Improved prediction of trans-membrane spans in proteins using an Artificial Neural Network.
CIBCB'09: Proceedings of the 6th Annual IEEE conference on Computational Intelligence in Bioinformatics and Computational Biology(2009)
摘要
Tools for the identification of trans-membrane spans from the protein sequence are widely used in the experimental community. Computational structural biology seeks to increase the prediction accuracy of such methods since they represent a first step towards membrane protein tertiary structure prediction from the amino acid sequence. We introduce a predictor that is able to identify trans-membrane spans from the sequence of a protein. The novelty of the approach presented here is the simultaneous prediction of trans-membrane spanning α-helices and β-strands within a single tool. An artificial neural network was trained on databases of 102 membrane proteins and 3499 soluble proteins. Prediction accuracies of up to 92% for soluble residues, 75% for residues in the interface, and 73% for TM residues are achieved. On average the algorithm predicts 79% of the residues correctly which is a substantial improvement from a previously published implementation which achieved 57% accuracy (Koehler et al., Proteins: Structure, Function, and Bioinformatics, 2008). The algorithm was applied to four membrane proteins to illustrate the applicability to both α-helical bundles and β-barrels.
更多查看译文
关键词
biology computing,biomembranes,molecular biophysics,neural nets,proteins,alpha-helical bundles,amino acid sequence,artificial neural network,beta-barrels,computational structural biology,protein sequence,transmembrane span,
AI 理解论文
溯源树
样例
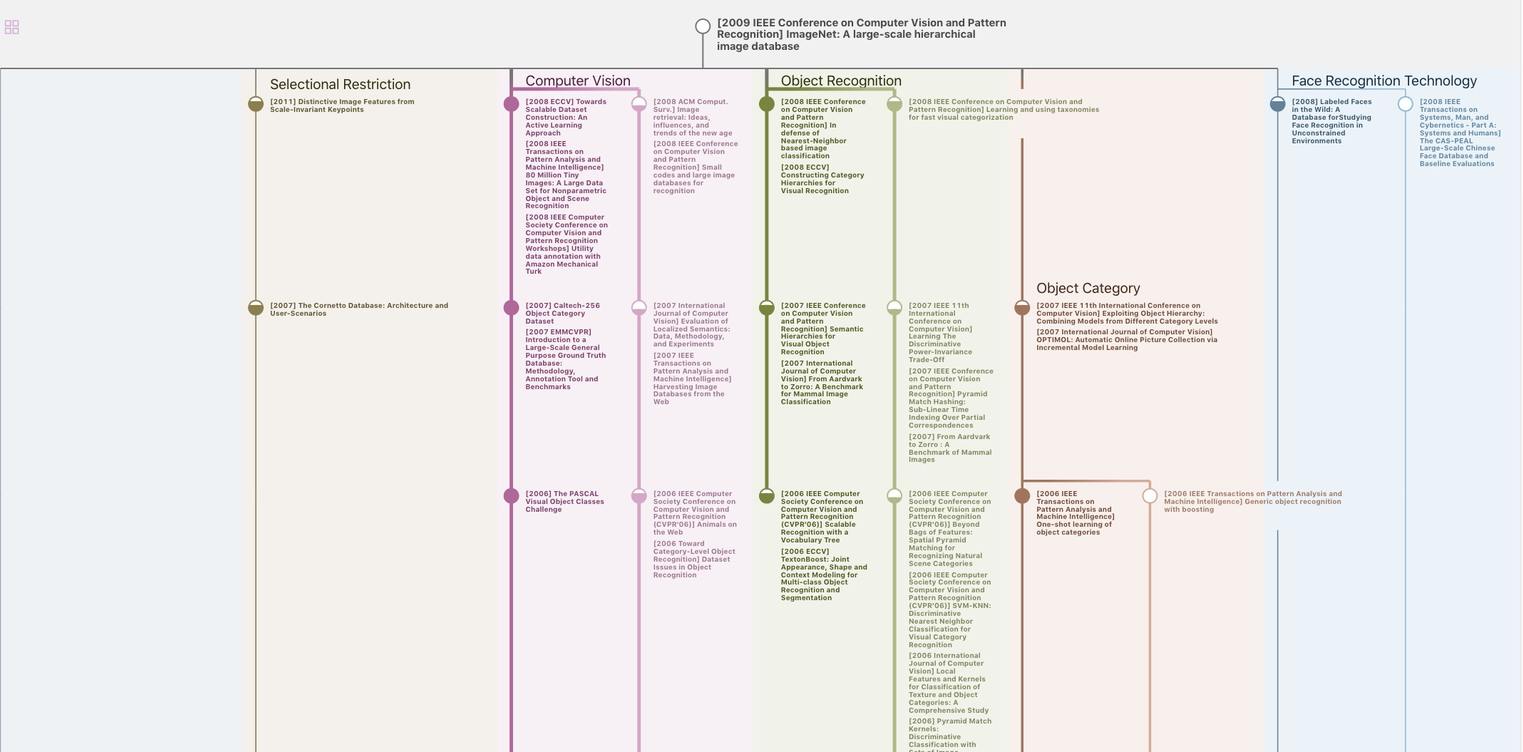
生成溯源树,研究论文发展脉络
Chat Paper
正在生成论文摘要