PROTOTYPE OPTIMIZATION BASED ON MINIMIZING CLASSIFICATION ORIENTED ERROR FUNCTION
JOURNAL OF THE CHINESE INSTITUTE OF ENGINEERS(2011)
摘要
A novel method of constructing optimized prototypes for nearest-neighbor classification is proposed. Based on an effective classification oriented error function containing class identification and class separation components, the corresponding updating rules for prototypes and feature weights are derived. By minimizing the error function, the optimized prototypes and feature weights from the nearest-neighbor classification point of view can be effectively constructed. The proposed method consists of several distinct process; Second, multiple prototypes not belonging to the true class of input sample x are updated when x is classified incorrectly; Third, it intrinsically assigns different learning factors to training samples, which enables a large amount of learning from constructive samples, and limited learning from outlier ones; Fourth, by adding a class separation component it avoids the degenerated situation where different prototypes coincide at the same feature position. Experiment results have shown that the proposed methods superior to LVQ2 and other method in previous work (Huang, 1995).
更多查看译文
关键词
neural networks,nearest neighbor classification,prototype optimization
AI 理解论文
溯源树
样例
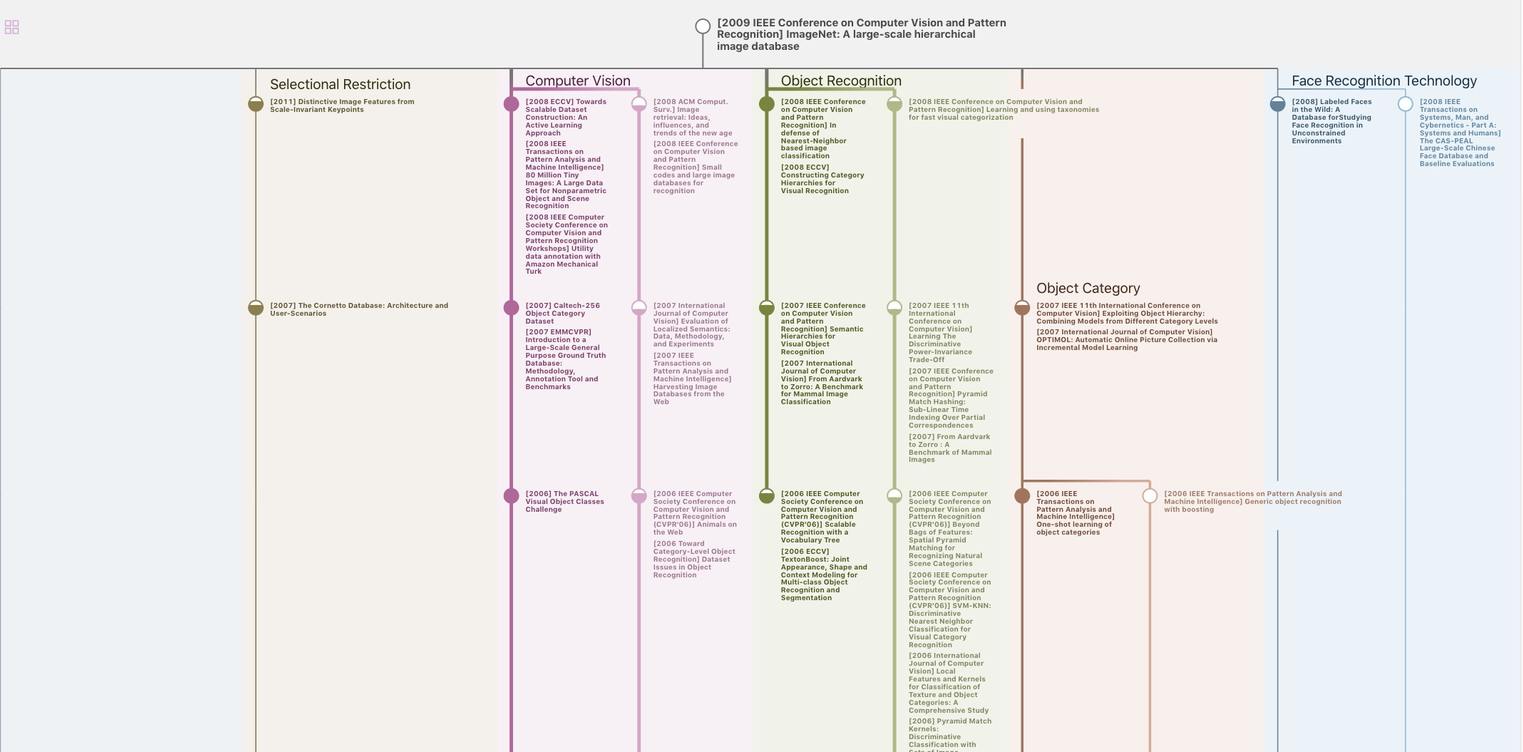
生成溯源树,研究论文发展脉络
Chat Paper
正在生成论文摘要