Auto-WEKA: Combined Selection and Hyperparameter Optimization of Classification Algorithms
KDD(2013)
摘要
Many different machine learning algorithms exist; taking into account each algorithm's hyperparameters, there is a staggeringly large number of possible alternatives overall. We consider the problem of simultaneously selecting a learning algorithm and setting its hyperparameters, going beyond previous work that addresses these issues in isolation. We show that this problem can be addressed by a fully automated approach, leveraging recent innovations in Bayesian optimization. Specifically, we consider a wide range of feature selection techniques (combining 3 search and 8 evaluator methods) and all classification approaches implemented in WEKA, spanning 2 ensemble methods, 10 meta-methods, 27 base classifiers, and hyperparameter settings for each classifier. On each of 21 popular datasets from the UCI repository, the KDD Cup 09, variants of the MNIST dataset and CIFAR-10, we show classification performance often much better than using standard selection/hyperparameter optimization methods. We hope that our approach will help non-expert users to more effectively identify machine learning algorithms and hyperparameter settings appropriate to their applications, and hence to achieve improved performance.
更多查看译文
关键词
Model selection,Hyperparameter optimization,WEKA
AI 理解论文
溯源树
样例
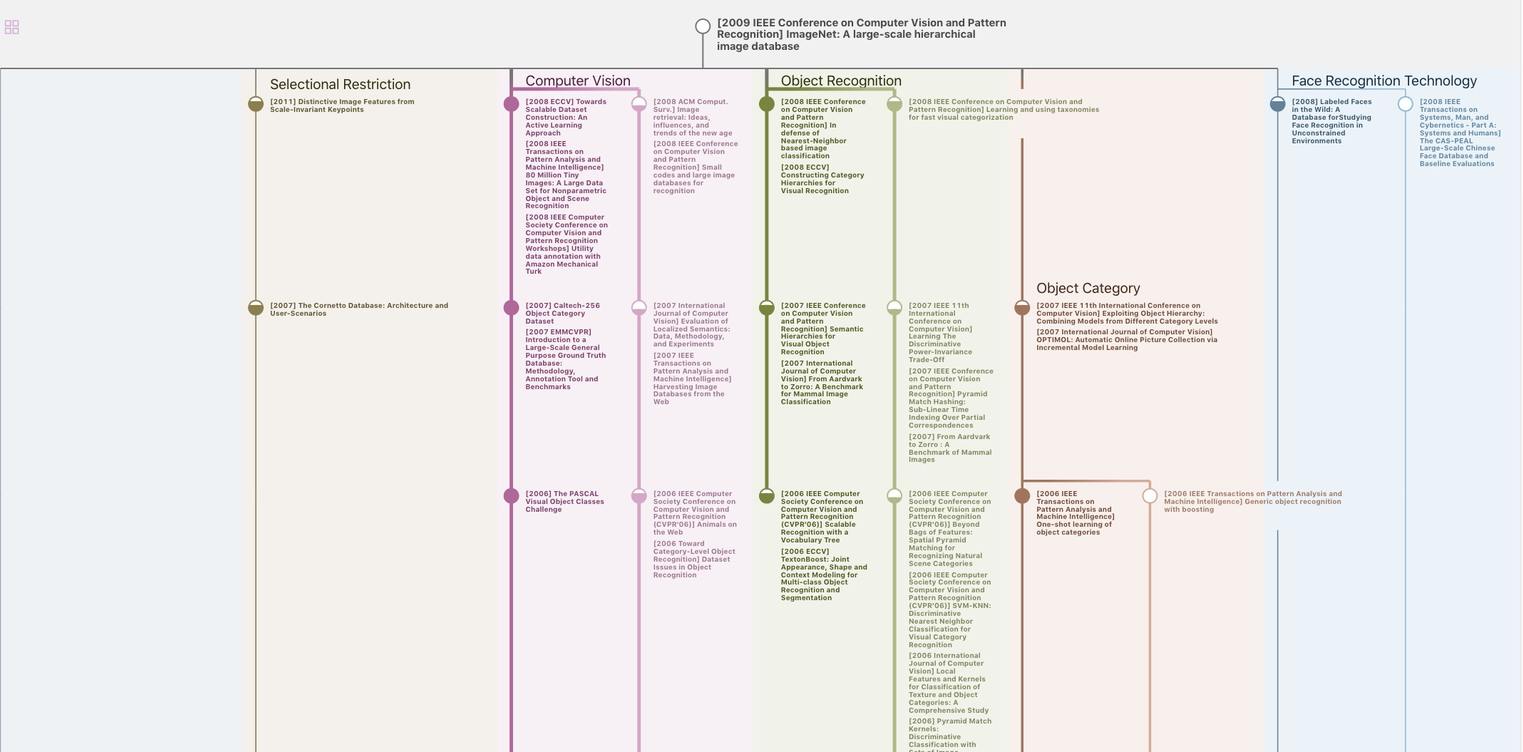
生成溯源树,研究论文发展脉络
Chat Paper
正在生成论文摘要