Statistical Uncertainty Of Eddy Flux-Based Estimates Of Gross Ecosystem Carbon Exchange At Howland Forest, Maine
JOURNAL OF GEOPHYSICAL RESEARCH-ATMOSPHERES(2006)
摘要
[1] We present an uncertainty analysis of gross ecosystem carbon exchange ( GEE) estimates derived from 7 years of continuous eddy covariance measurements of forest-atmosphere CO2 fluxes at Howland Forest, Maine, USA. These data, which have high temporal resolution, can be used to validate process modeling analyses, remote sensing assessments, and field surveys. However, separation of tower-based net ecosystem exchange ( NEE) into its components ( respiration losses and photosynthetic uptake) requires at least one application of a model, which is usually a regression model fitted to nighttime data and extrapolated for all daytime intervals. In addition, the existence of a significant amount of missing data in eddy flux time series requires a model for daytime NEE as well. Statistical approaches for analytically specifying prediction intervals associated with a regression require, among other things, constant variance of the data, normally distributed residuals, and linearizable regression models. Because the NEE data do not conform to these criteria, we used a Monte Carlo approach (bootstrapping) to quantify the statistical uncertainty of GEE estimates and present this uncertainty in the form of 90% prediction limits. We explore two examples of regression models for modeling respiration and daytime NEE: ( 1) a simple, physiologically based model from the literature and ( 2) a nonlinear regression model based on an artificial neural network. We find that uncertainty at the half-hourly timescale is generally on the order of the observations themselves (i.e., similar to 100%) but is much less at annual timescales ( similar to 10%). On the other hand, this small absolute uncertainty is commensurate with the interannual variability in estimated GEE. The largest uncertainty is associated with choice of model type, which raises basic questions about the relative roles of models and data.
更多查看译文
关键词
eddy flux,gee,uncertainty,eddy covariance,prediction interval,biogeochemical cycle,monte carlo,missing data,remote sensing,artificial neural network,carbon cycle,normal distribution,uncertainty analysis,regression model,process model,temporal resolution,time series,global change,nonlinear regression
AI 理解论文
溯源树
样例
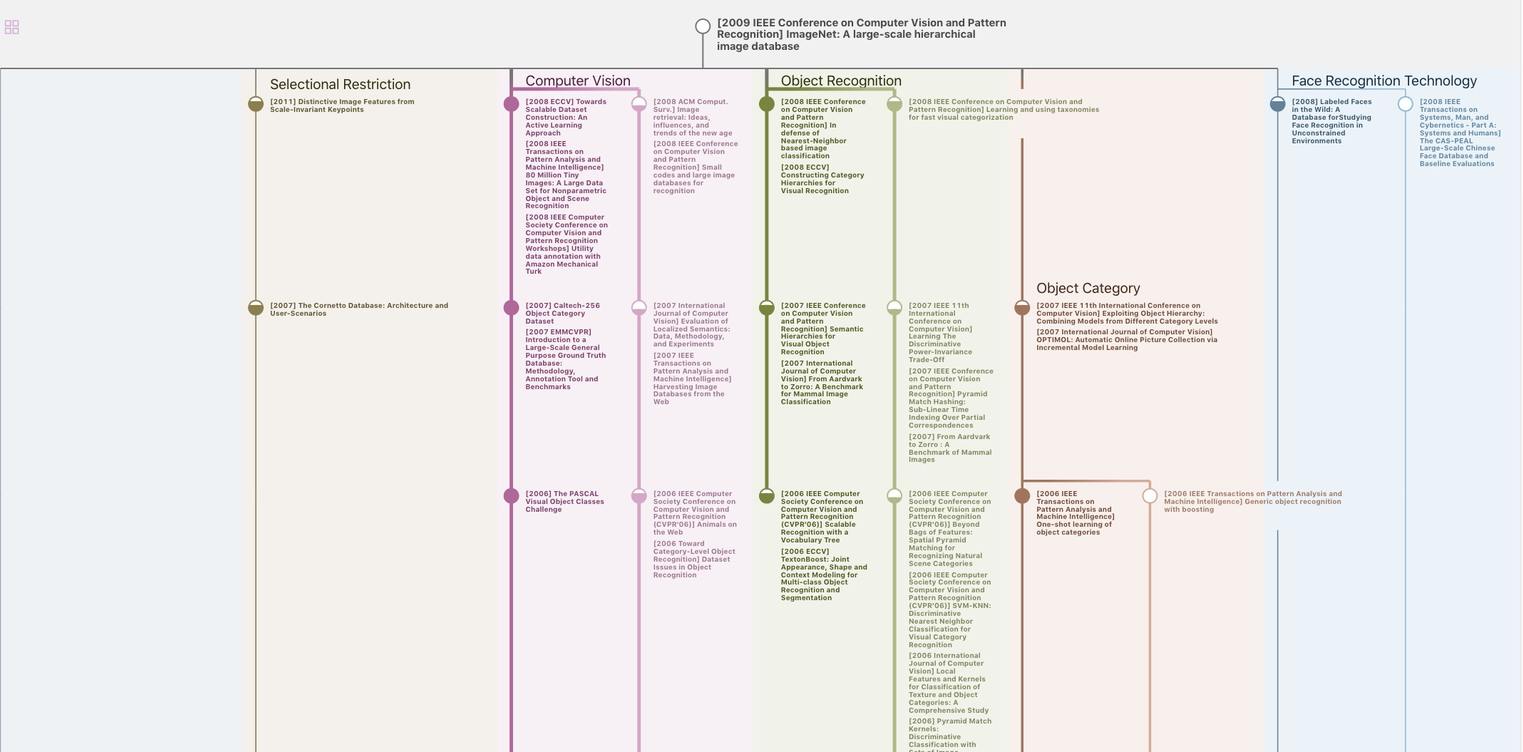
生成溯源树,研究论文发展脉络
Chat Paper
正在生成论文摘要