A new model for measuring the accuracies of majority voting ensembles
IJCNN(2012)
摘要
Good ensemble methods require accurate and diverse individual classifiers, but the relationship between the diversity of individual classifiers and the accuracy of an ensemble method is not clear. In this paper, we propose a novel model called COB (core, outlier, and boundary) to quantitatively measure the accuracies of majority voting ensembles for binary classification. In this model, we first divide data items into three subsets, core, outlier, and boundary, based on the prediction correctness of these items from individual classifiers in an ensemble method. Then we measure the accuracy of the ensemble method for each subset and combine the results together. We tested the performance of the COB model on 32 datasets from the UCI repository. The experiments use three different ensemble methods (bagging, random forests, and a randomized ensemble), two different numbers of individual classifiers (7 and 51), and three different individual machine learning algorithms (decision trees, k-nearest neighbors, and support vector machines). All 24 experiments showed less than 5% average absolute errors for 32 datasets between the accuracies by the COB model and the actual accuracies of ensembles. Also the experiments showed that the COB model performed significantly better than the binomial model. The COB model suggests that to achieve a high accuracy for an ensemble method, weak individual classifiers should be partly diverse instead of fully diverse, that is, be diverse on correctly predicted items but in agreement on some incorrectly predicted items.
更多查看译文
关键词
binary classification,majority voting ensembles,k-nearest neighbors,prediction correctness,ensemble methods,learning (artificial intelligence),accuracy,pattern classification,random forests,measurement,diverse individual classifiers,majority voting,bagging,machine learning,randomized ensemble,decision trees,support vector machines,predictive models,mathematical model,k nearest neighbors,learning artificial intelligence
AI 理解论文
溯源树
样例
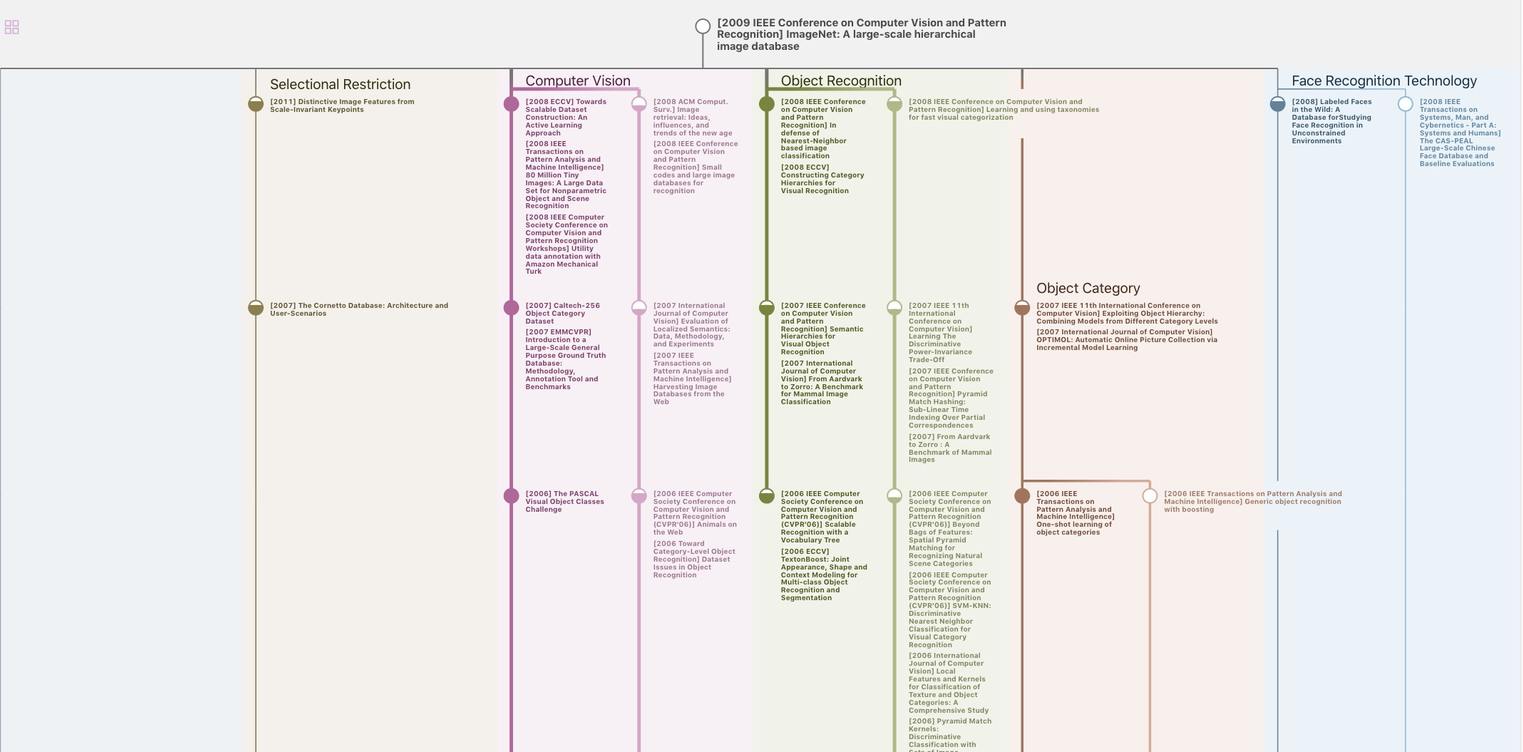
生成溯源树,研究论文发展脉络
Chat Paper
正在生成论文摘要