Mosaic: A Proximity Graph Approach For Agglomerative Clustering
DaWaK'07: Proceedings of the 9th international conference on Data Warehousing and Knowledge Discovery(2007)
摘要
Representative-based clustering algorithms are quite popular due to their relative high speed and because of their sound theoretical foundation. On the other hand, the clusters they can obtain are limited to convex shapes and clustering results are also highly sensitive to initializations. In this paper, a novel agglomerative clustering algorithm called MOSAIC is proposed which greedily merges neighboring clusters maximizing a given fitness function. MOSAIC uses Gabriel graphs to determine which clusters are neighboring and approximates non-convex shapes as the unions of small clusters that have been computed using a representative-based clustering algorithm. The experimental results show that this technique leads to clusters of higher quality compared to running a representative clustering algorithm stand-alone. Given a suitable fitness function, MOSAIC is able to detect arbitrary shape clusters. In addition, MOSAIC is capable of dealing with high dimensional data.
更多查看译文
关键词
post-processing,hybrid clustering,finding clusters of arbitrary shape,agglomerative clustering,using proximity graphs for clustering
AI 理解论文
溯源树
样例
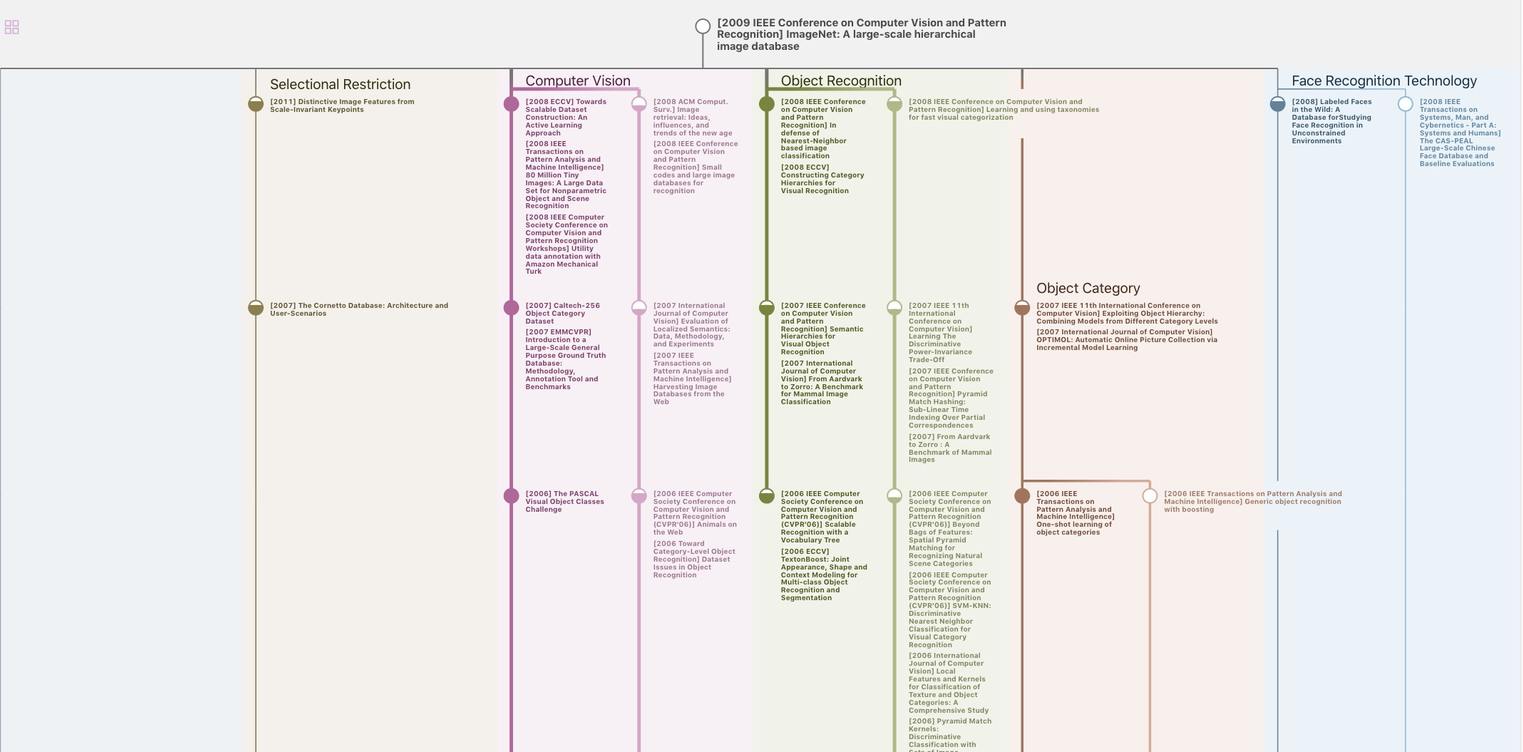
生成溯源树,研究论文发展脉络
Chat Paper
正在生成论文摘要