Prediction with Missing Data via Bayesian Additive Regression Trees.
CANADIAN JOURNAL OF STATISTICS-REVUE CANADIENNE DE STATISTIQUE(2015)
摘要
We present a method for incorporating missing data into general prediction problems which use nonparametric statistical learning. We focus on a tree-based method, Bayesian Additive Regression Trees (BART), enhanced with Missingness Incorporated in Attributes, a recently proposed approach for incorporating missingness into decision trees. This procedure extends the native partitioning mechanisms found in tree-based models and does not require imputation. Simulations on generated models and real data indicate that our procedure offers promise for both selection model and pattern-mixture frameworks as measured by out-of-sample predictive accuracy. We also illustrate BART's abilities to incorporate missingness into uncertainty intervals. Our implementation is readily available in the R package bartMachine. The Canadian Journal of Statistics 43: 224-239; 2015 (c) 2015 Statistical Society of Canada
更多查看译文
关键词
Bayesian,BART,Missing data,statistical learning,tree-based learning
AI 理解论文
溯源树
样例
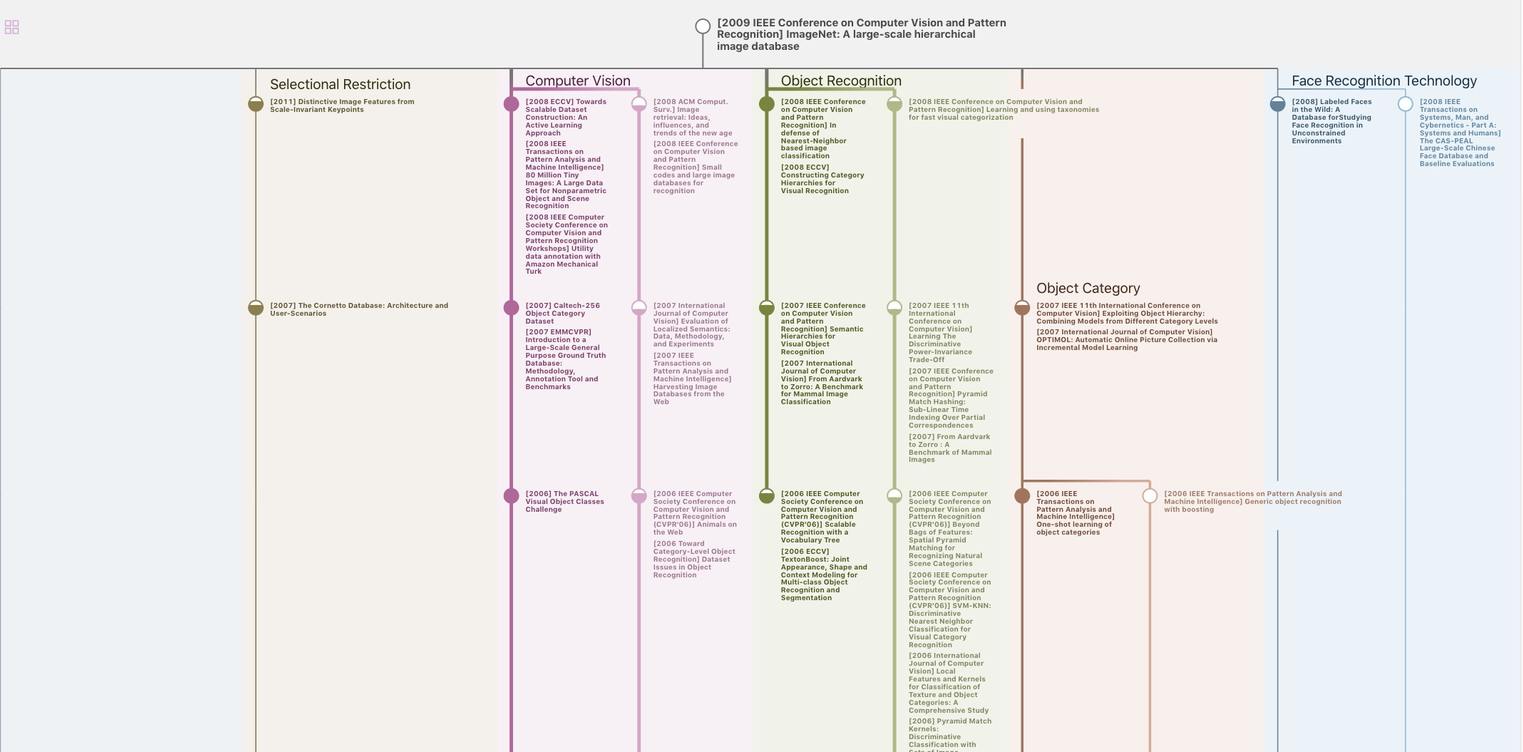
生成溯源树,研究论文发展脉络
Chat Paper
正在生成论文摘要