Navigation with memory in a partially observable environment
Robotics and Autonomous Systems(2006)
摘要
The paper presents an architecture that allows the reactive visual navigation via an unsupervised reinforcement learning. This objective is reached using Q-learning and a hierarchical approach to the developed architecture. Using these techniques requires a deviation from the Partially Observable Markov Decision Processes (POMDP) and some innovations: heuristic techniques for generalizing the experience and for treating the partial observability; a technique for the speed adjournment of the Q function; the definition of a special reinforcement policy adequate for learning a complex task without supervision. The result is a satisfactory learning of the navigation assignment in a simulated environment.
更多查看译文
关键词
Hierarchical representation of POMDPs,Task hierarchy,Hierarchical reinforcement learning,Q-learning,Self-localization,Navigation
AI 理解论文
溯源树
样例
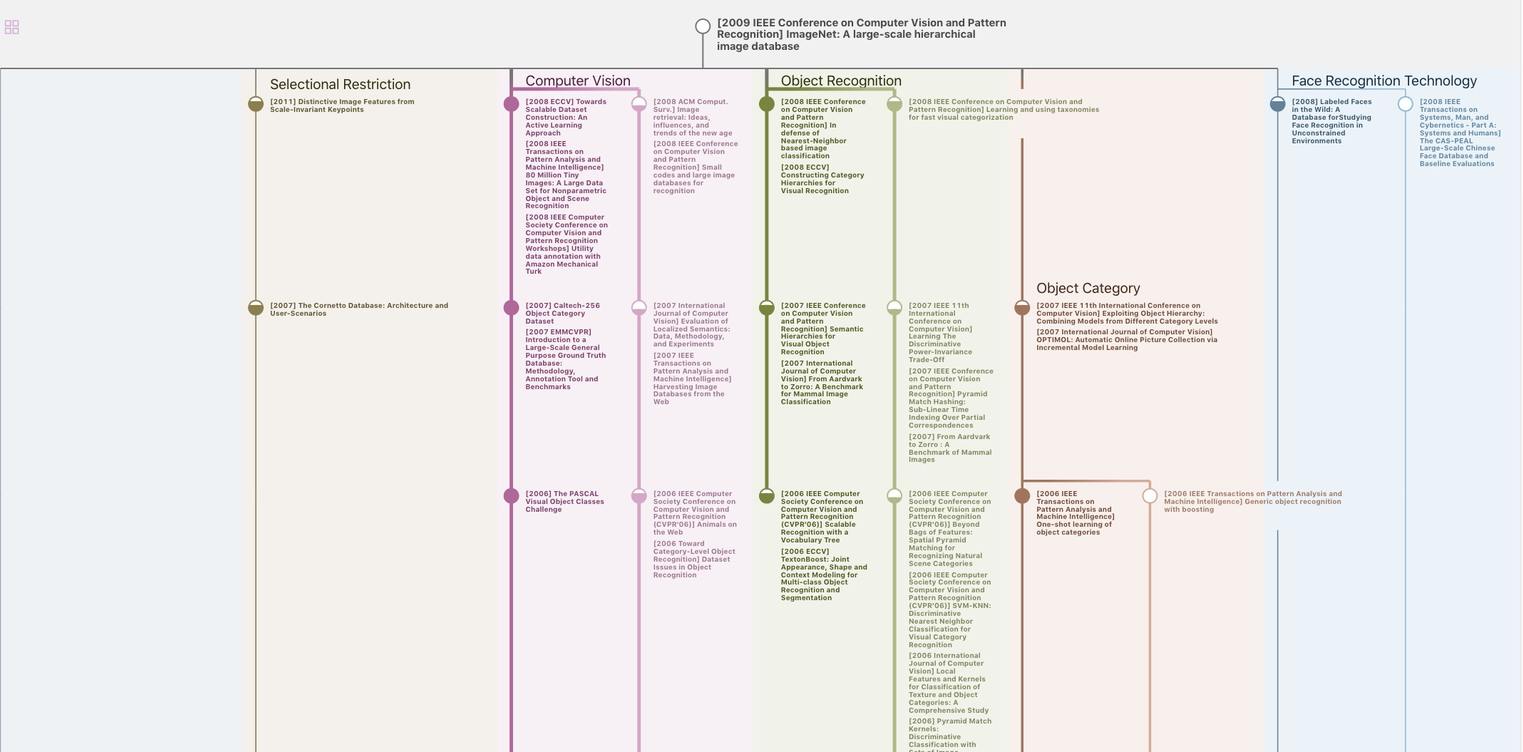
生成溯源树,研究论文发展脉络
Chat Paper
正在生成论文摘要