Learning control Lyapunov function to ensure stability of dynamical system-based robot reaching motions.
Robotics and Autonomous Systems(2014)
摘要
We consider an imitation learning approach to model robot point-to-point (also known as discrete or reaching) movements with a set of autonomous Dynamical Systems (DS). Each DS model codes a behavior (such as reaching for a cup and swinging a golf club) at the kinematic level. An estimate of these DS models are usually obtained from a set of demonstrations of the task. When modeling robot discrete motions with DS, ensuring stability of the learned DS is a key requirement to provide a useful policy. In this paper we propose an imitation learning approach that exploits the power of Control Lyapunov Function (CLF) control scheme to ensure global asymptotic stability of nonlinear DS. Given a set of demonstrations of a task, our approach proceeds in three steps: (1) Learning a valid Lyapunov function from the demonstrations by solving a constrained optimization problem, (2) Using one of the-state-of-the-art regression techniques to model an (unstable) estimate of the motion from the demonstrations, and (3) Using (1) to ensure stability of (2) during the task execution via solving a constrained convex optimization problem. The proposed approach allows learning a larger set of robot motions compared to existing methods that are based on quadratic Lyapunov functions. Additionally, by using the CLF formalism, the problem of ensuring stability of DS motions becomes independent from the choice of regression method. Hence it allows the user to adopt the most appropriate technique based on the requirements of the task at hand without compromising stability. We evaluate our approach both in simulation and on the 7 degrees of freedom Barrett WAM arm.
更多查看译文
关键词
Robot point-to-point movements,Imitation learning,Control Lyapunov function,Nonlinear dynamical systems,Stability analysis,Movement primitives
AI 理解论文
溯源树
样例
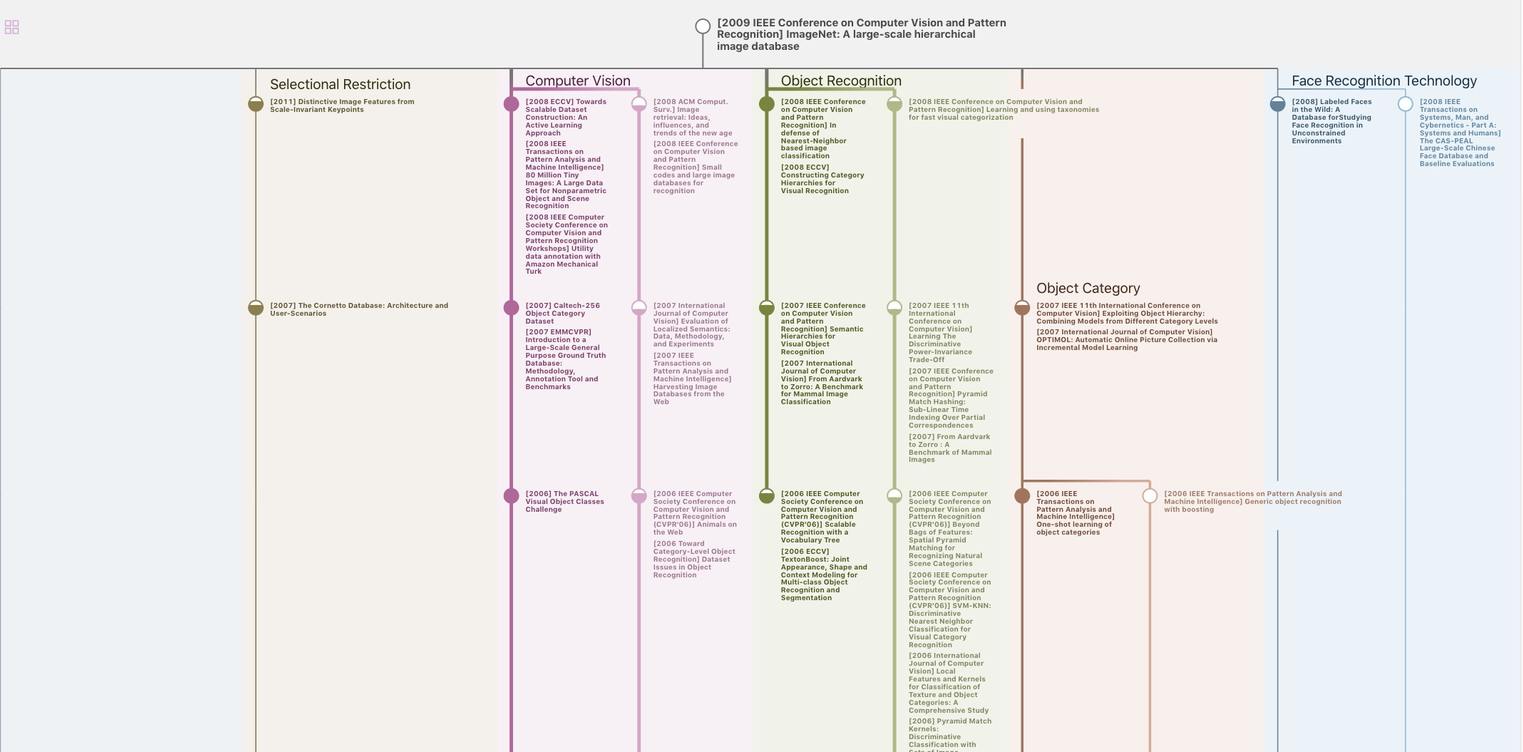
生成溯源树,研究论文发展脉络
Chat Paper
正在生成论文摘要