Speech Activity Detection On Youtube Using Deep Neural Networks
14TH ANNUAL CONFERENCE OF THE INTERNATIONAL SPEECH COMMUNICATION ASSOCIATION (INTERSPEECH 2013), VOLS 1-5(2013)
摘要
Speech activity detection (SAD) is an important first step in speech processing. Commonly used methods (e.g., frame-level classification using gaussian mixture models (GMMs)) work well under stationary noise conditions, but do not generalize well to domains such as YouTube, where videos may exhibit a diverse range of environmental conditions. One solution is to augment the conventional cepstral features with additional, hand-engineered features (e.g., spectral flux, spectral centroid, multiband spectral entropies) which are robust to changes in environment and recording condition. An alternative approach, explored here, is to learn robust features during the course of training using an appropriate architecture such as deep neural networks (DNNs). In this paper we demonstrate that a DNN with input consisting of multiple frames of mel frequency cepstral coefficients (MFCCs) yields drastically lower frame-wise error rates (19.6%) on YouTube videos compared to a conventional GMM based system (40%).
更多查看译文
关键词
speech activity detection,voice activity detection,segmentation,deep neural networks
AI 理解论文
溯源树
样例
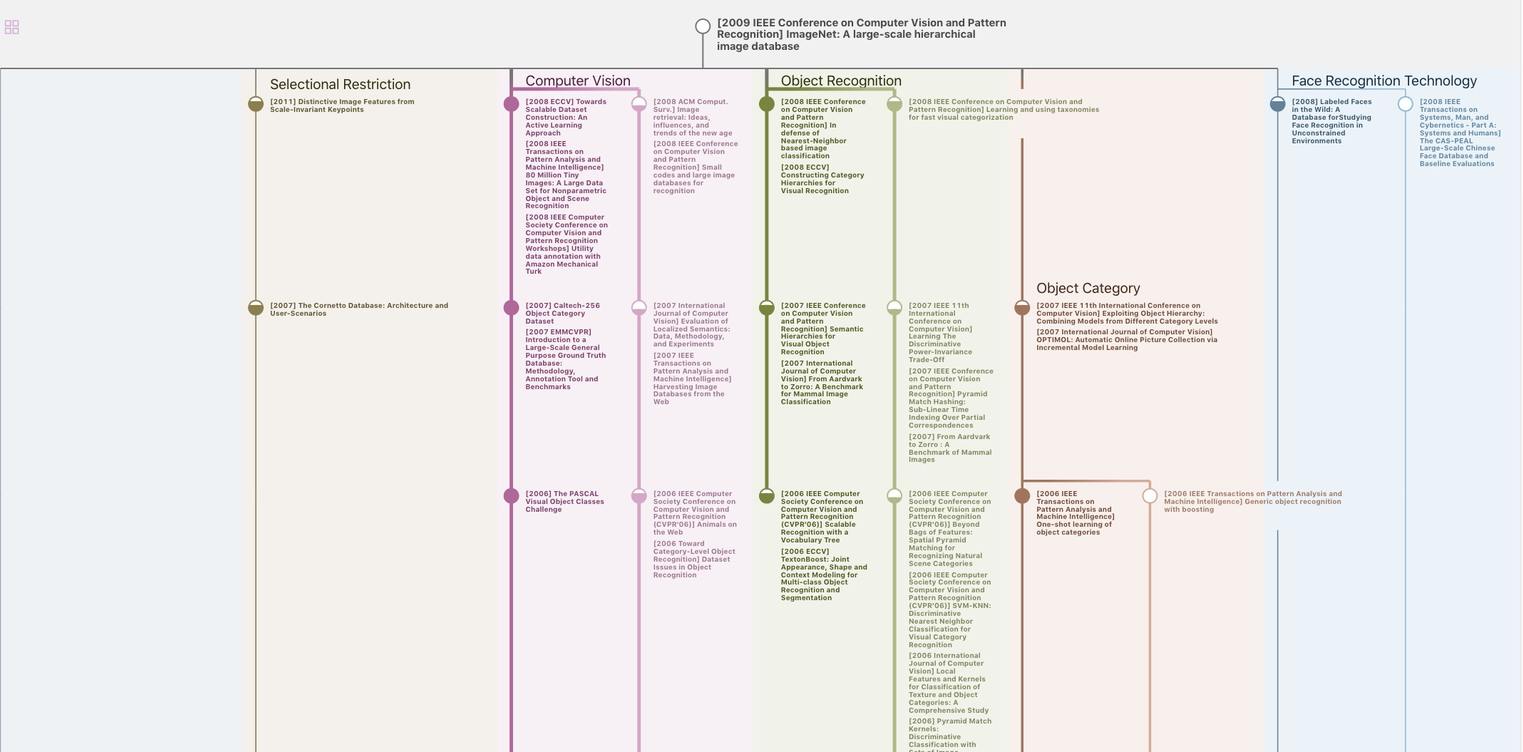
生成溯源树,研究论文发展脉络
Chat Paper
正在生成论文摘要