Modeling General and Specific Aspects of Documents with a Probabilistic Topic Model
NIPS(2006)
摘要
Techniques such as probabilistic topic models and latent-semantic indexing have been shown to be broadly useful at automatically extracting the topical or seman- tic content of documents, or more generally for dimension-reduction of sparse count data. These types of models and algorithms can be viewed as generating an abstraction from the words in a document to a lower-dimensional latent variable representation that captures what the document is generally about beyond the spe- cific words it contains. In this paper we propose a new probabi listic model that tempers this approach by representing each document as a combination of (a) a background distribution over common words, (b) a mixture distribution over gen- eral topics, and (c) a distribution over words that are treat ed as being specific to that document. We illustrate how this model can be used for information retrieval by matching documents both at a general topic level and at a specific word level, providing an advantage over techniques that only match documents at a general level (such as topic models or latent-sematic indexing) or t hat only match docu- ments at the specific word level (such as TF-IDF).
更多查看译文
关键词
information retrieval,indexation,latent semantic indexing,dimension reduction,count data,latent variable,mixture distribution
AI 理解论文
溯源树
样例
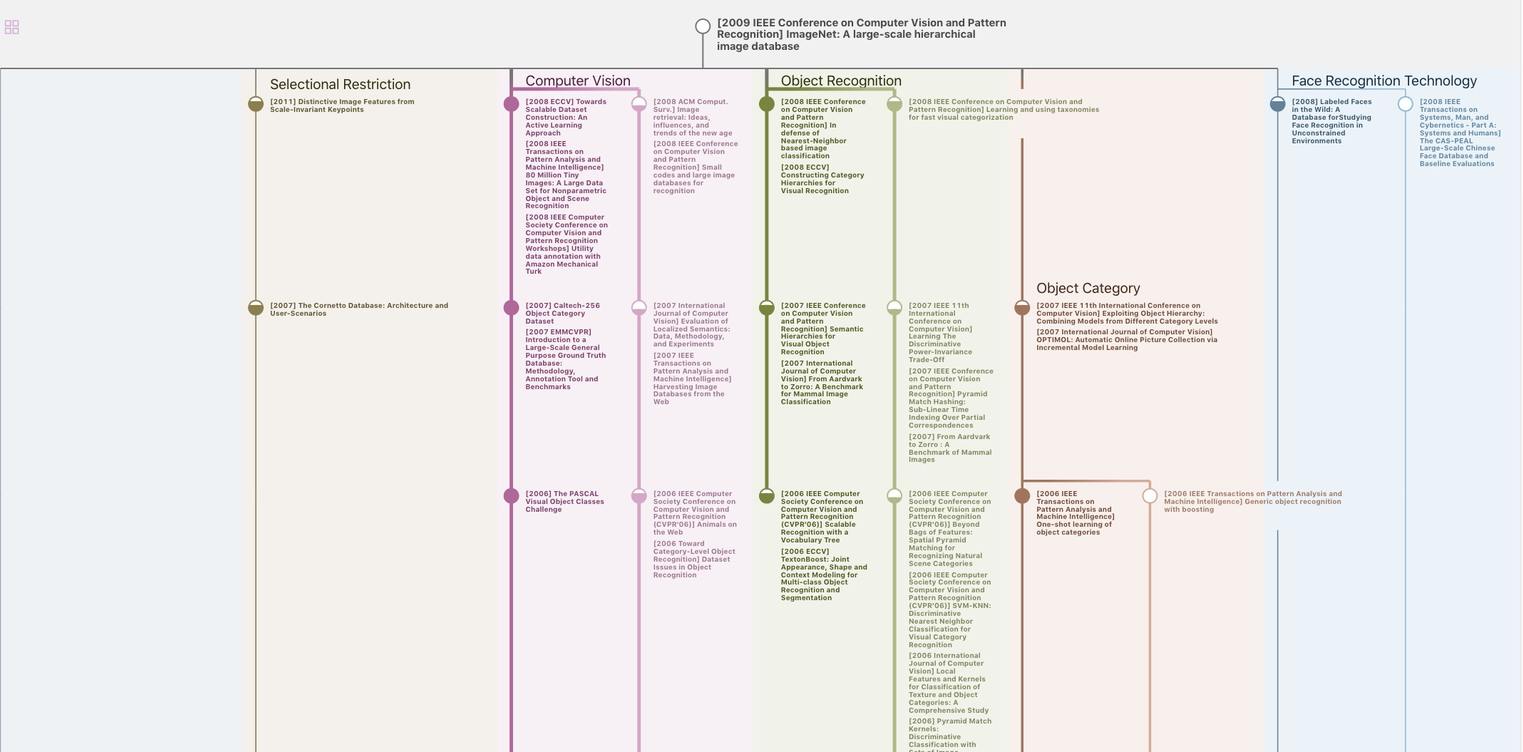
生成溯源树,研究论文发展脉络
Chat Paper
正在生成论文摘要