Learning tactic-based motion models with fast particle smoothing
ICRA(2008)
摘要
Learning parameters of a motion model is an important challenge for autonomous robots. We address the particular instance of parameter learning when tracking motions with a switching state-space model. We present a general algorithm for dealing simultaneously with both unknown fixed model parameters and state variables. Using an Expectation-Maximization approach, we apply a tactic-based multi-model particle filter to estimate the state variables in the E-step, and use particle smoothing to update the parameters in the M-step. We test our algorithm both in simulation and in a team robot soccer environment, as a substrate for applying the learned models to object tracking in a team. One of the soccer robots learns the actuation model of its teammate. The experimental results show that the particle smoothing efficiency is substantially increased and the tracking performance is significantly improved using the learned teammate actuation model.
更多查看译文
关键词
particle filtering (numerical methods),expectation-maximisation algorithm,state-space methods,expectation-maximization approach,smoothing methods,learning (artificial intelligence),switching state-space model,mobile robots,multi-robot systems,autonomous robot,tactic-based motion model parameter learning,sport,particle smoothing,object tracking,learned teammate actuation model,object detection,tactic-based multimodel particle filter,team robot soccer environment,robot vision,robot learning,state space model,tracking,expectation maximization,generic algorithm,robot kinematics,particle filters,particle filter,computer science,learning artificial intelligence,bayesian methods
AI 理解论文
溯源树
样例
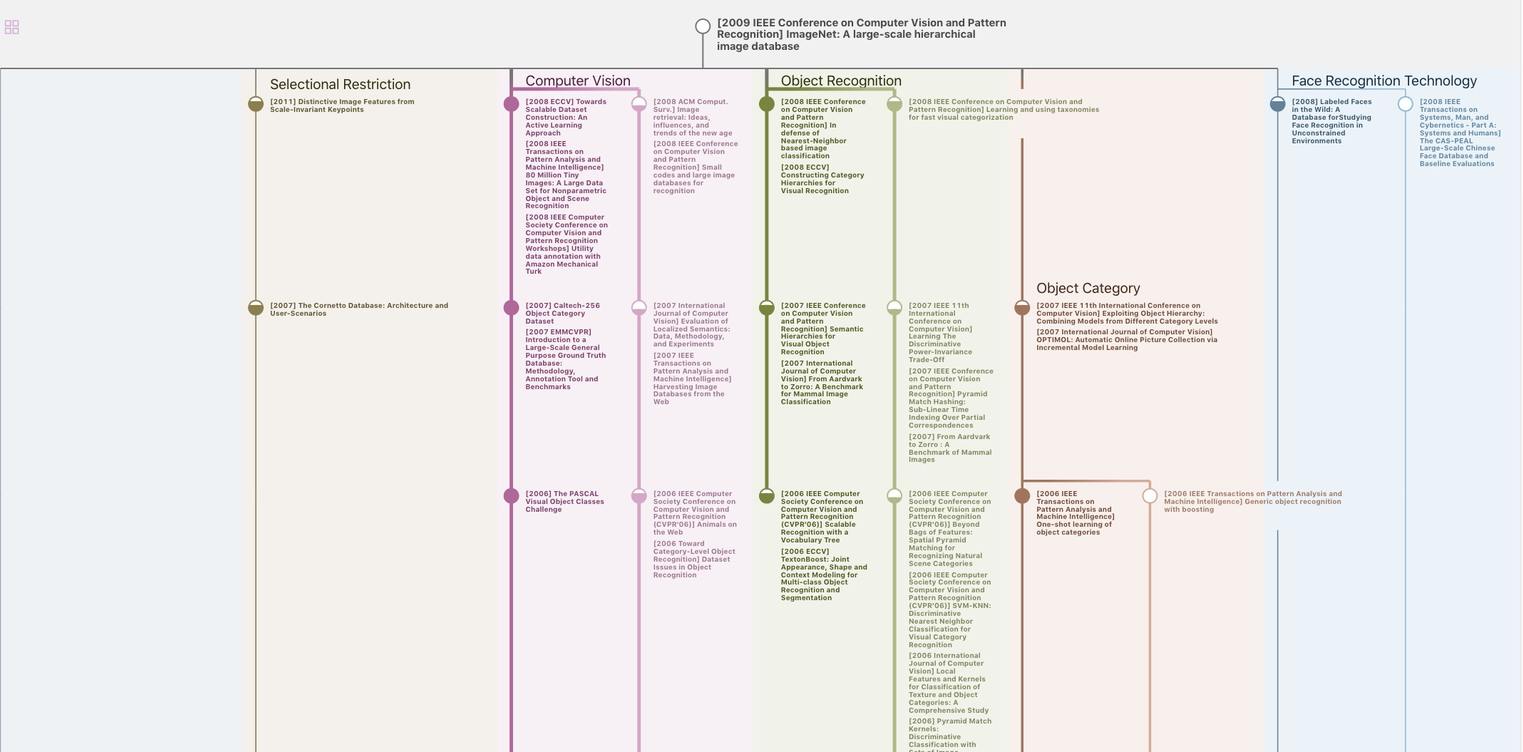
生成溯源树,研究论文发展脉络
Chat Paper
正在生成论文摘要