ATLAS: a probabilistic algorithm for high dimensional similarity search.
MOD(2011)
摘要
ABSTRACTGiven a set of high dimensional binary vectors and a similarity function (such as Jaccard and Cosine), we study the problem of finding all pairs of vectors whose similarity exceeds a given threshold. The solution to this problem is a key component in many applications with feature-rich objects, such as text, images, music, videos, or social networks. In particular, there are many important emerging applications that require the use of relatively low similarity thresholds. We propose ATLAS, a probabilistic similarity search algorithm that in expectation finds a 1 - δ fraction of all similar vector pairs. ATLAS uses truly random permutations both to filter candidate pairs of vectors and to estimate the similarity between vectors. At a 97.5% recall rate, ATLAS consistently outperforms all state-of-the-art approaches and achieves a speed-up of up to two orders of magnitude over both exact and approximate algorithms.
更多查看译文
AI 理解论文
溯源树
样例
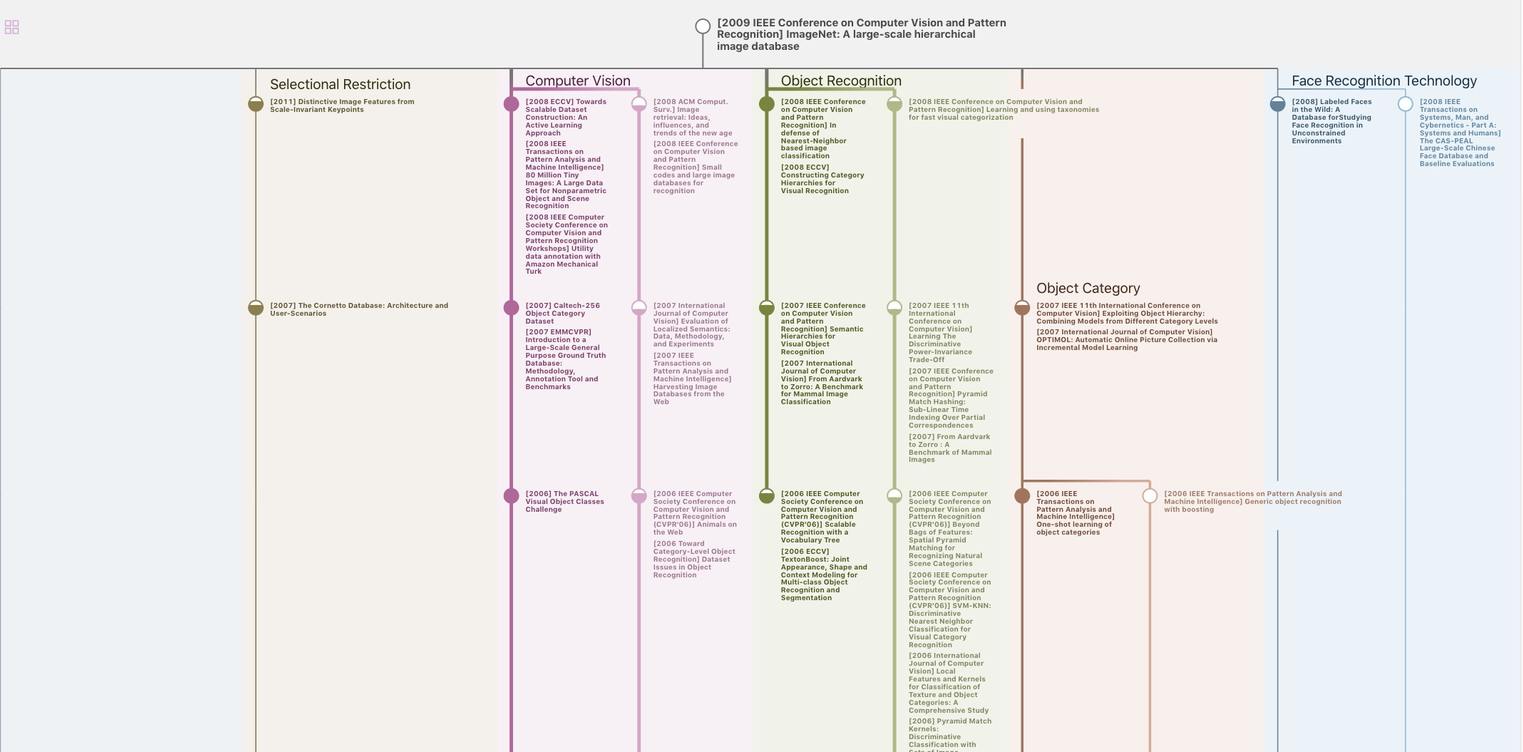
生成溯源树,研究论文发展脉络
Chat Paper
正在生成论文摘要