Generalized Domains for Empirical Evaluations in Reinforcement Learning
msra(2009)
摘要
Many empirical results in reinforcement learning are based on a very small set of envi- ronments. These results often represent the best algorithm parameters that were found after an ad-hoc tuning or tting process. We argue that presenting tuned scores from a small set of environments leads to method overtting, wherein results may not gener- alize to similar environments. To address this problem, we advocate empirical evalu- ations using generalized domains: parame- terized problem generators that explicitly en- code variations in the environment to which the learner should be robust. We argue that evaluating across a set of these generated problems oers a more meaningful evaluation of reinforcement learning algorithms.
更多查看译文
关键词
reinforcement learning
AI 理解论文
溯源树
样例
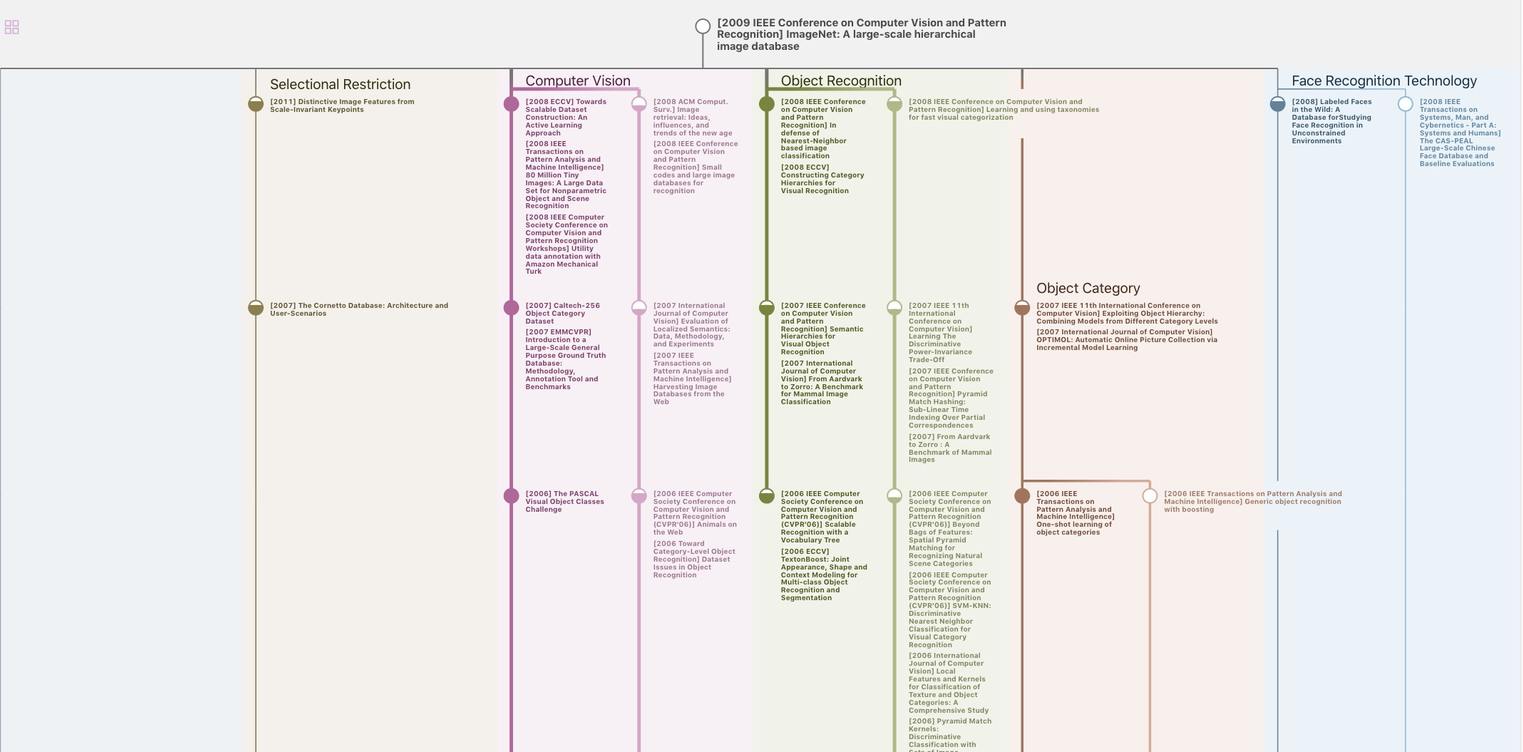
生成溯源树,研究论文发展脉络
Chat Paper
正在生成论文摘要