TopRec: domain-specific recommendation through community topic mining in social network
WWW(2013)
摘要
Traditionally, Collaborative Filtering assumes that similar users have similar responses to similar items. However, human activities exhibit heterogenous features across multiple domains such that users own similar tastes in one domain may behave quite differently in other domains. Moreover, highly sparse data presents crucial challenge in preference prediction. Intuitively, if users' interested domains are captured first, the recommender system is more likely to provide the enjoyed items while filter out those uninterested ones. Therefore, it is necessary to learn preference profiles from the correlated domains instead of the entire user-item matrix. In this paper, we propose a unified framework, TopRec, which detects topical communities to construct interpretable domains for domain-specific collaborative filtering. In order to mine communities as well as the corresponding topics, a semi-supervised probabilistic topic model is utilized by integrating user guidance with social network. Experimental results on real-world data from Epinions and Ciao demonstrate the effectiveness of the proposed framework.
更多查看译文
关键词
preference prediction,similar item,unified framework,similar taste,similar user,social network,similar response,real-world data,preference profile,proposed framework,sparse data,domain-specific recommendation,community topic mining,collaborative filtering,recommender systems
AI 理解论文
溯源树
样例
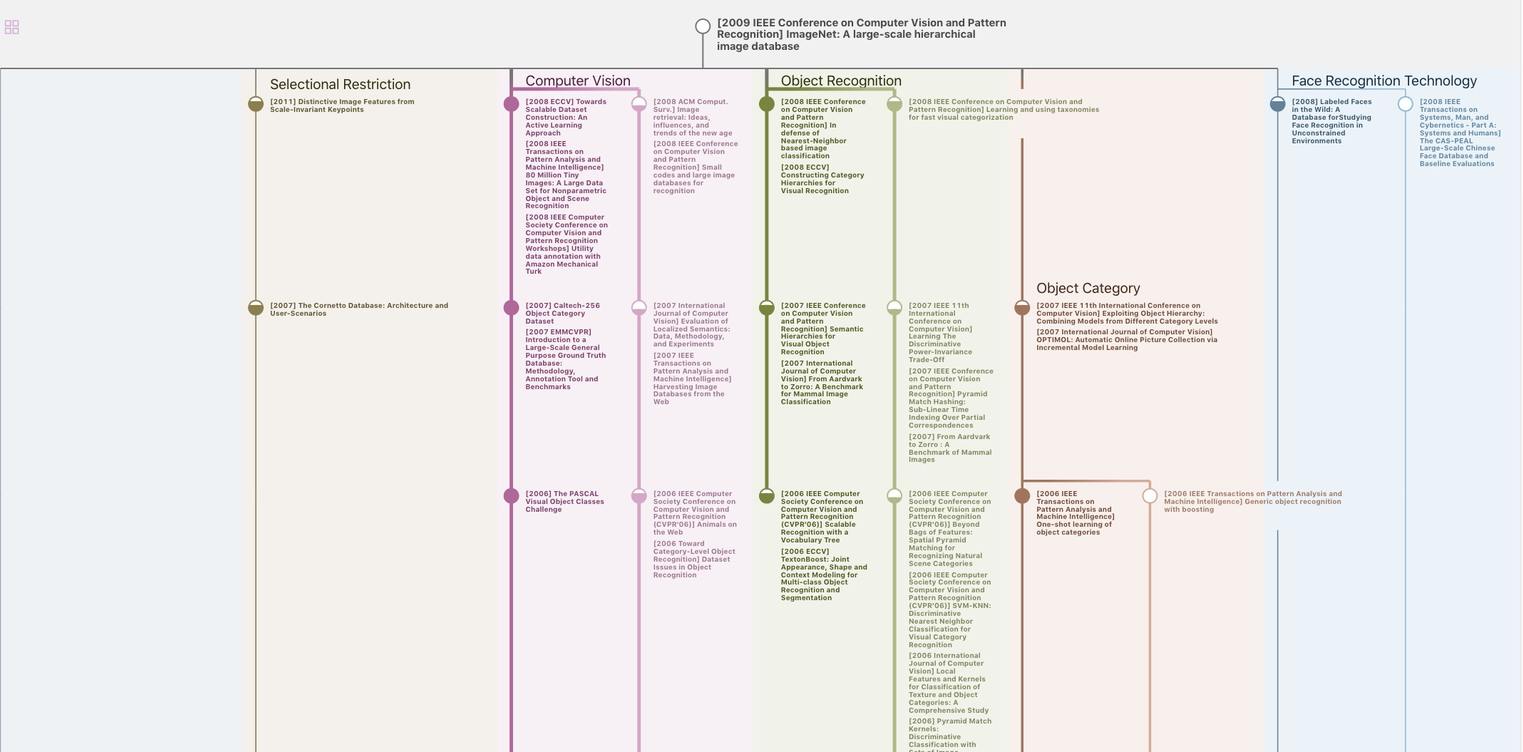
生成溯源树,研究论文发展脉络
Chat Paper
正在生成论文摘要