Clustering analysis using manifold kernel concept factorization.
Neurocomputing(2012)
摘要
Various exponential-growing documents and images have become omnipresent in past decades, and it is of vital importance to group them into clusters upon desired. Matrix factorization is exhibited to help yield encouraging clustering results in previous works, whereas the data manifold structure, which holds plentiful spatial model information, is not fully respected by most existing techniques. And kernel learning is advantageous for unfolding nonlinear structure. Therefore, in this paper we propose a novel clustering approach called Manifold Kernel Concept Factorization (MKCF) that incorporates the manifold kernel learning in concept factorization, which encodes the local geometrical structure in the kernel space. This method efficiently preserves the data semantic structure using graph Laplacian, and the nonlinear manifold learning in the warped RKHS potentially reflects the underlying local geometry of the data. Thus, the concepts consistent with the intrinsic manifold structure are well extracted, and this greatly benefits aggregating documents and images within the same concept into the same cluster. Extensive empirical studies demonstrate that MKCF owns the superiority of achieving the more satisfactory clustering performance as well as deriving the better-represented lower data space.
更多查看译文
关键词
Manifold kernel learning,Concept factorization,Graph Laplacian,Document clustering,Image clustering
AI 理解论文
溯源树
样例
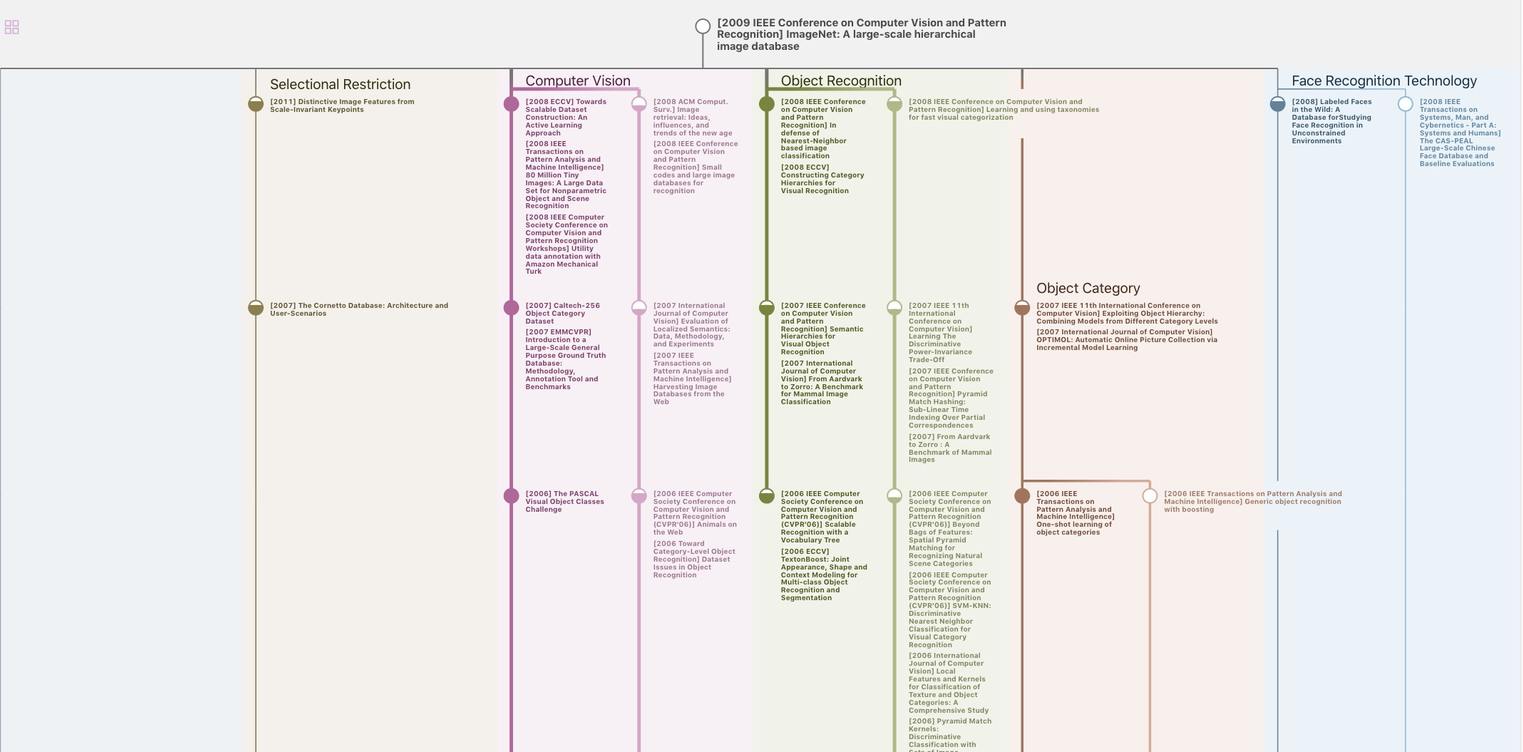
生成溯源树,研究论文发展脉络
Chat Paper
正在生成论文摘要