Feature data optimization with LVQ technique in semantic image annotation
ISDA(2010)
摘要
In order to improve the classifier performance in semantic image annotation, we propose a novel method which adopts learning vector quantization (LVQ) technique to optimize low level feature data extracted from given image. Some representative vectors are selected with LVQ to train support vector machine (SVM) classifier instead of using all feature data. Performance is compared between the methods with and without feature data optimization when SVM is applied to semantic image annotation. Experiment results show that the proposed method has a better performance than that without using LVQ technique.
更多查看译文
关键词
optimisation,learning vector quantilization,classifier performance,feature data optimization,learning (artificial intelligence),automatic image annotation,semantic image annotation,learning vector quantization technique,support vector machine classifier,image classification,support vector machine,image retrieval,low level feature data extraction,support vector machines,lvq technique,semantics,learning vector quantization,feature extraction,testing,learning artificial intelligence,image annotation
AI 理解论文
溯源树
样例
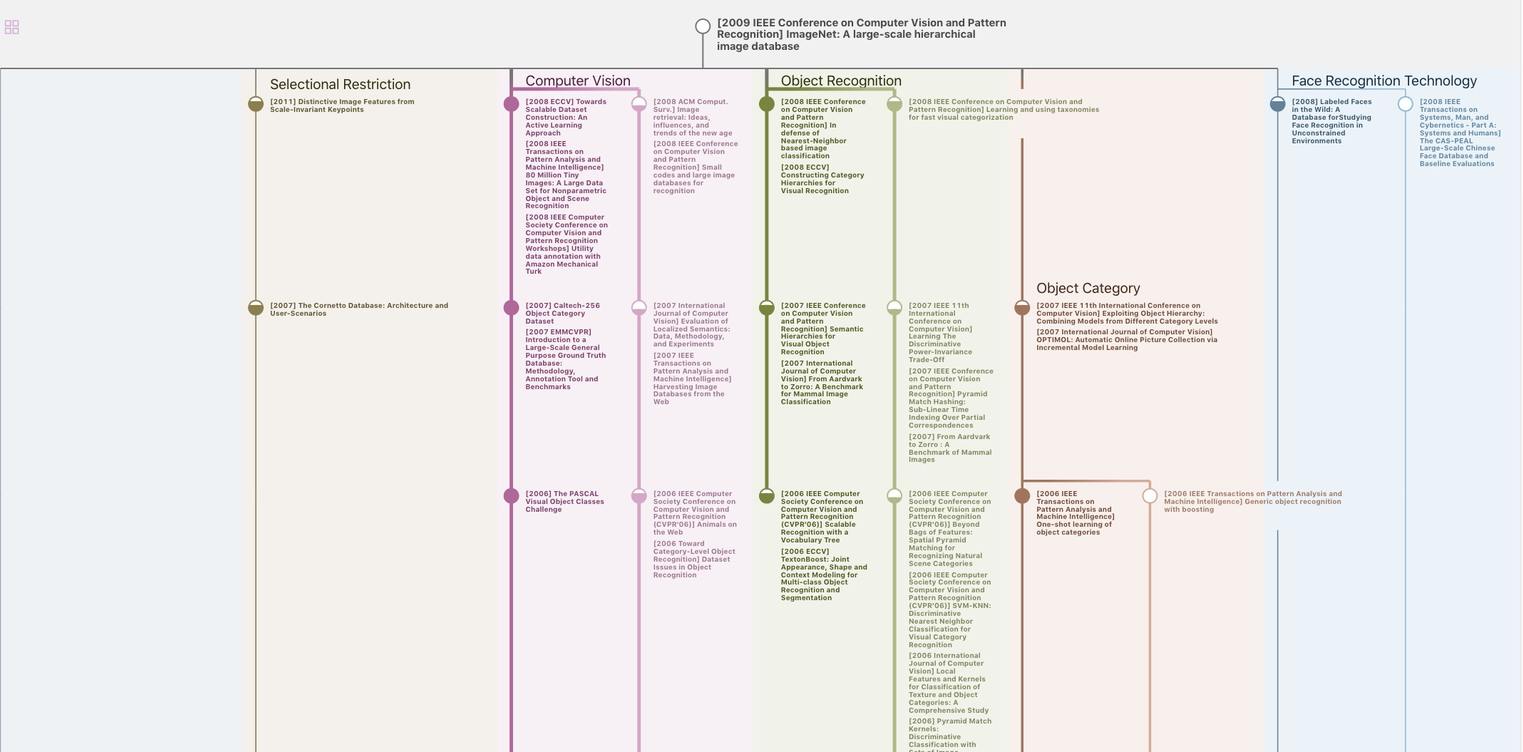
生成溯源树,研究论文发展脉络
Chat Paper
正在生成论文摘要