Unsupervised training of subspace gaussian mixture models for conversational telephone speech recognition
ICASSP(2012)
摘要
This paper presents our preliminary works on exploring unsupervised training of subspace gaussian mixture models for under-resourced CTS recognition task. The subspace model yields better performance than conventional GMM model, particularly in small or middle-sized training set. As an effective way to save human efforts, unsupervised learning is often applied to automatically transcribe a large amount of speech archives. The additional auto-transcribed data may help to improve model accuracy. In this paper, experiments are carried out on two publicly available English conversational telephone speech corpora. Both GMM and SGMM model in combination with unsupervised learning are examined and compared in this paper.
更多查看译文
关键词
autotranscribed data,subspace acoustic model,speech recognition with low resources,speech archives,speech recognition,conversational telephone speech recognition,learning (artificial intelligence),subspace gaussian mixture models,under-resourced cts recognition task,middle-sized training set,english conversational telephone speech corpora,gaussian processes,unsupervised learning,sgmm model,unsupervised training,acoustics,speech,learning artificial intelligence,data models,hidden markov models
AI 理解论文
溯源树
样例
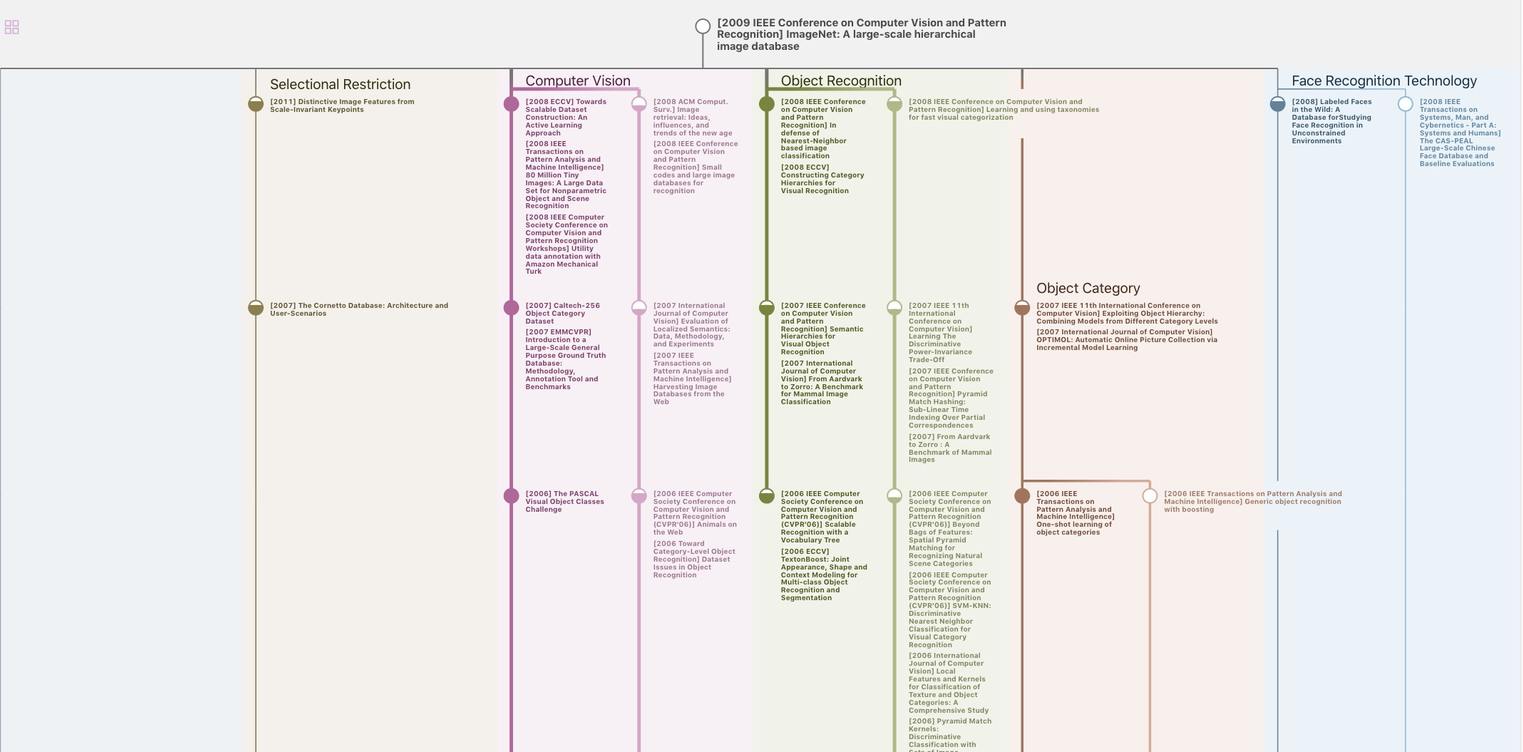
生成溯源树,研究论文发展脉络
Chat Paper
正在生成论文摘要