Knowledge-Based Lung Nodule Detection from Helical CT
Bildverarbeitung für die Medizin(1999)
摘要
Lung nodule detection from CT images has been proven a difficult task even for experienced radiologists. The crucial clinical information concerning the total number of nodules and their size can only be acquired by a reliable detection and out-coming analysis. Apart from detecting a suspicious lesion, its even more difficult to differentiate between true nodules and blood vessels. Recent advantages in CT imagery technique, like helical CT, allows acquisition of continuous 3D data of the lung without respiratory artifacts. Thus, lung structures can be 3D-reconstructed more precisely which allows a shape based separation between cylindrical blood vessels and spherical nodules. Based on helical CT 3D images we developed an automated detection method to find lung nodules by combining shape information and knowledge from lung structures and nodules out of the UCSF PACS environment. First we segment the lung reducing the search space and avoiding false detection outside the lung. The following inspection for suspicious lesions is done slice by slice for smaller nodules, inhabiting only one slice, and by using the whole 3D volume for larger nodules with the gradient Hough transformation for circles and spheres. Finally the detected suspicious lesions are segmented and examined by verifying knowledge about the lung structures. The resulting nodules are stored in a patient database for further analysis of biometrics and cancer tissue textures.
更多查看译文
关键词
knowledge base
AI 理解论文
溯源树
样例
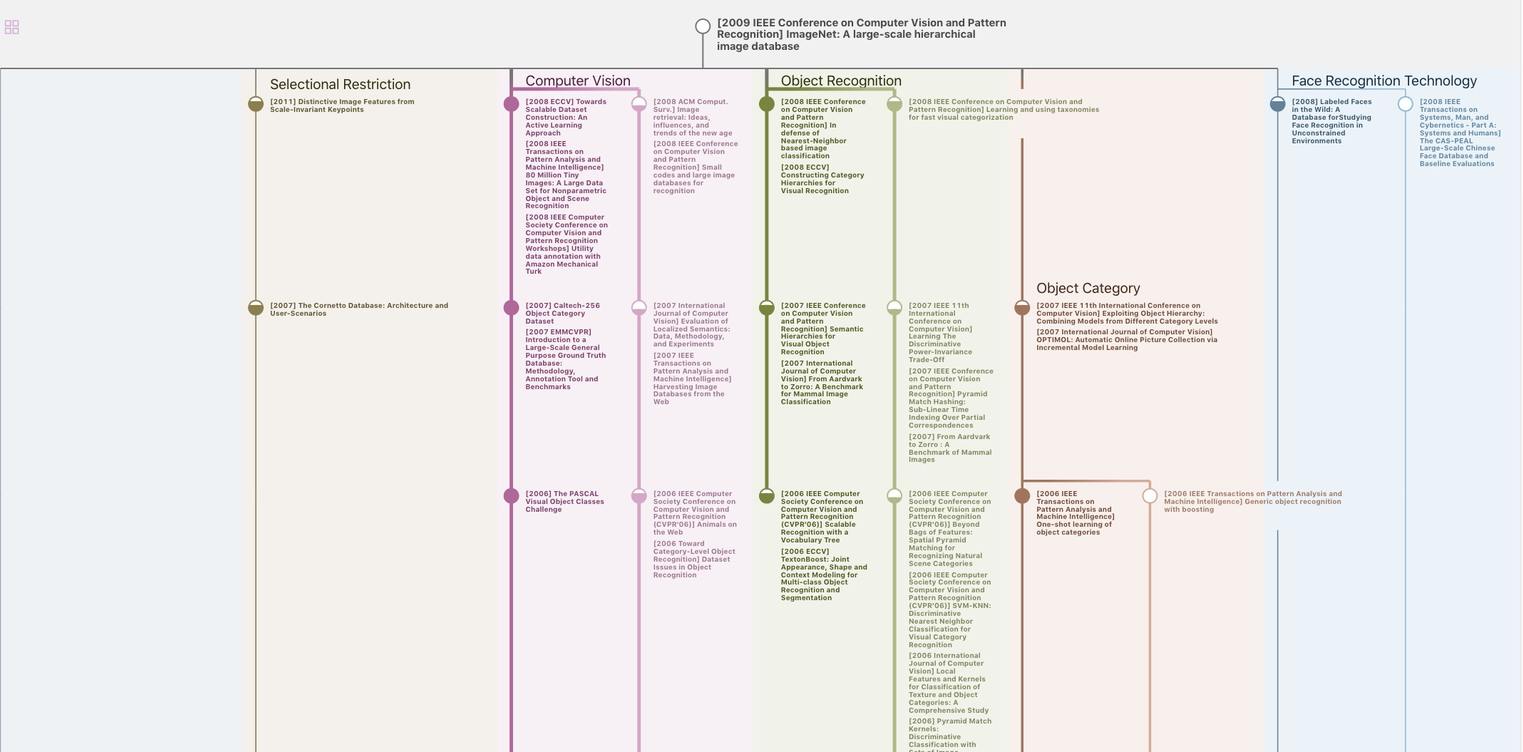
生成溯源树,研究论文发展脉络
Chat Paper
正在生成论文摘要