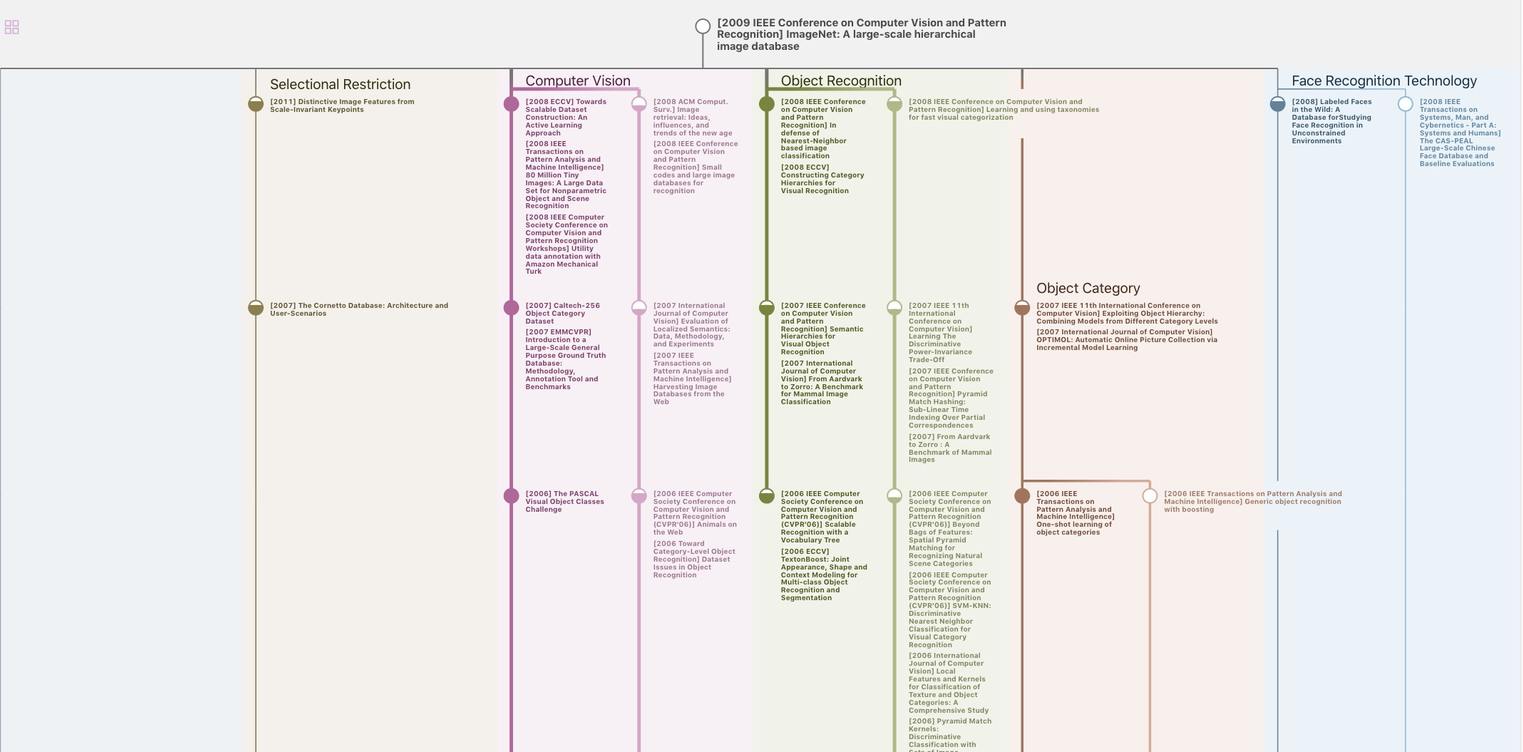
生成溯源树,研究论文发展脉络
Chat Paper
正在生成论文摘要