Mining Spatial Trajectories Using Non-parametric Density Functions.
MLDM'11: Proceedings of the 7th international conference on Machine learning and data mining in pattern recognition(2011)
摘要
Analyzing trajectories is important and has many applications, such as surveillance, analyzing traffic patterns and hurricane path prediction. In this paper, we propose a unique, non-parametric trajectory density estimation approach to obtain trajectory density functions that are used for two purposes. First, a density-based clustering algorithm DENTRAC that operates on such density functions is introduced. Second, unique post-analysis techniques that use the trajectory density function are proposed. Our method is capable of ranking trajectory clusters based on different characteristics of density clusters, and thus has the ability to summarize clusters from different perspectives, such as the compactness of member trajectories or the probability of their occurrence. We evaluate the proposed methods on synthetic traffic and real-world Atlantic hurricane datasets. The results show that our simple, yet effective approach extracts valuable knowledge from trajectories that is difficult to obtain with other approaches.
更多查看译文
关键词
Spatial Data Mining, Non-Parametric Density Function, Mining Spatial Trajectory Datasets, Density-based Trajectory Clustering
AI 理解论文
溯源树
样例
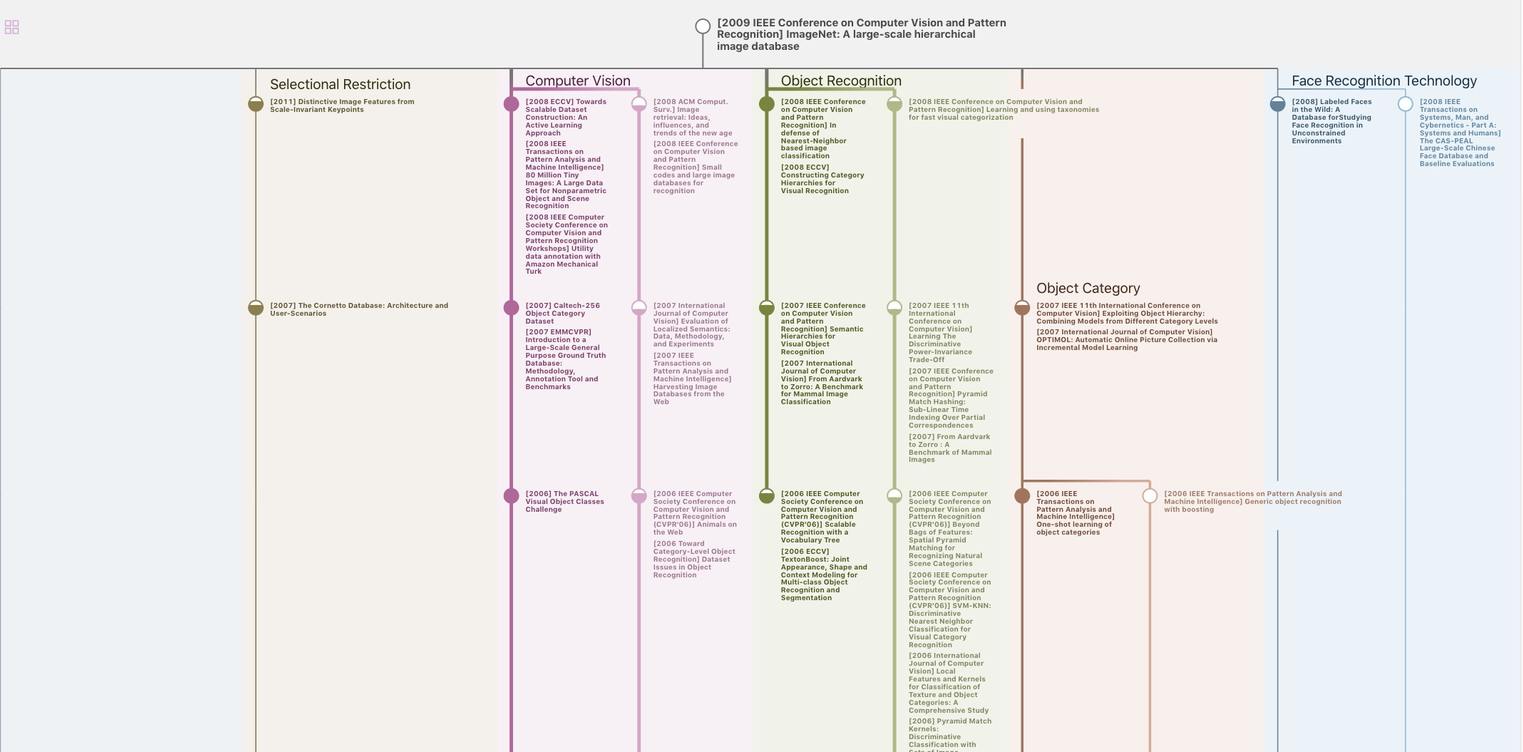
生成溯源树,研究论文发展脉络
Chat Paper
正在生成论文摘要