Skill as a function of time scale in ensembles of seasonal hindcasts
Climate Dynamics(2001)
摘要
Forecast skill as a function of time lead and time averaging is examined in two 6-member ensembles of seasonal hindcasts. One ensemble is produced with the second generation general circulation model of the Canadian Centre for Climate Modelling and Analysis (GCM2) and the other with a reduced resolution version of the numerical weather prediction model of the Canadian Meteorological Centre (SEF). The integrations are initiated from the NCEP/NCAR reanalyzed data. Monthly sea surface temperature anomalies observed prior to the forecast period are maintained throughout the forecast season. A statistical forecast improvement technique, based on the singular value decomposition of forecast and reanalyzed fields, is discussed and evaluated. A simple analogue of the hindcast integrations is used to examine the behavior of two common skill scores, the correlation skill score and the explained variance skill score. The maximal skill score and the corresponding optimal forecast in this analogue are identified. The total skill of the optimal forecast is a sum of two terms, one associated with the initial conditions and the other with the lower boundary forcing. The two sources of skill operate on different time scales, with initial conditions being more important in the first one-two weeks and the atmospheric response to the boundary forcing becoming more dominant for longer time leads and time averages. This suggests that these sources of skill should be considered separately in forecast optimization. The statistical technique is moderately successful in improving the skill of monthly to seasonal forecasts of 500 hPa height ( Z 500 ) and 700 hPa temperature ( T 700 ) in the Northern Hemisphere and in the North Pacific/North America sector. The improvement is better when the forecasts for the first week and for the rest of the season are optimized separately. The SEF model produces better Z 500 and T 700 forecasts than GCM2 in the first one-two weeks whereas GCM2 performs slightly better at longer time leads. The skill of zero time lead forecast decays rapidly with averaging interval for time averages up to about 30–45 days and stabilizes, or even rises, for longer time averages. Excluding the first week from seasonal forecasts results in substantial degradation of predictive skill.
更多查看译文
关键词
Time Lead,Skill Score,Seasonal Forecast,Numerical Weather Prediction Model,Time Lead Forecast
AI 理解论文
溯源树
样例
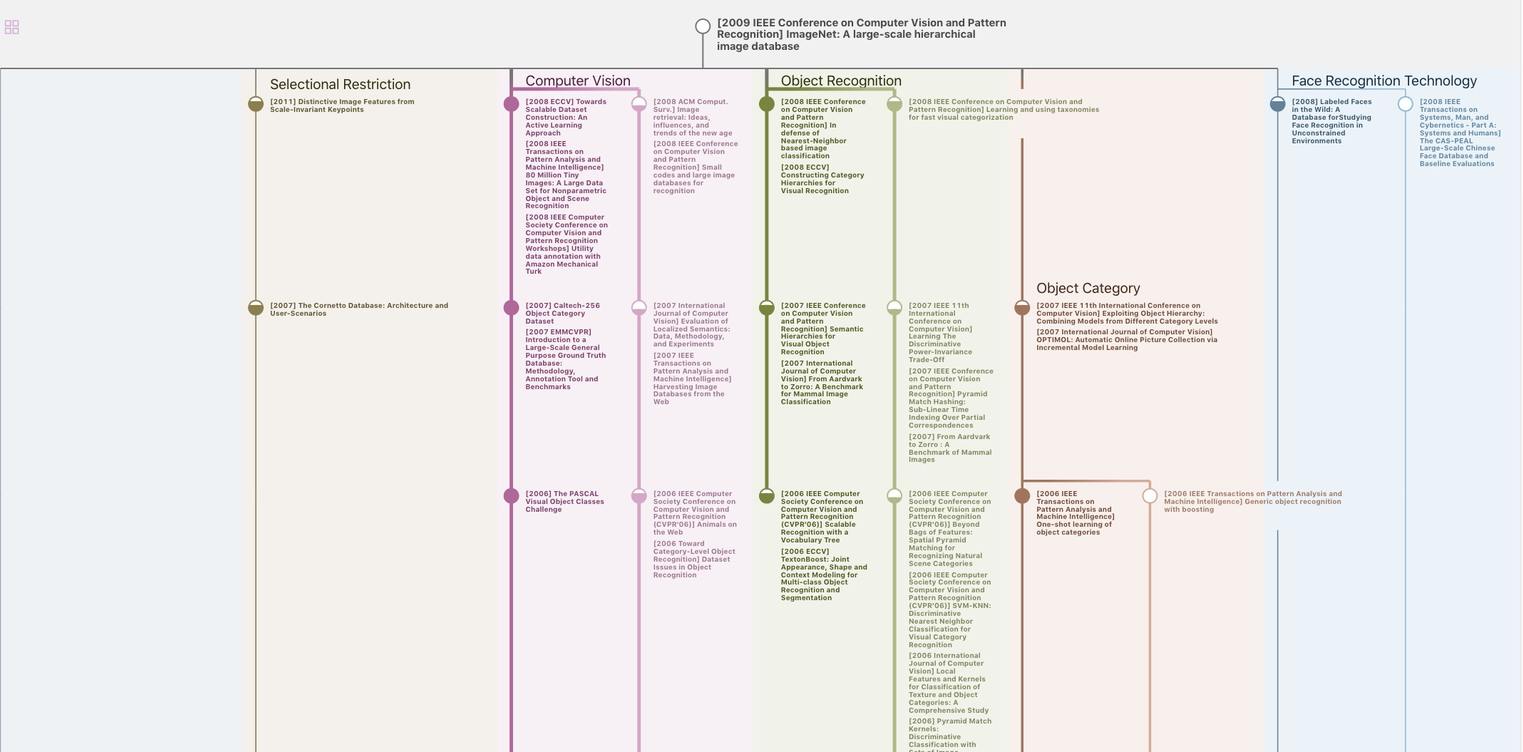
生成溯源树,研究论文发展脉络
Chat Paper
正在生成论文摘要