Exploiting Structural Information Of Data In Active Learning
ARTIFICIAL INTELLIGENCE AND SOFT COMPUTING, ICAISC 2014, PT II(2014)
摘要
In recent years, the active learning algorithms have focused on combining correlation criterion and uncertainty criterion for evaluating instances. Although these criteria might be useful, applying these measures on whole input space globally may lead to inefficient selected instances for active learning. The proposed method takes advantage of clustering to partition input space to subspaces. Then it exploits both labeled and unlabeled data locally for selection of instances by using a graph-based active learning. We define a novel utility score for selecting clusters by combining uncertainty criterion, local entropy of clusters and the factor of contribution of each cluster in queries. Experimental results reveal an elevated performance as compared to several state of the art and widely used active learning strategies.
更多查看译文
关键词
Active Learning, Clustering, Uncertainty Criterion, Correlation Criterion
AI 理解论文
溯源树
样例
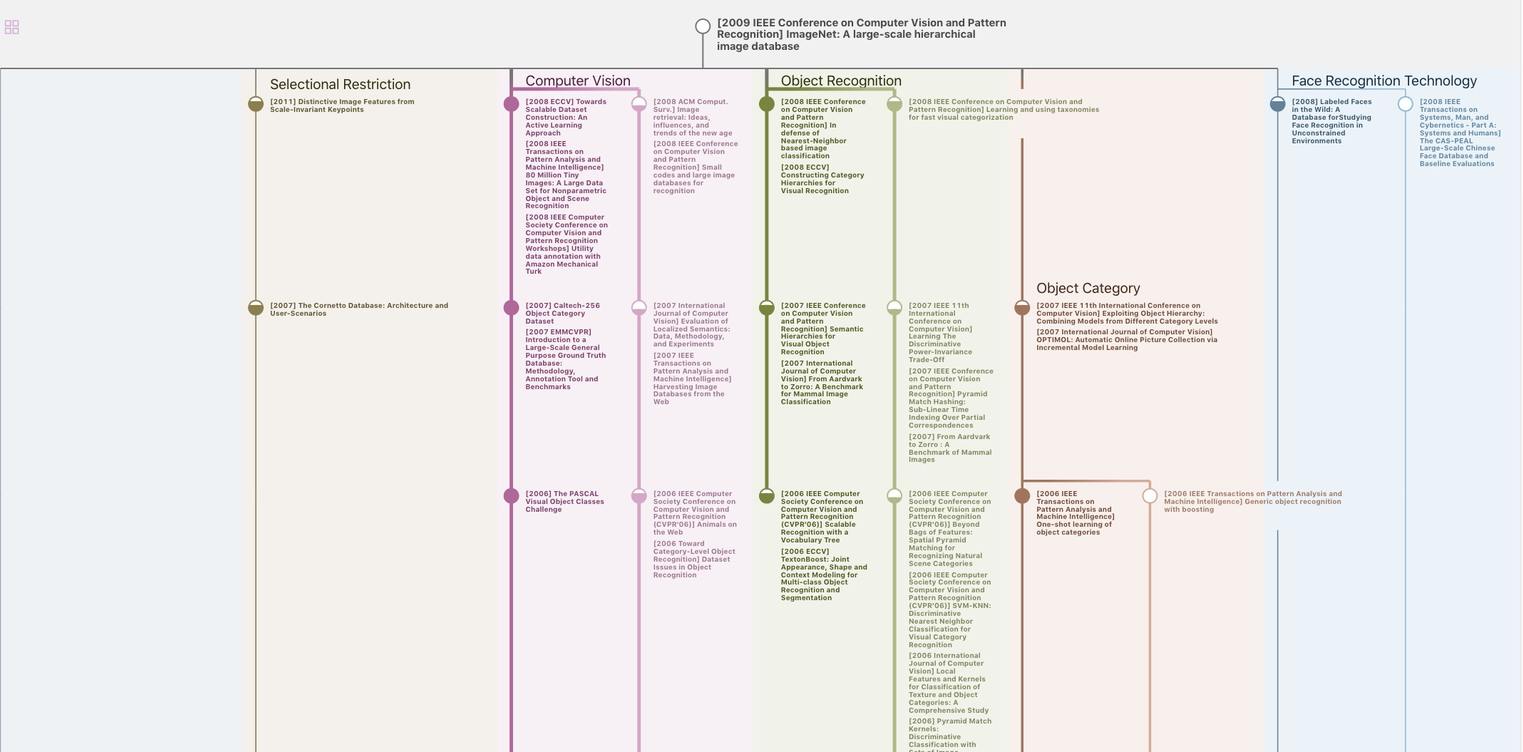
生成溯源树,研究论文发展脉络
Chat Paper
正在生成论文摘要