Parsimonious Support Vector Regression using Orthogonal Forward Selection with the Generalized Kernel Model
msra(2004)
摘要
Sparse regression modeling is addressed using a generalized kernel model in which kernel regressor has its individually tuned position (center) vector and diagonal covariance matrix. An orthogonal least squares forward selection procedure is employed to append regressors one by one. After the determination of the model structure, namely the selection certain number of regressors, the model weight parameters are calculated from the Lagrange dual problem of the regression problem with the regularized linear − ε insensitive loss function. Different from the support vector regression, this stage of the procedure involves neither reproducing kernel Hilbert nor Mercer decomposition concepts and thus the difficulties associated with selecting a mapping from the input space to the feature space, needed in the support vector machine methods, can be avoided. Moreover, as the regressors used here are not restricted to be positioned at training input points and each regressor has its own diagonal covariance matrix, sparser representation can be obtained. Experimental results involving one toy example and two data sets demonstrate the effectiveness of the proposed regression modeling approach.
更多查看译文
关键词
support vector machine,orthogonal least squares forward selection,regression,generalized kernel model,sparse modeling
AI 理解论文
溯源树
样例
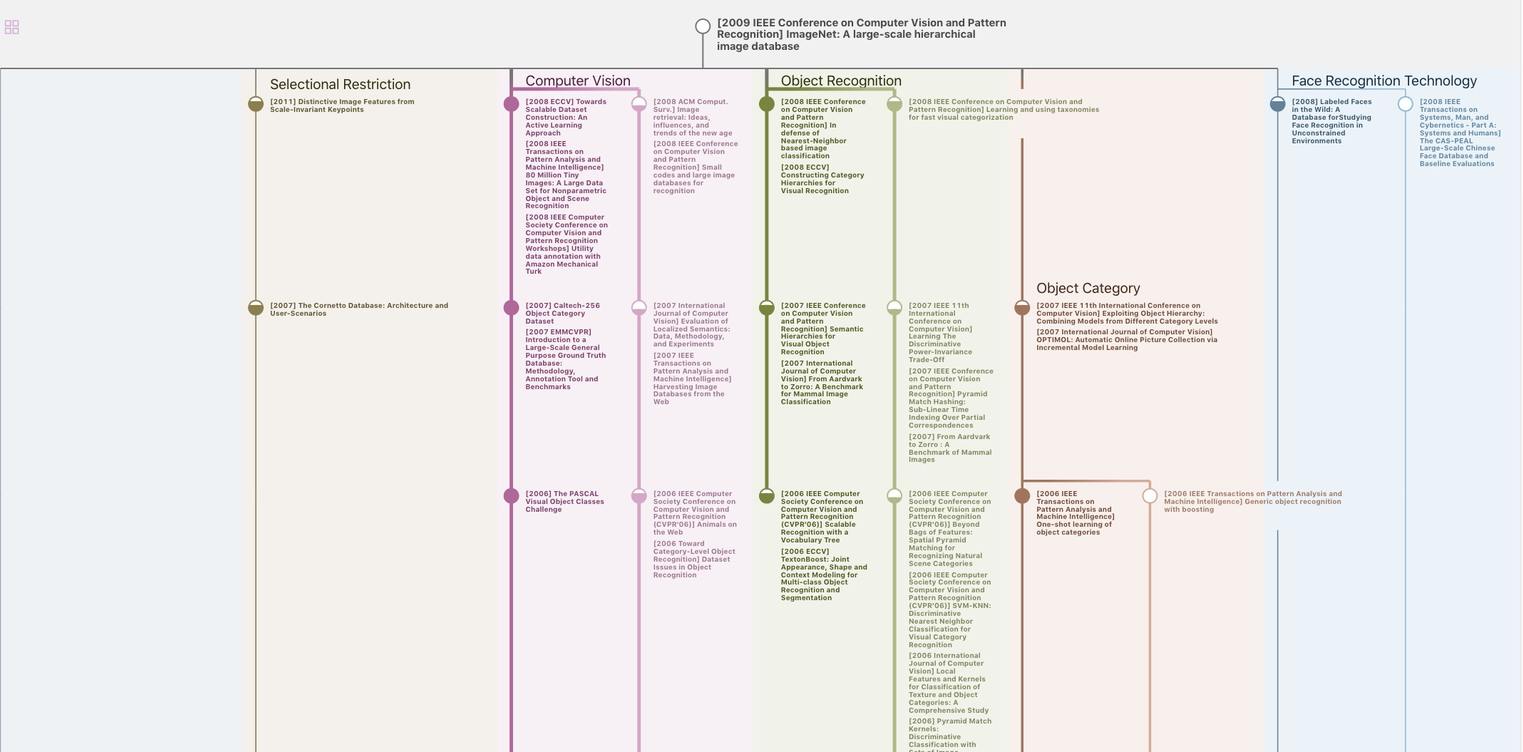
生成溯源树,研究论文发展脉络
Chat Paper
正在生成论文摘要