Temporal Relations In Videos For Unsupervised Activity Analysis
PROCEEDINGS OF THE BRITISH MACHINE VISION CONFERENCE 2011(2011)
摘要
Temporal consistency is a strong cue in continuous data streams and especially in videos. We exploit this concept and encode temporal relations between consecutive frames using discriminative slow feature analysis. Activities are automatically segmented and represented in a hierarchical coarse to fine structure. Simultaneously, they are modeled in a generative manner, in order to analyze unseen data. This analysis supports the detection of previously learned activities and of abnormal, novel patterns. Our technique is purely data-driven and feature-independent. Experiments validate the approach in several contexts, such as traffic flow analysis and the monitoring of human behavior. The results are competitive with the state-of-the-art in all cases.
更多查看译文
AI 理解论文
溯源树
样例
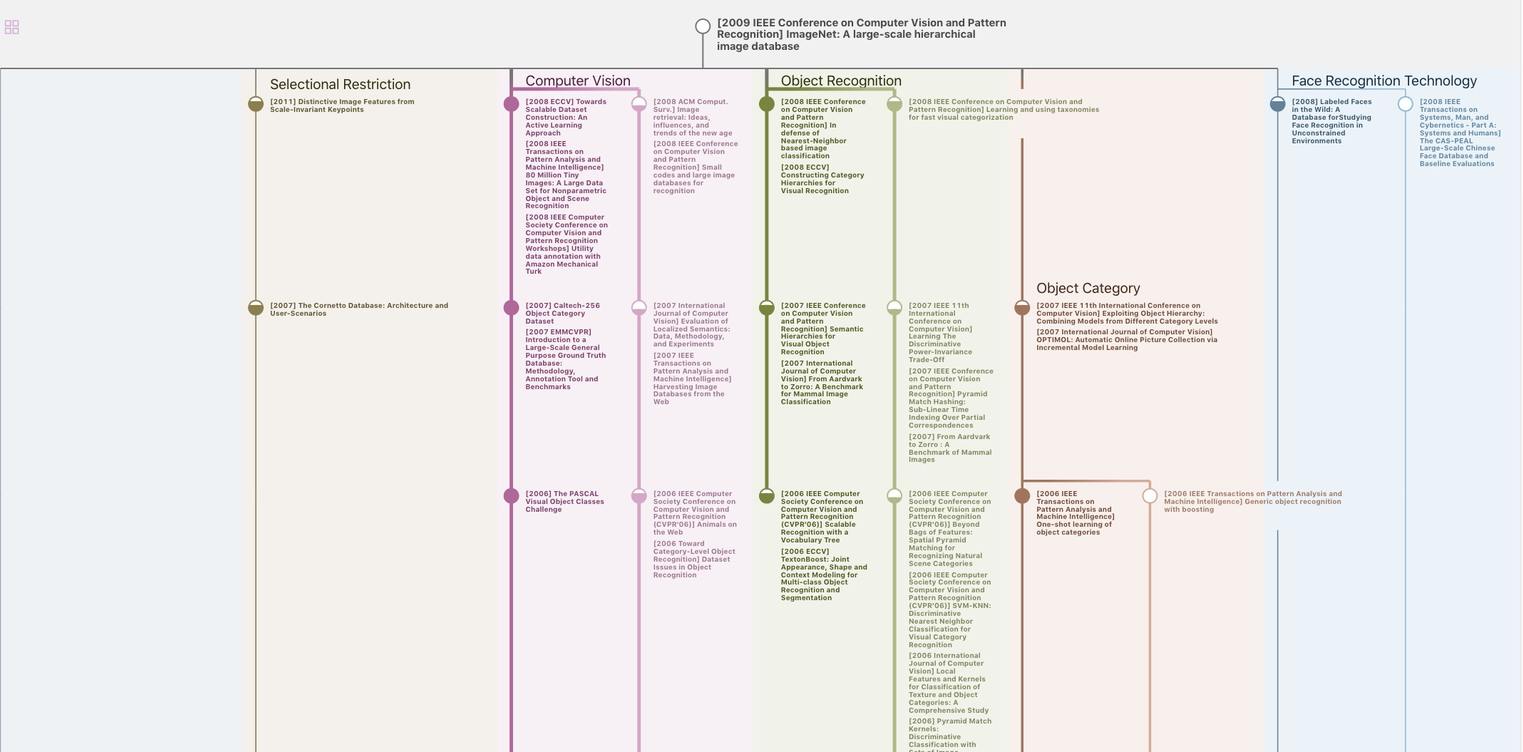
生成溯源树,研究论文发展脉络
Chat Paper
正在生成论文摘要