Theoretical and algorithmic developments in markov chain monte carlo
Theoretical and algorithmic developments in markov chain monte carlo(2008)
摘要
This PhD thesis is concerned with three problems: (1) “Bayesian Change Point Analysis for Mechanistic Modeling,” (3) “Assessing Convergence and Mixing of Markov Chain Monte Carlo via Stratification,” and (2) “Bayesian Analysis via Diffusion Monte Carlo.”In Bayesian parametric change point modeling, the primary challenges are detection of the number and locations of change points and obtaining the posterior distribution of other unknown parameters involved in the model. Chapter 2 suggests approaches for dealing with the computational burden arising in change point models that involving mechanistic reasoning as well as random explanatory variables. We present an example involving flow velocities of ice sheets in the Lambert Glacial Basin of east Antarctica. We pay special attention to the development of reasonable priors that can reflect a variety of prior information.In Chapter 3 we apply the notion of post-stratification to develop assessment tools for MCMC analysis. These tools are based on comparison of variances of two natural estimators. Based on the estimates of these variances we propose a test statistic which helps in checking convergence and mixing of MCMC. Our method is illustrated using a Bayesian change-point model for ice flow velocity in East Antarctica, a logistic regression model, a latent variable model for Arsenic concentration in public water systems in Arizona, and a regime-switching model for Pacific sea surface temperatures.Markov chain algorithms using langevin-type diffusion sometimes offer potentially useful methods in cases for which other MCMC methods are challenging. The key idea is to develop a stochastic process whose stationary distribution is the target posterior using diffusions represented as solutions to stochastic differential equations (SDE). The main challenge is to solve the required SDE’s. Naive discretizations like the Euler method can lead to lack of ergodicity. Hence, more sophisticated discretizations or Metropolis-Hastings correction steps are prescribed. Chapter 4 of my dissertation research deals with development of diffusion based algorithms for posterior distributions arising from non-conjugate Gaussian models with non-linear mean and variance functions.
更多查看译文
关键词
change point model,markov chain monte carlo,regime-switching model,latent variable model,non-conjugate Gaussian model,east Antarctica,Bayesian Change Point Analysis,Bayesian Analysis,algorithmic development,posterior distribution,logistic regression model,Bayesian change-point model
AI 理解论文
溯源树
样例
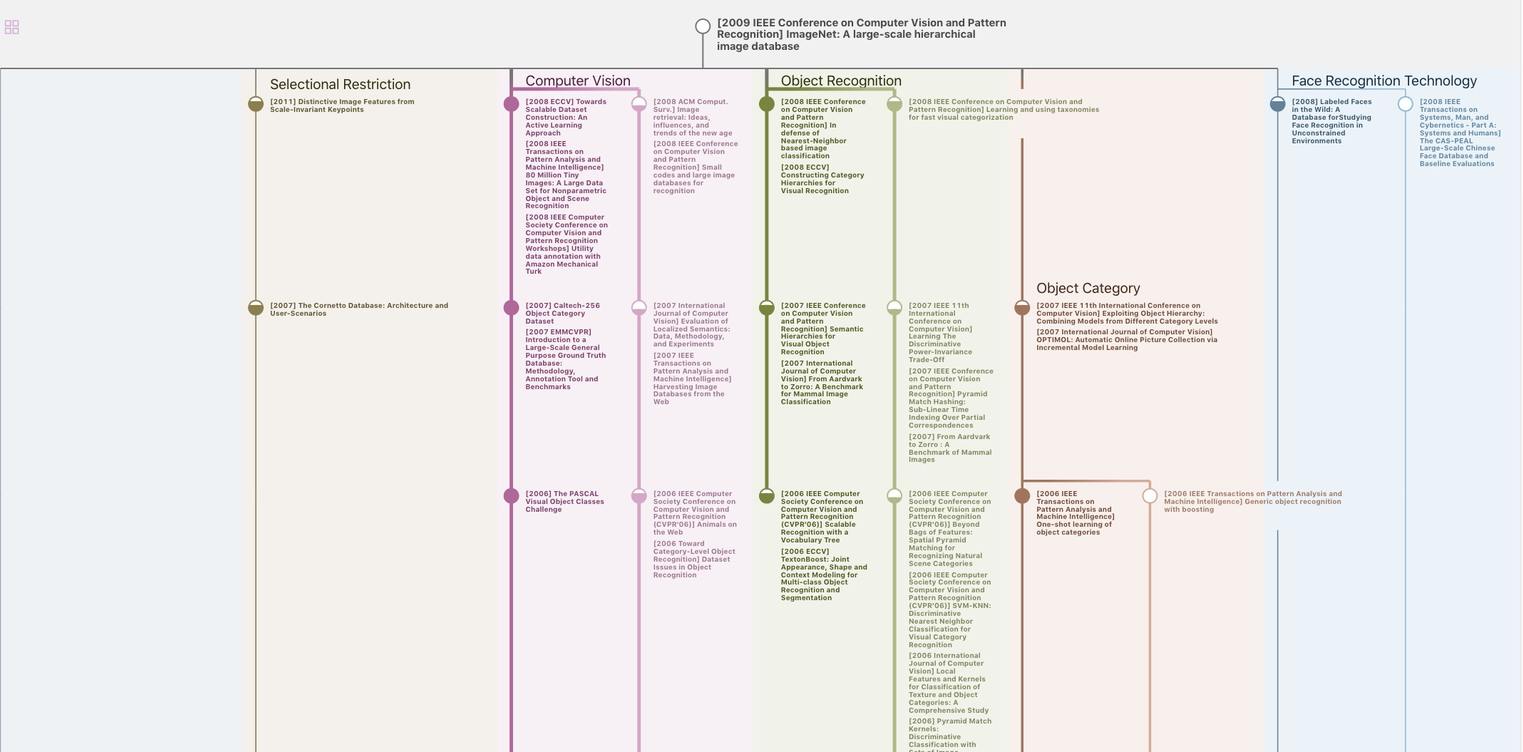
生成溯源树,研究论文发展脉络
Chat Paper
正在生成论文摘要