Hyper-parameter Selection for Sparse LS-SVM via Minimization of its Localized Generalization Error.
IJWMIP(2013)
摘要
Sparse LS-SVM yields better generalization capability and reduces prediction time in comparison to full dense LS-SVM. However, both methods require careful selection of hyper-parameters (HPS) to achieve high generalization capability. Leave-One-Out Cross Validation (LOO-CV) and k-fold Cross Validation (k-CV) are the two most widely used hyper-parameter selection methods for LS-SVMs. However, both fail to select good hyper-parameters for sparse LS-SVM. In this paper we propose a new hyper-parameter selection method, LGEM-HPS, for LS-SVM via minimization of the Localized Generalization Error (L-GEM). The L-GEM consists of two major components: empirical mean square error and sensitivity measure. A new sensitivity measure is derived for LS-SVM to enable the LGEM-HPS select hyper-parameters yielding LS-SVM with smaller training error and minimum sensitivity to minor changes in inputs. Experiments on eleven UCI data sets show the effectiveness of the proposed method for selecting hyper-parameters for sparse LS-SVM. © 2013 World Scientific Publishing Company.
更多查看译文
关键词
SUPPORT VECTOR MACHINES,OUT CROSS-VALIDATION,MODEL SELECTION,CLASSIFIERS
AI 理解论文
溯源树
样例
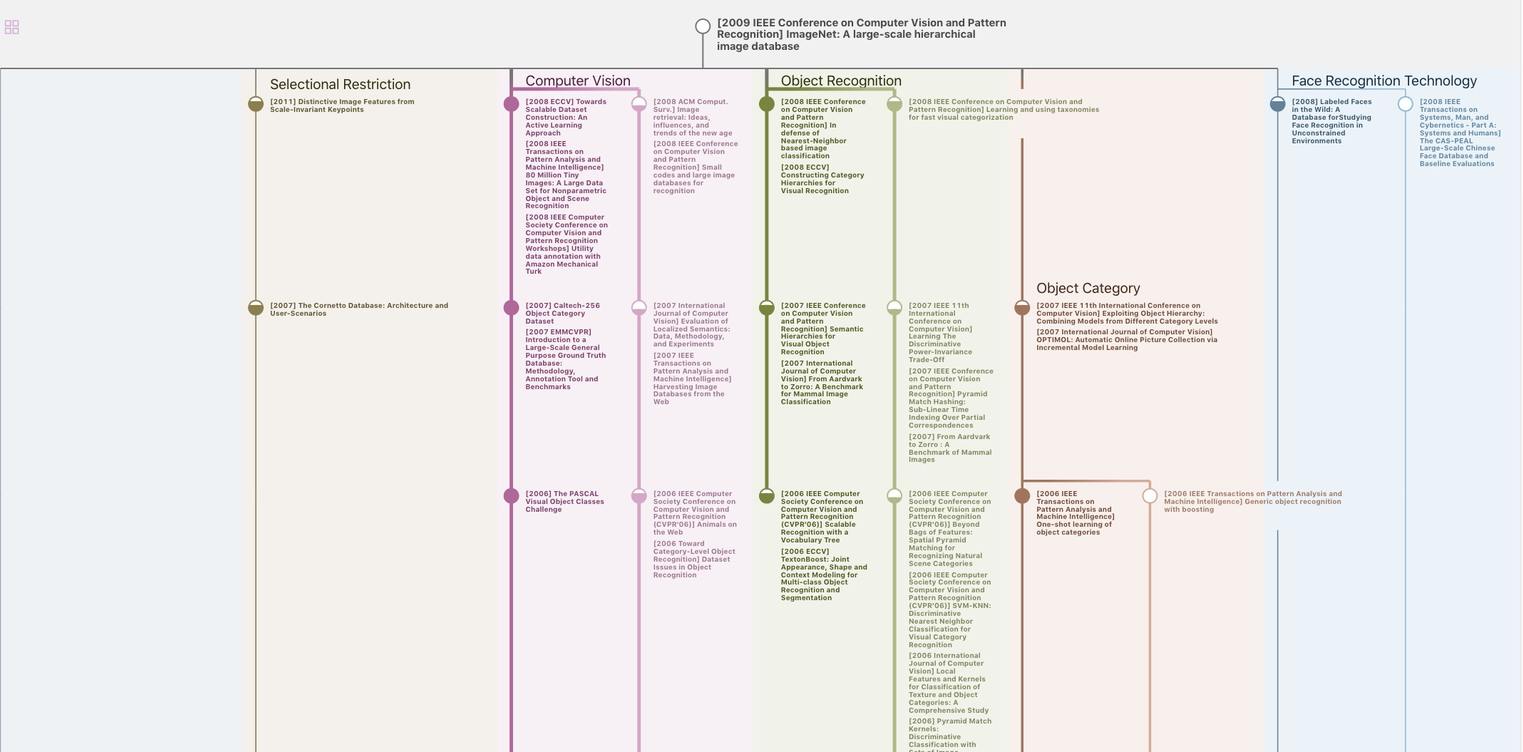
生成溯源树,研究论文发展脉络
Chat Paper
正在生成论文摘要